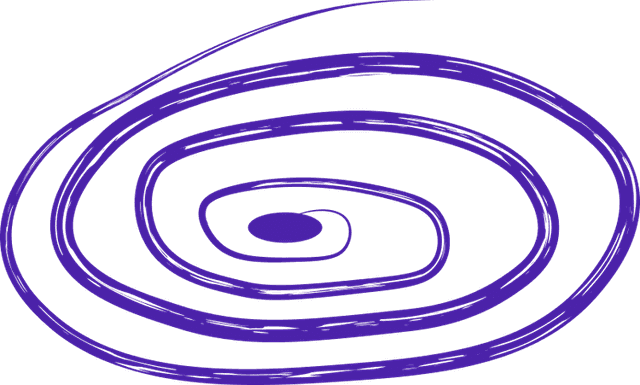
supervised vs unsupervised learning
Supervised vs Unsupervised Learning
Supervised learning and unsupervised learning are two fundamental approaches in the field of machine learning, each with its own unique characteristics and applications.
Supervised learning involves training a model on a labeled dataset, where the input data is paired with corresponding output labels. The goal of supervised learning is to learn a mapping function that can accurately predict the output labels for new, unseen input data. This is achieved by minimizing the error between the predicted output and the true output labels during the training process.
In supervised learning, the model is provided with explicit feedback in the form of labeled data, which serves as a guide for learning the underlying patterns and relationships in the data. This feedback allows the model to adjust its parameters and improve its performance over time. Common examples of supervised learning tasks include classification, regression, and object detection.
On the other hand, unsupervised learning involves training a model on an unlabeled dataset, where the input data is not paired with any output labels. The goal of unsupervised learning is to discover hidden patterns, structures, or relationships within the data without the need for explicit supervision. This is achieved through techniques such as clustering, dimensionality reduction, and anomaly detection.
In unsupervised learning, the model must autonomously identify meaningful patterns in the data without any guidance from labeled examples. This can be a more challenging task, as the model must rely on inherent similarities or differences in the data to uncover useful insights. Unsupervised learning is often used for exploratory data analysis, data preprocessing, and generating new features for downstream tasks.
In summary, supervised learning relies on labeled data to train a model for making predictions, while unsupervised learning leverages unlabeled data to discover patterns or structures within the data. Both approaches have their own strengths and weaknesses, and the choice between supervised and unsupervised learning depends on the specific goals and requirements of the task at hand.
Supervised learning involves training a model on a labeled dataset, where the input data is paired with corresponding output labels. The goal of supervised learning is to learn a mapping function that can accurately predict the output labels for new, unseen input data. This is achieved by minimizing the error between the predicted output and the true output labels during the training process.
In supervised learning, the model is provided with explicit feedback in the form of labeled data, which serves as a guide for learning the underlying patterns and relationships in the data. This feedback allows the model to adjust its parameters and improve its performance over time. Common examples of supervised learning tasks include classification, regression, and object detection.
On the other hand, unsupervised learning involves training a model on an unlabeled dataset, where the input data is not paired with any output labels. The goal of unsupervised learning is to discover hidden patterns, structures, or relationships within the data without the need for explicit supervision. This is achieved through techniques such as clustering, dimensionality reduction, and anomaly detection.
In unsupervised learning, the model must autonomously identify meaningful patterns in the data without any guidance from labeled examples. This can be a more challenging task, as the model must rely on inherent similarities or differences in the data to uncover useful insights. Unsupervised learning is often used for exploratory data analysis, data preprocessing, and generating new features for downstream tasks.
In summary, supervised learning relies on labeled data to train a model for making predictions, while unsupervised learning leverages unlabeled data to discover patterns or structures within the data. Both approaches have their own strengths and weaknesses, and the choice between supervised and unsupervised learning depends on the specific goals and requirements of the task at hand.