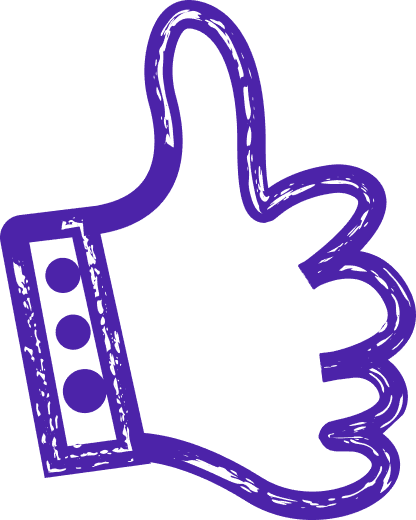
what is stream processing in big data
Stream Processing in Big Data
Stream processing in big data refers to the real-time processing and analysis of continuous data streams. It is a crucial component of big data analytics, enabling organizations to extract valuable insights and make informed decisions in real-time.
In today's fast-paced digital era, data is generated at an unprecedented rate from various sources such as sensors, social media, clickstreams, and IoT devices. Traditional batch processing methods are no longer sufficient to handle the velocity and volume of this data. Stream processing, on the other hand, allows for the continuous ingestion, processing, and analysis of data as it arrives, providing timely insights and enabling immediate actions.
At its core, stream processing involves the continuous processing of data records, often referred to as events or messages, in a real-time manner. These events are typically small and self-contained units of data that are processed individually or in small batches. Stream processing frameworks, such as Apache Kafka, Apache Flink, and Apache Storm, provide the necessary tools and infrastructure to handle the complexities of stream processing.
One of the key advantages of stream processing is its ability to handle data in motion. Unlike batch processing, which deals with static datasets, stream processing allows for the analysis of data as it flows, enabling organizations to detect patterns, anomalies, and trends in real-time. This real-time analysis is particularly valuable in use cases where immediate action is required, such as fraud detection, real-time monitoring, and predictive maintenance.
Stream processing also offers low-latency processing, as it eliminates the need to wait for data to accumulate before processing. This near-instantaneous processing allows organizations to respond swiftly to changing conditions and make data-driven decisions without delay. Moreover, stream processing enables organizations to continuously update and refine their models and algorithms, ensuring that they stay up-to-date with evolving data patterns.
In addition to real-time analysis, stream processing provides the capability to perform various operations on data streams. These operations include filtering, aggregating, transforming, joining, and enriching data streams. These operations can be applied to both raw data streams and derived streams, allowing organizations to derive meaningful insights from complex data sources.
Stream processing in big data has numerous applications across industries. In finance, it can be used for real-time risk analysis, fraud detection, and algorithmic trading. In retail, it can enable personalized marketing, inventory management, and supply chain optimization. In healthcare, it can facilitate real-time patient monitoring, disease surveillance, and drug discovery. These are just a few examples, and the potential use cases of stream processing are vast and diverse.
In conclusion, stream processing in big data is a powerful approach that enables organizations to extract real-time insights from continuous data streams. By processing data in motion, organizations can make timely decisions, respond swiftly to changing conditions, and gain a competitive edge in today's data-driven world. With the advancements in stream processing frameworks and technologies, organizations can harness the full potential of big data and unlock valuable insights that drive innovation and growth.
In today's fast-paced digital era, data is generated at an unprecedented rate from various sources such as sensors, social media, clickstreams, and IoT devices. Traditional batch processing methods are no longer sufficient to handle the velocity and volume of this data. Stream processing, on the other hand, allows for the continuous ingestion, processing, and analysis of data as it arrives, providing timely insights and enabling immediate actions.
At its core, stream processing involves the continuous processing of data records, often referred to as events or messages, in a real-time manner. These events are typically small and self-contained units of data that are processed individually or in small batches. Stream processing frameworks, such as Apache Kafka, Apache Flink, and Apache Storm, provide the necessary tools and infrastructure to handle the complexities of stream processing.
One of the key advantages of stream processing is its ability to handle data in motion. Unlike batch processing, which deals with static datasets, stream processing allows for the analysis of data as it flows, enabling organizations to detect patterns, anomalies, and trends in real-time. This real-time analysis is particularly valuable in use cases where immediate action is required, such as fraud detection, real-time monitoring, and predictive maintenance.
Stream processing also offers low-latency processing, as it eliminates the need to wait for data to accumulate before processing. This near-instantaneous processing allows organizations to respond swiftly to changing conditions and make data-driven decisions without delay. Moreover, stream processing enables organizations to continuously update and refine their models and algorithms, ensuring that they stay up-to-date with evolving data patterns.
In addition to real-time analysis, stream processing provides the capability to perform various operations on data streams. These operations include filtering, aggregating, transforming, joining, and enriching data streams. These operations can be applied to both raw data streams and derived streams, allowing organizations to derive meaningful insights from complex data sources.
Stream processing in big data has numerous applications across industries. In finance, it can be used for real-time risk analysis, fraud detection, and algorithmic trading. In retail, it can enable personalized marketing, inventory management, and supply chain optimization. In healthcare, it can facilitate real-time patient monitoring, disease surveillance, and drug discovery. These are just a few examples, and the potential use cases of stream processing are vast and diverse.
In conclusion, stream processing in big data is a powerful approach that enables organizations to extract real-time insights from continuous data streams. By processing data in motion, organizations can make timely decisions, respond swiftly to changing conditions, and gain a competitive edge in today's data-driven world. With the advancements in stream processing frameworks and technologies, organizations can harness the full potential of big data and unlock valuable insights that drive innovation and growth.