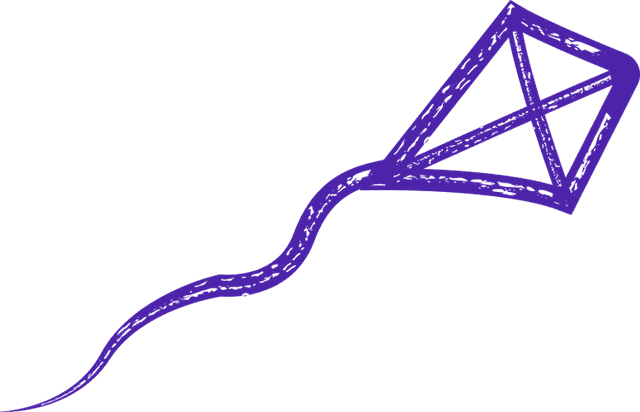
what is deep learning network architectures
What is Deep Learning Network Architectures - Startup House
Deep learning network architectures refer to the design and structure of artificial neural networks that are used in deep learning models. Deep learning is a subset of machine learning that involves training neural networks with multiple layers to learn complex patterns and relationships in data. Deep learning network architectures play a crucial role in determining the performance and capabilities of deep learning models.
There are several key components that make up deep learning network architectures, including the number of layers, the types of layers used, the activation functions, and the connections between layers. The number of layers in a deep learning network is often referred to as its depth, and deeper networks are generally able to learn more complex patterns in data. The types of layers used in a network can include convolutional layers for image data, recurrent layers for sequential data, and dense layers for general-purpose learning. Activation functions are used to introduce non-linearity into the network, allowing it to learn more complex relationships in the data.
One of the most common deep learning network architectures is the convolutional neural network (CNN), which is widely used for tasks such as image recognition and object detection. CNNs typically consist of multiple convolutional layers followed by pooling layers to reduce the spatial dimensions of the data. Another popular architecture is the recurrent neural network (RNN), which is designed to handle sequential data such as text or time series data. RNNs use feedback loops to process sequences of data and are commonly used for tasks such as natural language processing and speech recognition.
In recent years, there has been a growing interest in more complex deep learning network architectures such as deep residual networks (ResNets) and transformer networks. ResNets use skip connections to allow information to flow more easily through the network, enabling the training of very deep networks with hundreds of layers. Transformer networks, on the other hand, are designed specifically for processing sequential data and have been highly successful in tasks such as machine translation and language modeling.
Overall, deep learning network architectures are constantly evolving as researchers continue to explore new techniques and approaches to improve the performance of deep learning models. By understanding the principles behind these architectures and how they can be applied to different types of data, researchers and practitioners can develop more effective deep learning models for a wide range of applications.
There are several key components that make up deep learning network architectures, including the number of layers, the types of layers used, the activation functions, and the connections between layers. The number of layers in a deep learning network is often referred to as its depth, and deeper networks are generally able to learn more complex patterns in data. The types of layers used in a network can include convolutional layers for image data, recurrent layers for sequential data, and dense layers for general-purpose learning. Activation functions are used to introduce non-linearity into the network, allowing it to learn more complex relationships in the data.
One of the most common deep learning network architectures is the convolutional neural network (CNN), which is widely used for tasks such as image recognition and object detection. CNNs typically consist of multiple convolutional layers followed by pooling layers to reduce the spatial dimensions of the data. Another popular architecture is the recurrent neural network (RNN), which is designed to handle sequential data such as text or time series data. RNNs use feedback loops to process sequences of data and are commonly used for tasks such as natural language processing and speech recognition.
In recent years, there has been a growing interest in more complex deep learning network architectures such as deep residual networks (ResNets) and transformer networks. ResNets use skip connections to allow information to flow more easily through the network, enabling the training of very deep networks with hundreds of layers. Transformer networks, on the other hand, are designed specifically for processing sequential data and have been highly successful in tasks such as machine translation and language modeling.
Overall, deep learning network architectures are constantly evolving as researchers continue to explore new techniques and approaches to improve the performance of deep learning models. By understanding the principles behind these architectures and how they can be applied to different types of data, researchers and practitioners can develop more effective deep learning models for a wide range of applications.