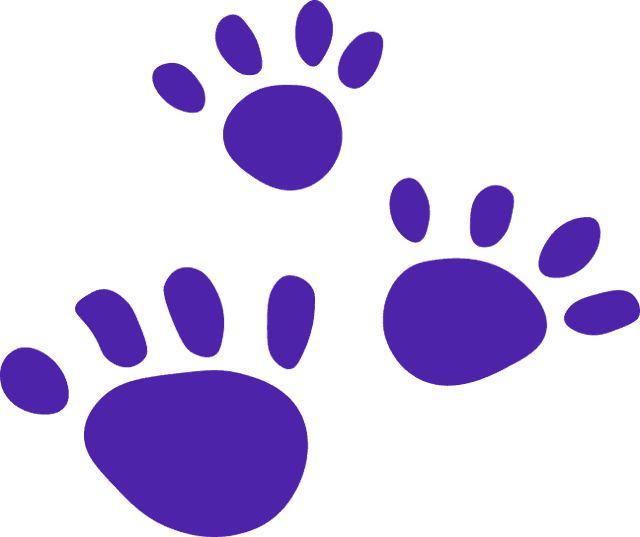
reinforcement learning
What is Reinforcement Learning
Reinforcement learning is a type of machine learning algorithm that is inspired by the way humans and animals learn through positive and negative feedback. In reinforcement learning, an agent learns to make decisions by interacting with an environment and receiving rewards or punishments based on its actions. The goal of the agent is to maximize its cumulative reward over time by learning which actions lead to the best outcomes.
One of the key features of reinforcement learning is the use of a reward signal to guide the learning process. The agent receives a numerical reward signal from the environment after each action it takes, which indicates how good or bad the action was in achieving its goal. Through trial and error, the agent learns to associate certain actions with positive rewards and others with negative rewards, allowing it to gradually improve its decision-making abilities.
Reinforcement learning is particularly well-suited for problems where the optimal decision-making strategy is not known in advance and must be learned through experience. This makes it a powerful tool for training AI systems to perform complex tasks such as playing video games, controlling robots, or making financial decisions.
One of the key challenges in reinforcement learning is the trade-off between exploration and exploitation. The agent must explore different actions to discover which ones lead to the highest rewards, but it also needs to exploit its current knowledge to maximize its cumulative reward. Balancing these two competing objectives is crucial for the agent to learn an effective policy for making decisions.
Recent advances in reinforcement learning, such as deep reinforcement learning, have enabled AI systems to achieve impressive results in a wide range of domains. By combining deep learning techniques with reinforcement learning algorithms, researchers have been able to train AI systems to outperform humans in tasks such as playing complex video games, mastering the game of Go, and controlling autonomous vehicles.
Overall, reinforcement learning represents a powerful approach to training AI systems to make decisions in complex, uncertain environments. By learning from experience and adapting to changing circumstances, these systems can achieve remarkable levels of performance and autonomy, making them valuable tools for solving a wide range of real-world problems.
One of the key features of reinforcement learning is the use of a reward signal to guide the learning process. The agent receives a numerical reward signal from the environment after each action it takes, which indicates how good or bad the action was in achieving its goal. Through trial and error, the agent learns to associate certain actions with positive rewards and others with negative rewards, allowing it to gradually improve its decision-making abilities.
Reinforcement learning is particularly well-suited for problems where the optimal decision-making strategy is not known in advance and must be learned through experience. This makes it a powerful tool for training AI systems to perform complex tasks such as playing video games, controlling robots, or making financial decisions.
One of the key challenges in reinforcement learning is the trade-off between exploration and exploitation. The agent must explore different actions to discover which ones lead to the highest rewards, but it also needs to exploit its current knowledge to maximize its cumulative reward. Balancing these two competing objectives is crucial for the agent to learn an effective policy for making decisions.
Recent advances in reinforcement learning, such as deep reinforcement learning, have enabled AI systems to achieve impressive results in a wide range of domains. By combining deep learning techniques with reinforcement learning algorithms, researchers have been able to train AI systems to outperform humans in tasks such as playing complex video games, mastering the game of Go, and controlling autonomous vehicles.
Overall, reinforcement learning represents a powerful approach to training AI systems to make decisions in complex, uncertain environments. By learning from experience and adapting to changing circumstances, these systems can achieve remarkable levels of performance and autonomy, making them valuable tools for solving a wide range of real-world problems.