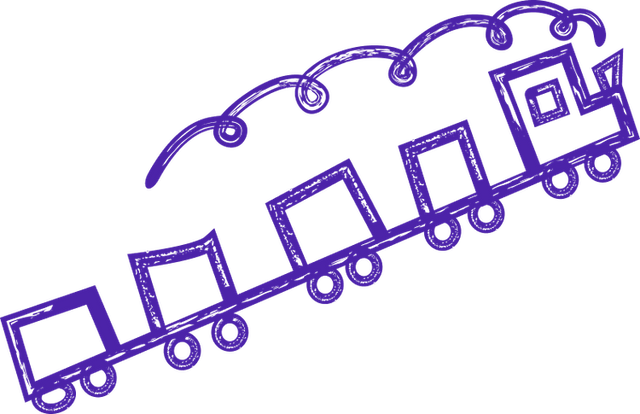
what is unsupervised learning in ai
Unsupervised Learning in AI
Unsupervised Learning in AI refers to a type of machine learning where an algorithm learns patterns, relationships, and structures within a dataset without any explicit guidance or labeled examples. Unlike supervised learning, which relies on labeled data to make predictions, unsupervised learning aims to discover hidden patterns and gain insights from unlabeled data.
In unsupervised learning, the algorithm explores the data on its own, identifying similarities, differences, and underlying structures. It does not receive any feedback or predefined categories to guide its learning process. Instead, the algorithm autonomously clusters or groups similar data points together, allowing patterns to emerge naturally.
One of the key techniques used in unsupervised learning is clustering, where the algorithm groups similar data points based on their characteristics or attributes. This helps in identifying patterns and understanding the inherent structure of the data. Another technique is dimensionality reduction, which aims to reduce the number of features or variables in a dataset while preserving important information. This can be useful in visualizing complex data or reducing computational complexity.
Unsupervised learning has numerous applications across various domains. For instance, in customer segmentation, unsupervised learning algorithms can analyze customer behavior and group them into distinct segments based on their preferences, demographics, or purchasing patterns. This enables businesses to tailor their marketing strategies and offerings to specific customer segments, leading to improved customer satisfaction and increased sales.
Another application is anomaly detection, where unsupervised learning algorithms can identify unusual or abnormal data points that deviate from the expected patterns. This is particularly valuable in fraud detection, network security, or predictive maintenance, where identifying anomalies can help in preventing potential threats or failures.
Additionally, unsupervised learning plays a crucial role in recommendation systems. By analyzing user behavior and preferences, unsupervised algorithms can suggest personalized recommendations, such as movies, products, or articles, based on similarities with other users or items. This enhances user experience and engagement, leading to higher user satisfaction and increased revenue for businesses.
Despite its wide range of applications, unsupervised learning also presents challenges. Since there is no ground truth or labeled data, evaluating the performance of unsupervised algorithms can be subjective. It often requires domain expertise and human interpretation to validate the discovered patterns or clusters. Furthermore, the scalability of unsupervised learning algorithms can be a concern when dealing with large and complex datasets.
In conclusion, unsupervised learning is a powerful technique in AI that enables machines to autonomously discover patterns and structures in unlabeled data. Through clustering, dimensionality reduction, and other techniques, unsupervised learning algorithms provide valuable insights, enabling businesses and researchers to gain a deeper understanding of their data and make informed decisions.
In unsupervised learning, the algorithm explores the data on its own, identifying similarities, differences, and underlying structures. It does not receive any feedback or predefined categories to guide its learning process. Instead, the algorithm autonomously clusters or groups similar data points together, allowing patterns to emerge naturally.
One of the key techniques used in unsupervised learning is clustering, where the algorithm groups similar data points based on their characteristics or attributes. This helps in identifying patterns and understanding the inherent structure of the data. Another technique is dimensionality reduction, which aims to reduce the number of features or variables in a dataset while preserving important information. This can be useful in visualizing complex data or reducing computational complexity.
Unsupervised learning has numerous applications across various domains. For instance, in customer segmentation, unsupervised learning algorithms can analyze customer behavior and group them into distinct segments based on their preferences, demographics, or purchasing patterns. This enables businesses to tailor their marketing strategies and offerings to specific customer segments, leading to improved customer satisfaction and increased sales.
Another application is anomaly detection, where unsupervised learning algorithms can identify unusual or abnormal data points that deviate from the expected patterns. This is particularly valuable in fraud detection, network security, or predictive maintenance, where identifying anomalies can help in preventing potential threats or failures.
Additionally, unsupervised learning plays a crucial role in recommendation systems. By analyzing user behavior and preferences, unsupervised algorithms can suggest personalized recommendations, such as movies, products, or articles, based on similarities with other users or items. This enhances user experience and engagement, leading to higher user satisfaction and increased revenue for businesses.
Despite its wide range of applications, unsupervised learning also presents challenges. Since there is no ground truth or labeled data, evaluating the performance of unsupervised algorithms can be subjective. It often requires domain expertise and human interpretation to validate the discovered patterns or clusters. Furthermore, the scalability of unsupervised learning algorithms can be a concern when dealing with large and complex datasets.
In conclusion, unsupervised learning is a powerful technique in AI that enables machines to autonomously discover patterns and structures in unlabeled data. Through clustering, dimensionality reduction, and other techniques, unsupervised learning algorithms provide valuable insights, enabling businesses and researchers to gain a deeper understanding of their data and make informed decisions.