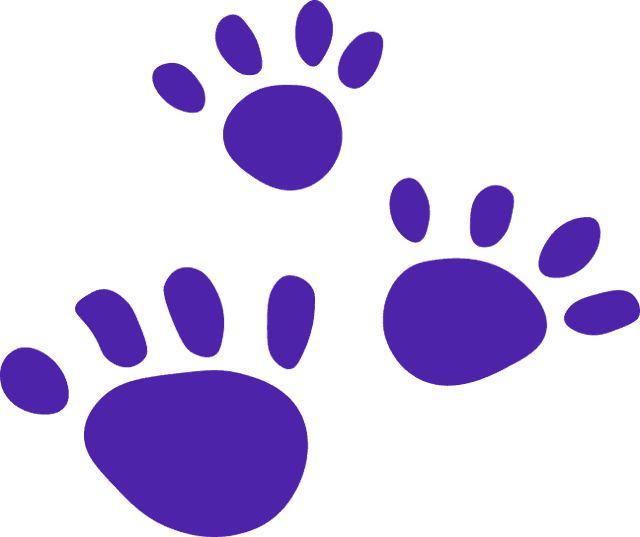
what is collaborative filtering
Collaborative Filtering
Collaborative filtering is a powerful algorithmic technique used in recommendation systems to provide personalized suggestions and recommendations to users based on their interests and preferences. It is a method that leverages the collective intelligence of a group of users to predict and recommend items, products, or content that a particular user might find interesting or relevant.
At its core, collaborative filtering analyzes the behavior and patterns of multiple users to identify similarities and connections between them. By examining their past actions, such as ratings, reviews, purchases, or interactions with certain items, the algorithm can effectively predict the preferences of a user and make recommendations accordingly.
The underlying idea behind collaborative filtering is that users who have similar tastes and preferences in the past are likely to have similar preferences in the future. This concept is often referred to as the "wisdom of the crowd," as it capitalizes on the collective knowledge and experiences of a community to provide accurate and personalized recommendations.
There are two main types of collaborative filtering techniques: user-based and item-based. User-based collaborative filtering compares the preferences of a target user with those of other users to find individuals with similar tastes. It then recommends items that those similar users have liked or purchased but are yet unknown to the target user. On the other hand, item-based collaborative filtering focuses on the similarity between items themselves, recommending items that are similar to those the target user has already shown interest in.
To implement collaborative filtering, a startup house can utilize various data sources, such as user profiles, historical data, user-generated content, and feedback. By collecting and analyzing this data, the algorithm can build a comprehensive understanding of user preferences, enabling accurate and relevant recommendations.
Collaborative filtering has become an integral part of many successful online platforms and startups, including e-commerce websites, streaming services, social media platforms, and content aggregators. By offering personalized recommendations, these platforms enhance user experience, increase engagement, and ultimately drive customer satisfaction and loyalty.
However, it is important to note that collaborative filtering algorithms may face certain challenges. One common issue is the "cold start problem," which occurs when a new user joins the platform or a new item is introduced, and there is limited or no data available to make accurate recommendations. To overcome this, startups can employ hybrid approaches that combine collaborative filtering with other techniques, such as content-based filtering or demographic-based recommendations, to provide initial suggestions.
In conclusion, collaborative filtering is a powerful algorithmic technique that enables startups to provide personalized recommendations and suggestions to their users. By leveraging the collective intelligence of a community, this approach enhances user engagement, improves customer satisfaction, and drives business growth. By implementing collaborative filtering effectively, a startup house can create a dynamic and personalized user experience, gaining a competitive advantage in today's crowded digital landscape.
At its core, collaborative filtering analyzes the behavior and patterns of multiple users to identify similarities and connections between them. By examining their past actions, such as ratings, reviews, purchases, or interactions with certain items, the algorithm can effectively predict the preferences of a user and make recommendations accordingly.
The underlying idea behind collaborative filtering is that users who have similar tastes and preferences in the past are likely to have similar preferences in the future. This concept is often referred to as the "wisdom of the crowd," as it capitalizes on the collective knowledge and experiences of a community to provide accurate and personalized recommendations.
There are two main types of collaborative filtering techniques: user-based and item-based. User-based collaborative filtering compares the preferences of a target user with those of other users to find individuals with similar tastes. It then recommends items that those similar users have liked or purchased but are yet unknown to the target user. On the other hand, item-based collaborative filtering focuses on the similarity between items themselves, recommending items that are similar to those the target user has already shown interest in.
To implement collaborative filtering, a startup house can utilize various data sources, such as user profiles, historical data, user-generated content, and feedback. By collecting and analyzing this data, the algorithm can build a comprehensive understanding of user preferences, enabling accurate and relevant recommendations.
Collaborative filtering has become an integral part of many successful online platforms and startups, including e-commerce websites, streaming services, social media platforms, and content aggregators. By offering personalized recommendations, these platforms enhance user experience, increase engagement, and ultimately drive customer satisfaction and loyalty.
However, it is important to note that collaborative filtering algorithms may face certain challenges. One common issue is the "cold start problem," which occurs when a new user joins the platform or a new item is introduced, and there is limited or no data available to make accurate recommendations. To overcome this, startups can employ hybrid approaches that combine collaborative filtering with other techniques, such as content-based filtering or demographic-based recommendations, to provide initial suggestions.
In conclusion, collaborative filtering is a powerful algorithmic technique that enables startups to provide personalized recommendations and suggestions to their users. By leveraging the collective intelligence of a community, this approach enhances user engagement, improves customer satisfaction, and drives business growth. By implementing collaborative filtering effectively, a startup house can create a dynamic and personalized user experience, gaining a competitive advantage in today's crowded digital landscape.