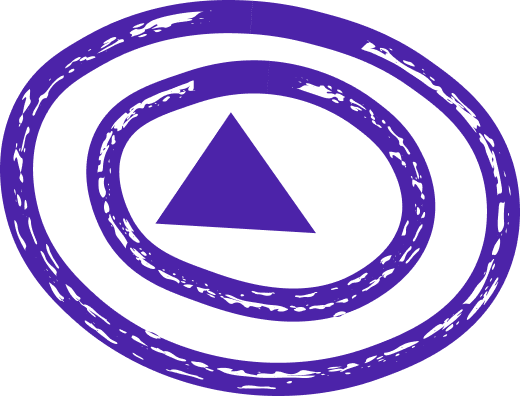
what is spectral analysis in signal processing
Spectral Analysis in Signal Processing
Spectral Analysis in Signal Processing is a fundamental technique used to analyze and understand the frequency content of a given signal. It plays a crucial role in various fields, including telecommunications, audio processing, image processing, and radar systems. By decomposing a signal into its constituent frequencies, spectral analysis enables researchers, engineers, and scientists to extract valuable information, detect patterns, and make informed decisions.
In its essence, spectral analysis involves transforming a time-domain signal into the frequency domain. This transformation is achieved using mathematical tools such as the Fourier Transform or the Discrete Fourier Transform (DFT). These transforms convert a signal from its original representation, where it is represented as a function of time, into a representation where it is expressed in terms of its frequency components.
The resulting frequency-domain representation of a signal is known as its spectrum. The spectrum provides a detailed description of the signal's frequency content, revealing the presence, strength, and distribution of various frequencies within the signal. This information is crucial for understanding the characteristics of the signal and extracting meaningful insights.
Spectral analysis techniques can be broadly categorized into two main types: non-parametric and parametric methods. Non-parametric methods, such as the Fast Fourier Transform (FFT), are widely used due to their simplicity and efficiency. These methods provide an estimate of the power spectral density (PSD) of a signal, which represents the distribution of power across different frequencies.
On the other hand, parametric methods assume a specific mathematical model for the signal and estimate its parameters. These methods are often more accurate but require prior knowledge or assumptions about the signal. Examples of parametric methods include autoregressive (AR) modeling, moving average (MA) modeling, and autoregressive moving average (ARMA) modeling.
Spectral analysis has numerous practical applications. In telecommunications, it is used for channel estimation, equalization, and modulation recognition. In audio processing, it is employed for speech recognition, noise cancellation, and audio compression. In image processing, it is utilized for image enhancement, pattern recognition, and object detection. Additionally, spectral analysis is crucial in radar systems for target detection, tracking, and classification.
In conclusion, spectral analysis in signal processing is a powerful technique that allows us to gain insights into the frequency content of a signal. By transforming signals from the time domain to the frequency domain, spectral analysis enables us to understand and manipulate signals more effectively. Its applications span across various industries and disciplines, making it an indispensable tool for researchers, engineers, and scientists working with signals and systems.
In its essence, spectral analysis involves transforming a time-domain signal into the frequency domain. This transformation is achieved using mathematical tools such as the Fourier Transform or the Discrete Fourier Transform (DFT). These transforms convert a signal from its original representation, where it is represented as a function of time, into a representation where it is expressed in terms of its frequency components.
The resulting frequency-domain representation of a signal is known as its spectrum. The spectrum provides a detailed description of the signal's frequency content, revealing the presence, strength, and distribution of various frequencies within the signal. This information is crucial for understanding the characteristics of the signal and extracting meaningful insights.
Spectral analysis techniques can be broadly categorized into two main types: non-parametric and parametric methods. Non-parametric methods, such as the Fast Fourier Transform (FFT), are widely used due to their simplicity and efficiency. These methods provide an estimate of the power spectral density (PSD) of a signal, which represents the distribution of power across different frequencies.
On the other hand, parametric methods assume a specific mathematical model for the signal and estimate its parameters. These methods are often more accurate but require prior knowledge or assumptions about the signal. Examples of parametric methods include autoregressive (AR) modeling, moving average (MA) modeling, and autoregressive moving average (ARMA) modeling.
Spectral analysis has numerous practical applications. In telecommunications, it is used for channel estimation, equalization, and modulation recognition. In audio processing, it is employed for speech recognition, noise cancellation, and audio compression. In image processing, it is utilized for image enhancement, pattern recognition, and object detection. Additionally, spectral analysis is crucial in radar systems for target detection, tracking, and classification.
In conclusion, spectral analysis in signal processing is a powerful technique that allows us to gain insights into the frequency content of a signal. By transforming signals from the time domain to the frequency domain, spectral analysis enables us to understand and manipulate signals more effectively. Its applications span across various industries and disciplines, making it an indispensable tool for researchers, engineers, and scientists working with signals and systems.