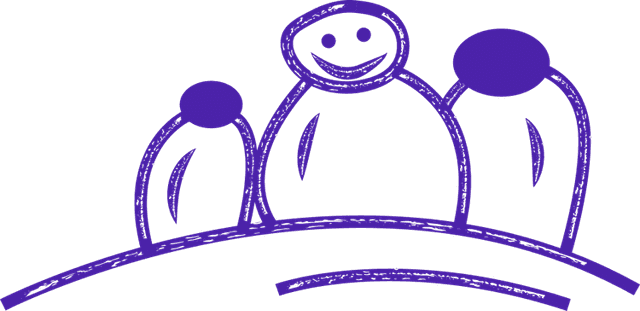
what is reinforcement learning
Reinforcement Learning
Reinforcement learning is a dynamic and powerful subfield of artificial intelligence (AI) that focuses on enabling machines to learn and make decisions through interactions with their environment. It is based on the concept of an agent, which is an entity that perceives its surroundings, takes actions, and receives feedback or rewards from the environment. This feedback is used to guide the agent's learning process and improve its decision-making abilities over time.
Unlike other machine learning approaches that rely on labeled data, reinforcement learning operates in an environment where the agent must learn through trial and error. The agent explores its environment by taking actions and receives positive or negative rewards based on the outcomes of those actions. By maximizing the cumulative rewards obtained over multiple interactions, the agent aims to find the optimal policy or strategy that leads to the highest possible long-term reward.
The core idea behind reinforcement learning is to use a combination of exploration and exploitation to strike a balance between trying out new actions and exploiting the knowledge gained from previous experiences. Through the use of various algorithms, such as Q-learning, policy gradients, and Monte Carlo methods, reinforcement learning allows agents to learn from their mistakes and adapt their behavior to achieve desired goals.
Reinforcement learning has found applications in a wide range of domains, including robotics, game playing, autonomous vehicles, recommendation systems, and finance. For example, in robotics, reinforcement learning can be used to teach a robot how to grasp objects or navigate through complex environments. In game playing, reinforcement learning has been instrumental in achieving superhuman performance in games like chess, Go, and poker.
One of the key advantages of reinforcement learning is its ability to handle environments with unknown dynamics or complex, high-dimensional state spaces. By learning directly from interactions, reinforcement learning algorithms can adapt to changing environments and learn optimal strategies even in the absence of prior knowledge.
However, reinforcement learning also poses several challenges. The exploration-exploitation trade-off, for instance, requires careful tuning to prevent the agent from getting stuck in suboptimal behaviors. Additionally, the training process can be computationally expensive and time-consuming, especially for complex tasks.
In conclusion, reinforcement learning is a fundamental and exciting area of AI that enables machines to learn and make decisions through interactions with their environment. By combining exploration and exploitation, reinforcement learning allows agents to learn from their experiences and improve their decision-making abilities over time. With its wide range of applications and potential for solving complex problems, reinforcement learning is poised to revolutionize various industries and pave the way for more intelligent and autonomous systems.
Unlike other machine learning approaches that rely on labeled data, reinforcement learning operates in an environment where the agent must learn through trial and error. The agent explores its environment by taking actions and receives positive or negative rewards based on the outcomes of those actions. By maximizing the cumulative rewards obtained over multiple interactions, the agent aims to find the optimal policy or strategy that leads to the highest possible long-term reward.
The core idea behind reinforcement learning is to use a combination of exploration and exploitation to strike a balance between trying out new actions and exploiting the knowledge gained from previous experiences. Through the use of various algorithms, such as Q-learning, policy gradients, and Monte Carlo methods, reinforcement learning allows agents to learn from their mistakes and adapt their behavior to achieve desired goals.
Reinforcement learning has found applications in a wide range of domains, including robotics, game playing, autonomous vehicles, recommendation systems, and finance. For example, in robotics, reinforcement learning can be used to teach a robot how to grasp objects or navigate through complex environments. In game playing, reinforcement learning has been instrumental in achieving superhuman performance in games like chess, Go, and poker.
One of the key advantages of reinforcement learning is its ability to handle environments with unknown dynamics or complex, high-dimensional state spaces. By learning directly from interactions, reinforcement learning algorithms can adapt to changing environments and learn optimal strategies even in the absence of prior knowledge.
However, reinforcement learning also poses several challenges. The exploration-exploitation trade-off, for instance, requires careful tuning to prevent the agent from getting stuck in suboptimal behaviors. Additionally, the training process can be computationally expensive and time-consuming, especially for complex tasks.
In conclusion, reinforcement learning is a fundamental and exciting area of AI that enables machines to learn and make decisions through interactions with their environment. By combining exploration and exploitation, reinforcement learning allows agents to learn from their experiences and improve their decision-making abilities over time. With its wide range of applications and potential for solving complex problems, reinforcement learning is poised to revolutionize various industries and pave the way for more intelligent and autonomous systems.