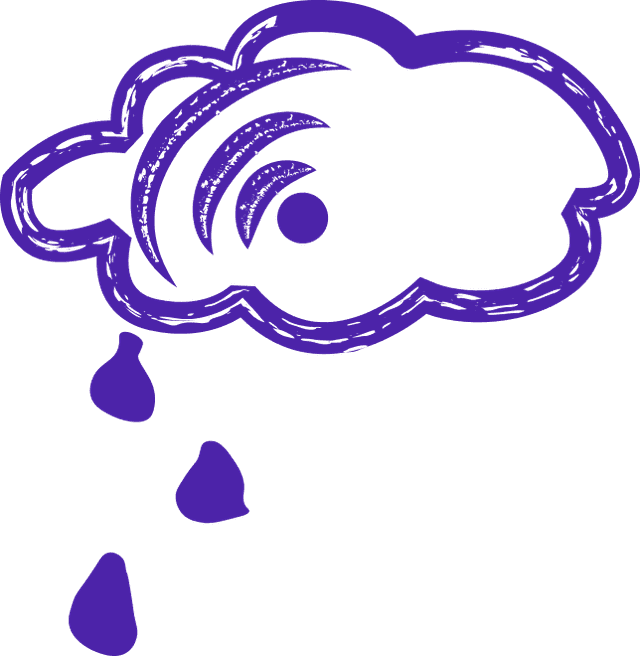
statistical learning theory
Statistical Learning Theory
Statistical learning theory is a branch of machine learning that focuses on understanding the underlying principles of how machines can learn from data. It is concerned with developing algorithms and models that can make predictions or decisions based on patterns and relationships found in data.
At its core, statistical learning theory is based on the idea that there is a relationship between data and the underlying process that generated that data. By analyzing and modeling this relationship, machines can make informed decisions or predictions about new, unseen data.
One of the key concepts in statistical learning theory is the trade-off between bias and variance. Bias refers to the error introduced by simplifying assumptions made by a model, while variance refers to the error introduced by the model's sensitivity to fluctuations in the training data. Finding the right balance between bias and variance is crucial for building models that generalize well to new data.
Another important concept in statistical learning theory is the notion of overfitting and underfitting. Overfitting occurs when a model is too complex and captures noise in the training data, leading to poor performance on new data. Underfitting, on the other hand, occurs when a model is too simple and fails to capture the underlying patterns in the data. Finding the right level of model complexity is essential for building models that can generalize well.
Overall, statistical learning theory provides a framework for understanding how machines can learn from data and make informed decisions. By studying the underlying principles of learning from data, researchers and practitioners can develop more robust and accurate models for a wide range of applications, from image recognition to natural language processing.
At its core, statistical learning theory is based on the idea that there is a relationship between data and the underlying process that generated that data. By analyzing and modeling this relationship, machines can make informed decisions or predictions about new, unseen data.
One of the key concepts in statistical learning theory is the trade-off between bias and variance. Bias refers to the error introduced by simplifying assumptions made by a model, while variance refers to the error introduced by the model's sensitivity to fluctuations in the training data. Finding the right balance between bias and variance is crucial for building models that generalize well to new data.
Another important concept in statistical learning theory is the notion of overfitting and underfitting. Overfitting occurs when a model is too complex and captures noise in the training data, leading to poor performance on new data. Underfitting, on the other hand, occurs when a model is too simple and fails to capture the underlying patterns in the data. Finding the right level of model complexity is essential for building models that can generalize well.
Overall, statistical learning theory provides a framework for understanding how machines can learn from data and make informed decisions. By studying the underlying principles of learning from data, researchers and practitioners can develop more robust and accurate models for a wide range of applications, from image recognition to natural language processing.