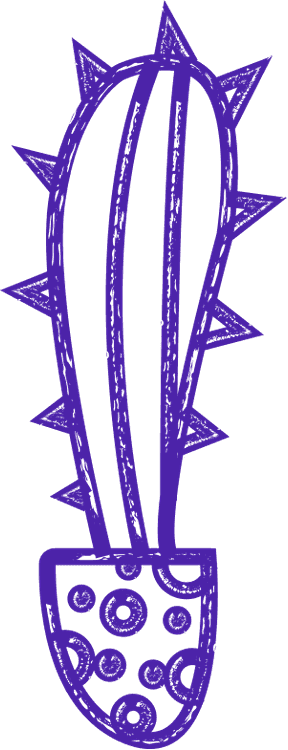
what is latent semantic analysis
Latent Semantic Analysis
Latent Semantic Analysis (LSA) is a powerful statistical method used in natural language processing and information retrieval to uncover the hidden meaning and semantic relationships between words and documents. By analyzing the patterns of word usage and co-occurrence within a large corpus of text, LSA aims to capture the underlying semantic structure of language.
LSA operates on the principle that words that appear in similar contexts tend to have similar meanings. It leverages the mathematical technique of singular value decomposition (SVD) to convert a matrix of word frequencies into a lower-dimensional representation, where the latent semantic relationships are revealed. This transformation allows LSA to identify the conceptual associations between words and documents, even when they may not share the exact same words.
The process of Latent Semantic Analysis involves several steps. First, a large collection of text documents is gathered and preprocessed to remove noise and irrelevant information. This preprocessing may include tasks such as tokenization, stop-word removal, and stemming. Next, a term-document matrix is constructed, where each row represents a unique word, each column represents a document, and the cells contain the frequency or weight of the word in the respective document.
Once the term-document matrix is created, LSA applies SVD to decompose it into three matrices: U, Σ, and V. The matrix U represents the relationship between words and latent semantic concepts, while the matrix V represents the relationship between documents and these concepts. The diagonal matrix Σ contains the singular values, which indicate the importance of each latent concept.
LSA allows for the reduction of the dimensionality of the original data, as the number of singular values retained can be adjusted. By selecting a smaller number of singular values, the resulting representation captures the most salient semantic relationships while filtering out noise and irrelevant information. This dimensionality reduction enables efficient and effective information retrieval and text mining tasks.
The applications of Latent Semantic Analysis are diverse and far-reaching. In information retrieval, LSA can be used to improve search engines by matching user queries with relevant documents based on their semantic similarity. It can also be utilized in text classification, clustering, and summarization tasks, where it helps in identifying related documents and extracting key themes.
Furthermore, LSA has found applications in recommendation systems, where it can identify similar items or content based on their latent semantic features. It has also been used in machine translation, sentiment analysis, and question-answering systems, enhancing their accuracy and performance.
In conclusion, Latent Semantic Analysis is a sophisticated technique that uncovers the hidden semantic relationships between words and documents. By leveraging statistical methods and matrix decomposition, LSA provides a powerful tool for understanding and processing natural language. Its ability to capture the underlying meaning of text has made it an invaluable asset in various fields, revolutionizing information retrieval and enabling advanced language processing applications.
LSA operates on the principle that words that appear in similar contexts tend to have similar meanings. It leverages the mathematical technique of singular value decomposition (SVD) to convert a matrix of word frequencies into a lower-dimensional representation, where the latent semantic relationships are revealed. This transformation allows LSA to identify the conceptual associations between words and documents, even when they may not share the exact same words.
The process of Latent Semantic Analysis involves several steps. First, a large collection of text documents is gathered and preprocessed to remove noise and irrelevant information. This preprocessing may include tasks such as tokenization, stop-word removal, and stemming. Next, a term-document matrix is constructed, where each row represents a unique word, each column represents a document, and the cells contain the frequency or weight of the word in the respective document.
Once the term-document matrix is created, LSA applies SVD to decompose it into three matrices: U, Σ, and V. The matrix U represents the relationship between words and latent semantic concepts, while the matrix V represents the relationship between documents and these concepts. The diagonal matrix Σ contains the singular values, which indicate the importance of each latent concept.
LSA allows for the reduction of the dimensionality of the original data, as the number of singular values retained can be adjusted. By selecting a smaller number of singular values, the resulting representation captures the most salient semantic relationships while filtering out noise and irrelevant information. This dimensionality reduction enables efficient and effective information retrieval and text mining tasks.
The applications of Latent Semantic Analysis are diverse and far-reaching. In information retrieval, LSA can be used to improve search engines by matching user queries with relevant documents based on their semantic similarity. It can also be utilized in text classification, clustering, and summarization tasks, where it helps in identifying related documents and extracting key themes.
Furthermore, LSA has found applications in recommendation systems, where it can identify similar items or content based on their latent semantic features. It has also been used in machine translation, sentiment analysis, and question-answering systems, enhancing their accuracy and performance.
In conclusion, Latent Semantic Analysis is a sophisticated technique that uncovers the hidden semantic relationships between words and documents. By leveraging statistical methods and matrix decomposition, LSA provides a powerful tool for understanding and processing natural language. Its ability to capture the underlying meaning of text has made it an invaluable asset in various fields, revolutionizing information retrieval and enabling advanced language processing applications.