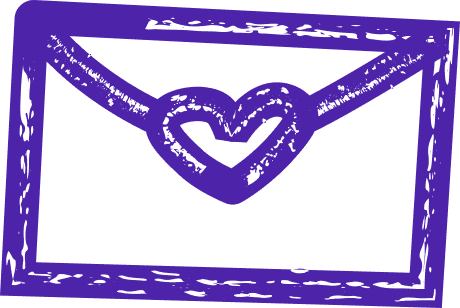
what is federated learning
Federated Learning
Federated Learning is a machine learning technique that allows multiple devices to collaboratively train a model without the need for data to be centralized in one location. This method of training is becoming increasingly popular in the age of big data, where data privacy and security are paramount concerns.
In traditional machine learning, all data is sent to a central location, where it is processed and used to train a model. However, this approach presents significant privacy and security risks, as sensitive data can be compromised in transit or at rest. Federated Learning overcomes these challenges by allowing data to remain on the device, while the model is trained collaboratively.
The process of Federated Learning begins with a central server that sends a model to a group of devices, such as smartphones or IoT devices. Each device then trains the model using its local data, and sends the updated model back to the server. The server aggregates the updates and sends a new model to the devices, repeating the process until the model is sufficiently trained.
Federated Learning offers several advantages over traditional machine learning. First and foremost, it preserves data privacy, as data remains on the device and is not shared with the central server or other devices. This is particularly important for sensitive data such as health records or financial information.
Secondly, Federated Learning allows for faster model training, as the process can be distributed across multiple devices. This means that models can be trained more quickly and efficiently, which is essential for real-time applications such as fraud detection or autonomous vehicles.
Finally, Federated Learning is more scalable than traditional machine learning, as it can handle large volumes of data without requiring a centralized infrastructure. This makes it ideal for applications where data is generated at the edge, such as IoT devices or mobile phones.
Overall, Federated Learning is a powerful technique that is transforming the field of machine learning. By enabling collaborative model training without compromising data privacy, it has the potential to unlock new applications and insights across a wide range of industries.
In traditional machine learning, all data is sent to a central location, where it is processed and used to train a model. However, this approach presents significant privacy and security risks, as sensitive data can be compromised in transit or at rest. Federated Learning overcomes these challenges by allowing data to remain on the device, while the model is trained collaboratively.
The process of Federated Learning begins with a central server that sends a model to a group of devices, such as smartphones or IoT devices. Each device then trains the model using its local data, and sends the updated model back to the server. The server aggregates the updates and sends a new model to the devices, repeating the process until the model is sufficiently trained.
Federated Learning offers several advantages over traditional machine learning. First and foremost, it preserves data privacy, as data remains on the device and is not shared with the central server or other devices. This is particularly important for sensitive data such as health records or financial information.
Secondly, Federated Learning allows for faster model training, as the process can be distributed across multiple devices. This means that models can be trained more quickly and efficiently, which is essential for real-time applications such as fraud detection or autonomous vehicles.
Finally, Federated Learning is more scalable than traditional machine learning, as it can handle large volumes of data without requiring a centralized infrastructure. This makes it ideal for applications where data is generated at the edge, such as IoT devices or mobile phones.
Overall, Federated Learning is a powerful technique that is transforming the field of machine learning. By enabling collaborative model training without compromising data privacy, it has the potential to unlock new applications and insights across a wide range of industries.