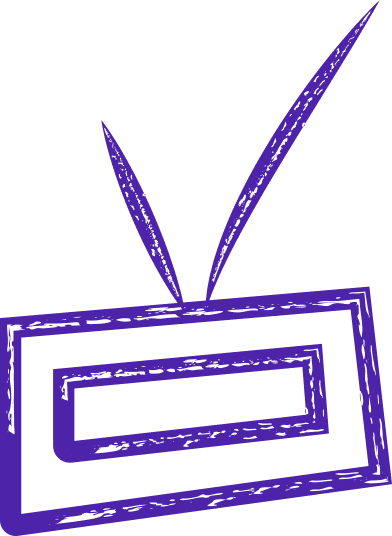
what is statistical machine translation
Statistical Machine Translation
Statistical Machine Translation (SMT) is a cutting-edge technology that enables the automatic translation of text from one language to another. It is a subfield of machine translation that utilizes statistical models and algorithms to generate accurate and fluent translations.
SMT relies on a vast amount of bilingual text data, known as parallel corpora, to train its models. These corpora consist of pairs of sentences or documents in the source language and their corresponding translations in the target language. By analyzing these bilingual texts, SMT algorithms learn patterns and statistical relationships between words, phrases, and sentence structures in different languages.
The core concept behind statistical machine translation is based on the principle of probability. SMT algorithms calculate the likelihood of a particular translation given a specific source sentence, and then generate the translation that has the highest probability. This approach allows SMT systems to generate translations that are contextually accurate and idiomatic, capturing the nuances and subtleties of the source language.
One of the key advantages of statistical machine translation is its ability to adapt and improve over time. As more bilingual data becomes available, SMT models can be retrained to incorporate this new information, resulting in enhanced translation quality. This adaptability makes SMT a valuable tool for industries such as e-commerce, travel, and global communication, where accurate and efficient translation is essential.
However, it is important to note that statistical machine translation is not without its limitations. SMT models heavily rely on the quality and quantity of the training data. If the parallel corpora used for training are limited or of poor quality, the translation output may suffer from inaccuracies and inconsistencies. Additionally, SMT may struggle with translating rare or domain-specific terminology, as it relies on statistical patterns that may not be well-represented in the training data.
To overcome these limitations, researchers and developers have been exploring various approaches to improve statistical machine translation. This includes incorporating linguistic knowledge and rule-based systems into the statistical models, as well as leveraging neural networks and deep learning techniques to enhance the translation quality.
In conclusion, statistical machine translation is a powerful technology that revolutionizes the way we communicate across different languages. By harnessing the power of statistics and probability, SMT systems can generate translations that are both accurate and fluent. While there are challenges to overcome, ongoing advancements in this field continue to push the boundaries of machine translation, making it an indispensable tool in our increasingly globalized world.
SMT relies on a vast amount of bilingual text data, known as parallel corpora, to train its models. These corpora consist of pairs of sentences or documents in the source language and their corresponding translations in the target language. By analyzing these bilingual texts, SMT algorithms learn patterns and statistical relationships between words, phrases, and sentence structures in different languages.
The core concept behind statistical machine translation is based on the principle of probability. SMT algorithms calculate the likelihood of a particular translation given a specific source sentence, and then generate the translation that has the highest probability. This approach allows SMT systems to generate translations that are contextually accurate and idiomatic, capturing the nuances and subtleties of the source language.
One of the key advantages of statistical machine translation is its ability to adapt and improve over time. As more bilingual data becomes available, SMT models can be retrained to incorporate this new information, resulting in enhanced translation quality. This adaptability makes SMT a valuable tool for industries such as e-commerce, travel, and global communication, where accurate and efficient translation is essential.
However, it is important to note that statistical machine translation is not without its limitations. SMT models heavily rely on the quality and quantity of the training data. If the parallel corpora used for training are limited or of poor quality, the translation output may suffer from inaccuracies and inconsistencies. Additionally, SMT may struggle with translating rare or domain-specific terminology, as it relies on statistical patterns that may not be well-represented in the training data.
To overcome these limitations, researchers and developers have been exploring various approaches to improve statistical machine translation. This includes incorporating linguistic knowledge and rule-based systems into the statistical models, as well as leveraging neural networks and deep learning techniques to enhance the translation quality.
In conclusion, statistical machine translation is a powerful technology that revolutionizes the way we communicate across different languages. By harnessing the power of statistics and probability, SMT systems can generate translations that are both accurate and fluent. While there are challenges to overcome, ongoing advancements in this field continue to push the boundaries of machine translation, making it an indispensable tool in our increasingly globalized world.