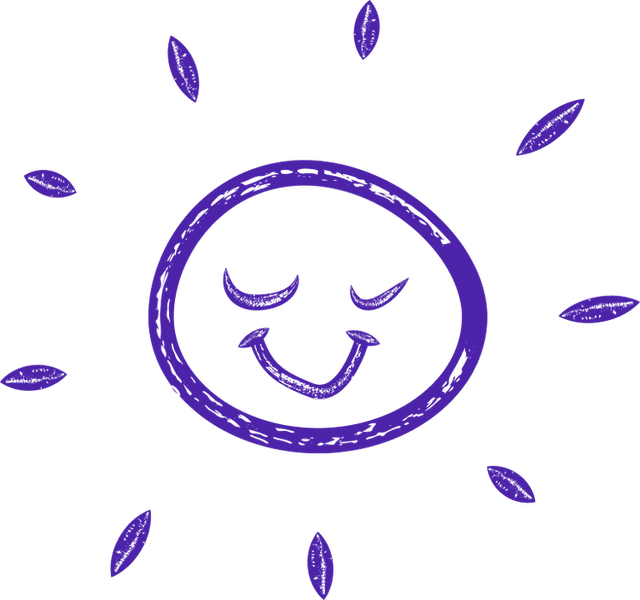
what is time series analysis in data science
Time Series Analysis in Data Science
Time Series Analysis in Data Science refers to the statistical technique used to analyze and interpret data that is collected over a period of time. It involves studying the patterns, trends, and relationships within the data to make predictions and forecasts for future events or outcomes.
In today's data-driven world, businesses and organizations collect vast amounts of time-stamped data from various sources such as sensors, social media, financial markets, weather stations, and more. Time Series Analysis provides a powerful toolset to extract valuable insights from this data and leverage it for decision-making, planning, and optimization purposes.
The fundamental concept behind Time Series Analysis is that the data points are ordered chronologically, with each observation being dependent on the previous ones. This temporal dependency makes time series data distinct from other types of data, and it requires specialized techniques to analyze and interpret it effectively.
One of the primary goals of Time Series Analysis is to understand and describe the underlying patterns and trends within the data. This involves identifying components such as trend, seasonality, cyclicality, and irregular fluctuations. Trend refers to the long-term direction of the data, while seasonality represents recurring patterns that occur within shorter time intervals. Cyclicality refers to patterns that occur over longer time intervals, and irregular fluctuations are random or unpredictable variations in the data.
To analyze time series data, various statistical and mathematical models are employed. These models can be classified into two main categories: descriptive and predictive models. Descriptive models aim to summarize and explain the observed patterns in the data, while predictive models focus on forecasting future values based on historical data.
Some commonly used techniques for Time Series Analysis include:
1. Moving Averages: This method calculates the average of a specific number of consecutive data points to smooth out short-term fluctuations and highlight long-term trends.
2. Autoregressive Integrated Moving Average (ARIMA): ARIMA models are widely used for forecasting time series data. They combine autoregressive (AR), differencing (I), and moving average (MA) components to capture the patterns and dependencies within the data.
3. Exponential Smoothing: This technique assigns exponentially decreasing weights to older observations, giving more importance to recent data points. It is particularly useful for data with no clear trend or seasonality.
4. Fourier Analysis: Fourier analysis decomposes a time series into its constituent frequencies, allowing the identification of periodic patterns and their associated magnitudes.
5. Seasonal Decomposition of Time Series (STL): This method decomposes a time series into its trend, seasonal, and residual components, enabling a detailed understanding of each component's contribution to the overall behavior.
Time Series Analysis finds applications in various domains, including finance, economics, sales forecasting, weather prediction, demand forecasting, and anomaly detection. By analyzing past trends and patterns, organizations can make informed decisions, optimize processes, and develop strategies to achieve their goals.
In conclusion, Time Series Analysis is a crucial tool in the field of Data Science that enables the extraction of valuable insights from time-stamped data. By understanding the underlying patterns and trends within the data, businesses and organizations can make accurate predictions and forecasts, leading to better decision-making and improved performance.
In today's data-driven world, businesses and organizations collect vast amounts of time-stamped data from various sources such as sensors, social media, financial markets, weather stations, and more. Time Series Analysis provides a powerful toolset to extract valuable insights from this data and leverage it for decision-making, planning, and optimization purposes.
The fundamental concept behind Time Series Analysis is that the data points are ordered chronologically, with each observation being dependent on the previous ones. This temporal dependency makes time series data distinct from other types of data, and it requires specialized techniques to analyze and interpret it effectively.
One of the primary goals of Time Series Analysis is to understand and describe the underlying patterns and trends within the data. This involves identifying components such as trend, seasonality, cyclicality, and irregular fluctuations. Trend refers to the long-term direction of the data, while seasonality represents recurring patterns that occur within shorter time intervals. Cyclicality refers to patterns that occur over longer time intervals, and irregular fluctuations are random or unpredictable variations in the data.
To analyze time series data, various statistical and mathematical models are employed. These models can be classified into two main categories: descriptive and predictive models. Descriptive models aim to summarize and explain the observed patterns in the data, while predictive models focus on forecasting future values based on historical data.
Some commonly used techniques for Time Series Analysis include:
1. Moving Averages: This method calculates the average of a specific number of consecutive data points to smooth out short-term fluctuations and highlight long-term trends.
2. Autoregressive Integrated Moving Average (ARIMA): ARIMA models are widely used for forecasting time series data. They combine autoregressive (AR), differencing (I), and moving average (MA) components to capture the patterns and dependencies within the data.
3. Exponential Smoothing: This technique assigns exponentially decreasing weights to older observations, giving more importance to recent data points. It is particularly useful for data with no clear trend or seasonality.
4. Fourier Analysis: Fourier analysis decomposes a time series into its constituent frequencies, allowing the identification of periodic patterns and their associated magnitudes.
5. Seasonal Decomposition of Time Series (STL): This method decomposes a time series into its trend, seasonal, and residual components, enabling a detailed understanding of each component's contribution to the overall behavior.
Time Series Analysis finds applications in various domains, including finance, economics, sales forecasting, weather prediction, demand forecasting, and anomaly detection. By analyzing past trends and patterns, organizations can make informed decisions, optimize processes, and develop strategies to achieve their goals.
In conclusion, Time Series Analysis is a crucial tool in the field of Data Science that enables the extraction of valuable insights from time-stamped data. By understanding the underlying patterns and trends within the data, businesses and organizations can make accurate predictions and forecasts, leading to better decision-making and improved performance.