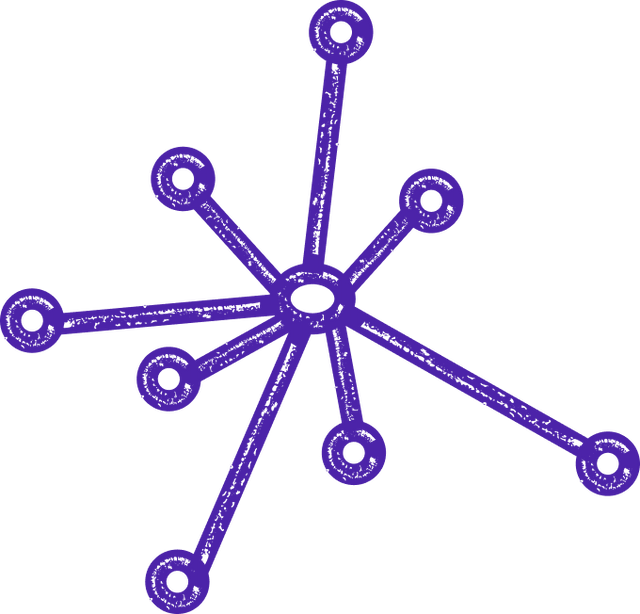
what is markov decision processes
Markov Decision Processes
Markov Decision Processes (MDPs) are a mathematical framework used to model decision-making problems in various fields, including artificial intelligence, operations research, and economics. MDPs provide a structured way to analyze and solve problems that involve sequential decision-making in uncertain environments.
In an MDP, a decision-maker, often referred to as an agent, interacts with an environment over a series of discrete time steps. At each time step, the agent observes the current state of the environment and selects an action to take. The environment responds by transitioning to a new state and providing a reward to the agent. The goal of the agent is to maximize the cumulative reward it receives over time.
The key characteristic of MDPs is the Markov property, which states that the future state and reward only depend on the current state and action, and not on the history of previous states and actions. This property allows for efficient computation and analysis of MDPs.
MDPs are defined by a set of states, actions, transition probabilities, and rewards. The state space represents all possible states the environment can be in, while the action space represents all possible actions the agent can take. Transition probabilities describe the likelihood of transitioning from one state to another after taking a particular action. Rewards quantify the desirability of being in a certain state or taking a specific action.
Solving an MDP involves finding a policy, which is a mapping from states to actions, that maximizes the expected cumulative reward. There are several algorithms and techniques available to solve MDPs, including dynamic programming, reinforcement learning, and Monte Carlo methods. These approaches aim to find an optimal policy that maximizes the expected long-term reward.
MDPs have numerous real-world applications, such as autonomous robot navigation, resource allocation, inventory control, and route planning. By using MDPs, decision-makers can make informed choices in uncertain and dynamic environments, leading to improved efficiency, resource utilization, and overall performance.
In conclusion, Markov Decision Processes provide a powerful framework for modeling and solving decision-making problems in uncertain environments. By considering the Markov property, MDPs allow decision-makers to navigate complex scenarios and optimize their actions to achieve long-term goals. With their broad range of applications, MDPs play a crucial role in the development of intelligent systems and decision support tools.
In an MDP, a decision-maker, often referred to as an agent, interacts with an environment over a series of discrete time steps. At each time step, the agent observes the current state of the environment and selects an action to take. The environment responds by transitioning to a new state and providing a reward to the agent. The goal of the agent is to maximize the cumulative reward it receives over time.
The key characteristic of MDPs is the Markov property, which states that the future state and reward only depend on the current state and action, and not on the history of previous states and actions. This property allows for efficient computation and analysis of MDPs.
MDPs are defined by a set of states, actions, transition probabilities, and rewards. The state space represents all possible states the environment can be in, while the action space represents all possible actions the agent can take. Transition probabilities describe the likelihood of transitioning from one state to another after taking a particular action. Rewards quantify the desirability of being in a certain state or taking a specific action.
Solving an MDP involves finding a policy, which is a mapping from states to actions, that maximizes the expected cumulative reward. There are several algorithms and techniques available to solve MDPs, including dynamic programming, reinforcement learning, and Monte Carlo methods. These approaches aim to find an optimal policy that maximizes the expected long-term reward.
MDPs have numerous real-world applications, such as autonomous robot navigation, resource allocation, inventory control, and route planning. By using MDPs, decision-makers can make informed choices in uncertain and dynamic environments, leading to improved efficiency, resource utilization, and overall performance.
In conclusion, Markov Decision Processes provide a powerful framework for modeling and solving decision-making problems in uncertain environments. By considering the Markov property, MDPs allow decision-makers to navigate complex scenarios and optimize their actions to achieve long-term goals. With their broad range of applications, MDPs play a crucial role in the development of intelligent systems and decision support tools.