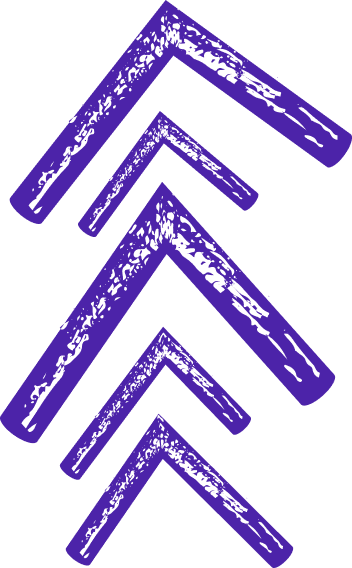
recurrent neural network
What is Recurrent Neural Network
A Recurrent Neural Network (RNN) is a type of artificial neural network designed to handle sequential data and time series information. Unlike traditional feedforward neural networks, which process data in a fixed order and do not have memory of past inputs, RNNs have the ability to retain information about previous inputs through a feedback loop in their architecture. This allows them to effectively model and analyze sequences of data, making them particularly well-suited for tasks such as speech recognition, natural language processing, and time series prediction.
The key feature that sets RNNs apart from other neural network architectures is their ability to maintain a state or memory of previous inputs. This is achieved by connecting the output of a neuron back to its own input, creating a loop that allows information to flow from one time step to the next. As a result, RNNs are able to capture temporal dependencies in data and learn patterns that evolve over time.
One of the main advantages of RNNs is their flexibility in handling input sequences of varying lengths. Unlike traditional feedforward networks, which require fixed-size inputs, RNNs can process sequences of arbitrary length, making them well-suited for tasks where the length of the input data may vary, such as speech recognition or text generation.
However, RNNs are not without their limitations. One of the main challenges with traditional RNNs is the issue of vanishing or exploding gradients, which can make it difficult for the network to learn long-term dependencies in the data. To address this problem, several variants of RNNs have been developed, such as Long Short-Term Memory (LSTM) networks and Gated Recurrent Units (GRUs), which have been shown to be more effective at capturing long-range dependencies in data.
In conclusion, Recurrent Neural Networks are a powerful tool for modeling sequential data and time series information in the field of artificial intelligence. Their ability to retain memory of past inputs and capture temporal dependencies make them well-suited for a wide range of applications, from speech recognition to natural language processing. While they do have limitations, ongoing research and development in the field of RNNs continues to improve their performance and expand their capabilities in AI applications.
The key feature that sets RNNs apart from other neural network architectures is their ability to maintain a state or memory of previous inputs. This is achieved by connecting the output of a neuron back to its own input, creating a loop that allows information to flow from one time step to the next. As a result, RNNs are able to capture temporal dependencies in data and learn patterns that evolve over time.
One of the main advantages of RNNs is their flexibility in handling input sequences of varying lengths. Unlike traditional feedforward networks, which require fixed-size inputs, RNNs can process sequences of arbitrary length, making them well-suited for tasks where the length of the input data may vary, such as speech recognition or text generation.
However, RNNs are not without their limitations. One of the main challenges with traditional RNNs is the issue of vanishing or exploding gradients, which can make it difficult for the network to learn long-term dependencies in the data. To address this problem, several variants of RNNs have been developed, such as Long Short-Term Memory (LSTM) networks and Gated Recurrent Units (GRUs), which have been shown to be more effective at capturing long-range dependencies in data.
In conclusion, Recurrent Neural Networks are a powerful tool for modeling sequential data and time series information in the field of artificial intelligence. Their ability to retain memory of past inputs and capture temporal dependencies make them well-suited for a wide range of applications, from speech recognition to natural language processing. While they do have limitations, ongoing research and development in the field of RNNs continues to improve their performance and expand their capabilities in AI applications.