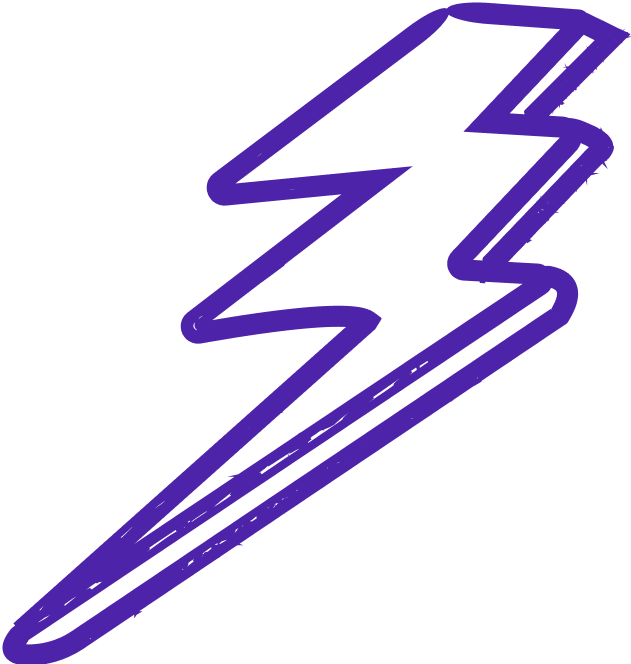
schema matching
Schema Matching: The Art of Harmonizing Diverse Data Landscapes
Schema matching is a process used in data integration to find semantic correspondences between different data schemas. A schema is a blueprint of how data is organized and structured in a database. When data from different sources needs to be integrated, their schemas may not align perfectly, creating a hurdle for seamless data interoperability. That's where schema matching comes in, identifying similarities and equivalences between elements of the schemas, helping to bridge the data gap.
The complexity of schema matching stems from the heterogeneity of data sources. Differences in naming conventions, data types, structures, and levels of granularity can make the matching process a challenging puzzle to solve. But when done right, it enables a unified view of data that would otherwise remain siloed and disconnected.
There are multiple techniques employed in schema matching, including linguistic, structural, constraint-based, and instance-based matching. Each approach has its strengths and weaknesses, and often, a combination of methods is used to achieve the most accurate match.
Schema matching has a broad range of applications. It's crucial in data warehousing, where data from different sources needs to be harmonized. It's vital in the world of web services, where disparate services need to exchange data seamlessly. And it's essential in the realm of E-commerce, where product data from various suppliers needs to be integrated.
In the evolving landscape of big data and artificial intelligence, schema matching is becoming increasingly important. As the volume, variety, and velocity of data continue to grow, so does the need for effective ways to integrate and make sense of this data.
As we wrap up our exploration of schema matching, let's conclude with a light-hearted touch. Here's a tech-inspired limerick to remember our topic by:
In a world where data is catching,
There's a process that's truly fetching.
It matches the schema, in data arena,
Making sense of the data patching.
Indeed, schema matching is the unsung hero in the data world, tirelessly working behind the scenes to connect disparate data dots, enabling us to derive insights from the unified picture they create.
The complexity of schema matching stems from the heterogeneity of data sources. Differences in naming conventions, data types, structures, and levels of granularity can make the matching process a challenging puzzle to solve. But when done right, it enables a unified view of data that would otherwise remain siloed and disconnected.
There are multiple techniques employed in schema matching, including linguistic, structural, constraint-based, and instance-based matching. Each approach has its strengths and weaknesses, and often, a combination of methods is used to achieve the most accurate match.
Schema matching has a broad range of applications. It's crucial in data warehousing, where data from different sources needs to be harmonized. It's vital in the world of web services, where disparate services need to exchange data seamlessly. And it's essential in the realm of E-commerce, where product data from various suppliers needs to be integrated.
In the evolving landscape of big data and artificial intelligence, schema matching is becoming increasingly important. As the volume, variety, and velocity of data continue to grow, so does the need for effective ways to integrate and make sense of this data.
As we wrap up our exploration of schema matching, let's conclude with a light-hearted touch. Here's a tech-inspired limerick to remember our topic by:
In a world where data is catching,
There's a process that's truly fetching.
It matches the schema, in data arena,
Making sense of the data patching.
Indeed, schema matching is the unsung hero in the data world, tirelessly working behind the scenes to connect disparate data dots, enabling us to derive insights from the unified picture they create.