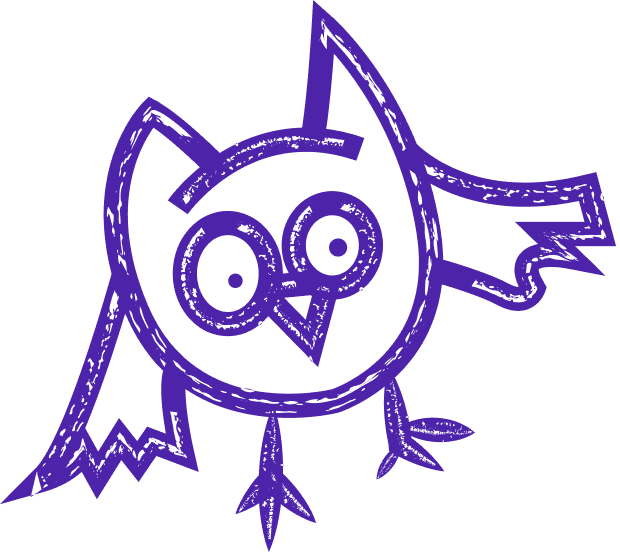
what is feature engineering in machine learning
Feature Engineering in Machine Learning
Feature engineering is a crucial aspect of machine learning, playing a significant role in enhancing the performance and accuracy of predictive models. It refers to the process of transforming raw data into meaningful features that can be easily understood and utilized by machine learning algorithms. In simpler terms, feature engineering involves selecting, creating, and transforming variables from the available data to facilitate the learning process of a machine learning model.
The importance of feature engineering lies in the fact that the quality and relevance of the features used directly impact the model's ability to make accurate predictions or classifications. By carefully selecting and engineering features, data scientists can extract valuable insights and patterns from the data, enabling the model to generalize well on unseen data.
There are several techniques involved in feature engineering, each serving a specific purpose. One common technique is feature selection, where relevant features are chosen from the available dataset based on their importance and contribution to the target variable. This helps in reducing the dimensionality of the data, eliminating irrelevant or redundant features, and improving the model's efficiency and interpretability.
Another technique is feature extraction, which involves creating new features by combining or transforming existing variables. This can be done through mathematical operations, such as scaling, normalization, logarithmic transformations, or polynomial expansions. Feature extraction helps in capturing complex relationships and patterns that may not be evident in the original data, thus enhancing the model's ability to capture important information.
Furthermore, feature engineering can also involve domain-specific knowledge and expertise. This means that experts with a deep understanding of the problem domain can engineer features that are specifically tailored to the problem at hand. For example, in natural language processing tasks, features like word frequency, n-grams, or sentiment scores can be engineered to capture the semantic meaning of text data.
It is worth noting that feature engineering requires iterative experimentation and domain knowledge. Data scientists often need to iterate through multiple rounds of feature engineering, model building, and evaluation to find the optimal set of features that yield the best performance. This process can be time-consuming and resource-intensive, but it is a crucial step to ensure the success of machine learning models.
In summary, feature engineering is a fundamental step in machine learning that involves transforming raw data into meaningful features. It encompasses techniques like feature selection, feature extraction, and domain-specific knowledge to enhance the performance and accuracy of predictive models. By investing time and effort into feature engineering, data scientists can unlock the full potential of their data and build robust and accurate machine learning models.
The importance of feature engineering lies in the fact that the quality and relevance of the features used directly impact the model's ability to make accurate predictions or classifications. By carefully selecting and engineering features, data scientists can extract valuable insights and patterns from the data, enabling the model to generalize well on unseen data.
There are several techniques involved in feature engineering, each serving a specific purpose. One common technique is feature selection, where relevant features are chosen from the available dataset based on their importance and contribution to the target variable. This helps in reducing the dimensionality of the data, eliminating irrelevant or redundant features, and improving the model's efficiency and interpretability.
Another technique is feature extraction, which involves creating new features by combining or transforming existing variables. This can be done through mathematical operations, such as scaling, normalization, logarithmic transformations, or polynomial expansions. Feature extraction helps in capturing complex relationships and patterns that may not be evident in the original data, thus enhancing the model's ability to capture important information.
Furthermore, feature engineering can also involve domain-specific knowledge and expertise. This means that experts with a deep understanding of the problem domain can engineer features that are specifically tailored to the problem at hand. For example, in natural language processing tasks, features like word frequency, n-grams, or sentiment scores can be engineered to capture the semantic meaning of text data.
It is worth noting that feature engineering requires iterative experimentation and domain knowledge. Data scientists often need to iterate through multiple rounds of feature engineering, model building, and evaluation to find the optimal set of features that yield the best performance. This process can be time-consuming and resource-intensive, but it is a crucial step to ensure the success of machine learning models.
In summary, feature engineering is a fundamental step in machine learning that involves transforming raw data into meaningful features. It encompasses techniques like feature selection, feature extraction, and domain-specific knowledge to enhance the performance and accuracy of predictive models. By investing time and effort into feature engineering, data scientists can unlock the full potential of their data and build robust and accurate machine learning models.