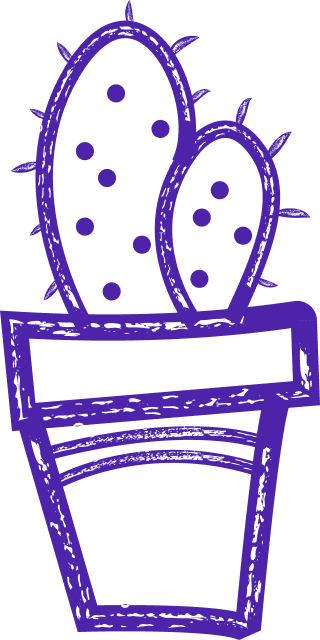
what is predictive modeling in data science
Predictive Modeling in Data Science
Predictive modeling in data science is a powerful technique that utilizes historical data to make informed predictions or forecasts about future events or outcomes. It involves the use of various statistical and machine learning algorithms to analyze patterns, relationships, and trends within a dataset, enabling the generation of reliable predictions.
At its core, predictive modeling aims to understand the underlying patterns and behaviors in the data, enabling data scientists to build accurate models that can predict outcomes with a high degree of certainty. This process typically involves several steps, including data collection, data preprocessing, feature engineering, model selection, model training, and model evaluation.
Data collection is the initial step where relevant data is gathered from various sources, ensuring that it is comprehensive and representative of the problem domain. Once the data is collected, it undergoes preprocessing, which involves cleaning, transforming, and normalizing the data to ensure its quality and consistency.
Feature engineering is a crucial step in predictive modeling, as it involves selecting and creating relevant features or variables from the available data that can significantly impact the prediction accuracy. This step requires domain expertise and a deep understanding of the problem at hand.
Next, data scientists choose an appropriate model based on the specific problem and the nature of the data. There are various algorithms available, such as linear regression, decision trees, random forests, support vector machines, and neural networks, each with its strengths and weaknesses. The selection of the model depends on factors such as interpretability, complexity, scalability, and performance requirements.
Once the model is selected, it is trained using historical data, where the algorithm learns the underlying patterns and relationships between the features and the target variable. This training process involves adjusting the model's parameters to minimize the prediction errors and optimize its performance.
After the model is trained, it is evaluated using a separate dataset, known as the test set, to assess its predictive accuracy. This evaluation helps determine whether the model is overfitting or underfitting the data and provides insights into its generalization capabilities.
Predictive modeling finds applications in various industries and domains, including finance, healthcare, marketing, manufacturing, and many others. It can be used for a wide range of purposes, such as customer segmentation, fraud detection, risk assessment, demand forecasting, recommendation systems, and predictive maintenance.
The success of predictive modeling heavily relies on the quality and quantity of the available data, as well as the expertise of the data scientists involved. Additionally, it is essential to continuously update and refine the models as new data becomes available to ensure their accuracy and relevance.
In conclusion, predictive modeling is a fundamental technique in data science that enables businesses and organizations to make data-driven decisions and gain valuable insights into future events or outcomes. By leveraging historical data and advanced algorithms, predictive modeling empowers businesses to anticipate trends, optimize processes, and enhance decision-making, ultimately leading to improved efficiency and competitive advantage.
At its core, predictive modeling aims to understand the underlying patterns and behaviors in the data, enabling data scientists to build accurate models that can predict outcomes with a high degree of certainty. This process typically involves several steps, including data collection, data preprocessing, feature engineering, model selection, model training, and model evaluation.
Data collection is the initial step where relevant data is gathered from various sources, ensuring that it is comprehensive and representative of the problem domain. Once the data is collected, it undergoes preprocessing, which involves cleaning, transforming, and normalizing the data to ensure its quality and consistency.
Feature engineering is a crucial step in predictive modeling, as it involves selecting and creating relevant features or variables from the available data that can significantly impact the prediction accuracy. This step requires domain expertise and a deep understanding of the problem at hand.
Next, data scientists choose an appropriate model based on the specific problem and the nature of the data. There are various algorithms available, such as linear regression, decision trees, random forests, support vector machines, and neural networks, each with its strengths and weaknesses. The selection of the model depends on factors such as interpretability, complexity, scalability, and performance requirements.
Once the model is selected, it is trained using historical data, where the algorithm learns the underlying patterns and relationships between the features and the target variable. This training process involves adjusting the model's parameters to minimize the prediction errors and optimize its performance.
After the model is trained, it is evaluated using a separate dataset, known as the test set, to assess its predictive accuracy. This evaluation helps determine whether the model is overfitting or underfitting the data and provides insights into its generalization capabilities.
Predictive modeling finds applications in various industries and domains, including finance, healthcare, marketing, manufacturing, and many others. It can be used for a wide range of purposes, such as customer segmentation, fraud detection, risk assessment, demand forecasting, recommendation systems, and predictive maintenance.
The success of predictive modeling heavily relies on the quality and quantity of the available data, as well as the expertise of the data scientists involved. Additionally, it is essential to continuously update and refine the models as new data becomes available to ensure their accuracy and relevance.
In conclusion, predictive modeling is a fundamental technique in data science that enables businesses and organizations to make data-driven decisions and gain valuable insights into future events or outcomes. By leveraging historical data and advanced algorithms, predictive modeling empowers businesses to anticipate trends, optimize processes, and enhance decision-making, ultimately leading to improved efficiency and competitive advantage.