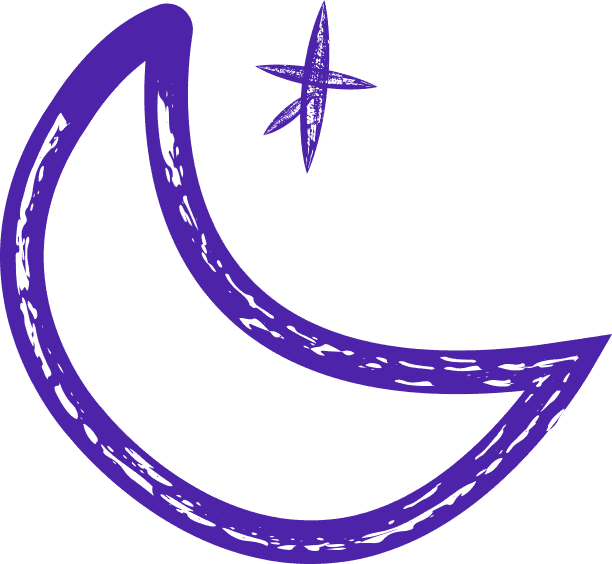
what is information entropy
Information Entropy
Information entropy is a concept that originated in information theory and is used to measure the uncertainty or randomness of a given set of information. It is a measure of the amount of information contained in a message or signal, and it is typically expressed in bits.
In essence, information entropy can be thought of as a measure of the amount of surprise or unpredictability in a message. The more unexpected or surprising a message is, the higher its entropy will be.
Information entropy is closely related to the concept of probability. If a message is very likely to occur, then it contains less entropy than a message that is very unlikely to occur. For example, if we flip a fair coin, the result is equally likely to be heads or tails. Therefore, the entropy of the message is one bit, because there are two equally likely outcomes. However, if we flip a biased coin that is more likely to land on heads, the entropy of the message will be less than one bit, because the outcome is less uncertain.
Information entropy can be applied to a wide range of fields, including computer science, physics, and statistics. In computer science, it is used to measure the amount of information that can be stored in a digital system, such as a hard drive or a memory card. In physics, it is used to describe the behavior of particles and the flow of energy in a system. In statistics, it is used to measure the amount of uncertainty or randomness in a data set.
One of the most important applications of information entropy is in the field of data compression. By analyzing the entropy of a message, it is possible to identify patterns and redundancies in the data that can be exploited to compress the message without losing any information. This is the basis for many modern compression algorithms, such as ZIP and JPEG.
Information entropy is also closely related to the concept of information gain, which is used in machine learning and data analysis. Information gain is a measure of how much a given feature or attribute contributes to the overall entropy of a data set. By analyzing the information gain of different features, it is possible to identify the most important factors that contribute to a particular outcome or result.
In conclusion, information entropy is a powerful concept that has applications in a wide range of fields. It is a measure of the uncertainty or randomness of a message, and it can be used to identify patterns and redundancies in data, compress messages, and analyze the behavior of complex systems. Understanding information entropy is essential for anyone working in fields such as computer science, physics, statistics, and data analysis.
In essence, information entropy can be thought of as a measure of the amount of surprise or unpredictability in a message. The more unexpected or surprising a message is, the higher its entropy will be.
Information entropy is closely related to the concept of probability. If a message is very likely to occur, then it contains less entropy than a message that is very unlikely to occur. For example, if we flip a fair coin, the result is equally likely to be heads or tails. Therefore, the entropy of the message is one bit, because there are two equally likely outcomes. However, if we flip a biased coin that is more likely to land on heads, the entropy of the message will be less than one bit, because the outcome is less uncertain.
Information entropy can be applied to a wide range of fields, including computer science, physics, and statistics. In computer science, it is used to measure the amount of information that can be stored in a digital system, such as a hard drive or a memory card. In physics, it is used to describe the behavior of particles and the flow of energy in a system. In statistics, it is used to measure the amount of uncertainty or randomness in a data set.
One of the most important applications of information entropy is in the field of data compression. By analyzing the entropy of a message, it is possible to identify patterns and redundancies in the data that can be exploited to compress the message without losing any information. This is the basis for many modern compression algorithms, such as ZIP and JPEG.
Information entropy is also closely related to the concept of information gain, which is used in machine learning and data analysis. Information gain is a measure of how much a given feature or attribute contributes to the overall entropy of a data set. By analyzing the information gain of different features, it is possible to identify the most important factors that contribute to a particular outcome or result.
In conclusion, information entropy is a powerful concept that has applications in a wide range of fields. It is a measure of the uncertainty or randomness of a message, and it can be used to identify patterns and redundancies in data, compress messages, and analyze the behavior of complex systems. Understanding information entropy is essential for anyone working in fields such as computer science, physics, statistics, and data analysis.