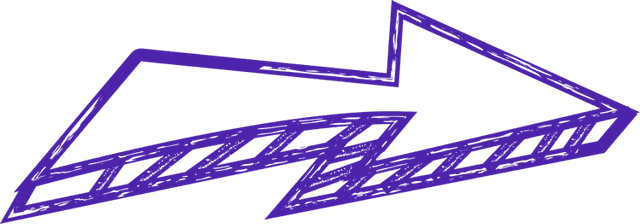
unsupervised learning definition
What is Unsupervised Learning Definition
Unsupervised learning is a type of machine learning algorithm that is used to uncover patterns and relationships within data without the need for labeled outputs. In other words, unsupervised learning allows AI systems to learn from the data itself, rather than being explicitly taught by a human.
One of the key distinctions between unsupervised learning and supervised learning is that in unsupervised learning, the algorithm is not given a specific goal or objective to achieve. Instead, it is left to its own devices to identify patterns and structures within the data. This can be particularly useful in situations where the data is unstructured or where the underlying patterns are not immediately obvious.
There are several different types of unsupervised learning algorithms, each with its own strengths and weaknesses. Clustering algorithms, for example, are used to group similar data points together based on their features, while dimensionality reduction algorithms are used to reduce the complexity of the data by capturing the most important information.
Unsupervised learning has many practical applications in the field of artificial intelligence. For example, it can be used to segment customers based on their purchasing behaviors, to identify anomalies in network traffic that may indicate security threats, or to group similar images together in a photo library.
Overall, unsupervised learning is a powerful tool in the AI toolkit that allows systems to learn from data in a more autonomous and flexible way. By enabling AI systems to uncover hidden patterns and relationships within data, unsupervised learning has the potential to unlock new insights and drive innovation in a wide range of industries.
One of the key distinctions between unsupervised learning and supervised learning is that in unsupervised learning, the algorithm is not given a specific goal or objective to achieve. Instead, it is left to its own devices to identify patterns and structures within the data. This can be particularly useful in situations where the data is unstructured or where the underlying patterns are not immediately obvious.
There are several different types of unsupervised learning algorithms, each with its own strengths and weaknesses. Clustering algorithms, for example, are used to group similar data points together based on their features, while dimensionality reduction algorithms are used to reduce the complexity of the data by capturing the most important information.
Unsupervised learning has many practical applications in the field of artificial intelligence. For example, it can be used to segment customers based on their purchasing behaviors, to identify anomalies in network traffic that may indicate security threats, or to group similar images together in a photo library.
Overall, unsupervised learning is a powerful tool in the AI toolkit that allows systems to learn from data in a more autonomous and flexible way. By enabling AI systems to uncover hidden patterns and relationships within data, unsupervised learning has the potential to unlock new insights and drive innovation in a wide range of industries.