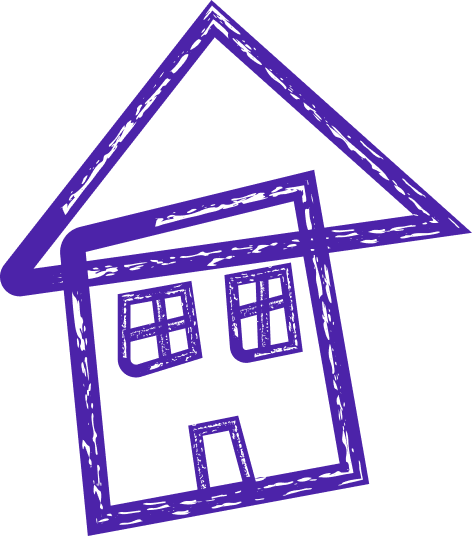
recommender systems
Recommender Systems
Recommender systems are algorithms designed to predict and suggest items or content that a user may be interested in based on their past behaviors, preferences, and interactions with the system. These systems are widely used in various online platforms such as e-commerce websites, streaming services, social media platforms, and more to enhance user experience and engagement.
Recommender systems utilize various techniques such as collaborative filtering, content-based filtering, and hybrid approaches to generate personalized recommendations for users. Collaborative filtering analyzes user behavior and preferences to identify patterns and similarities between users to recommend items that similar users have liked in the past. Content-based filtering, on the other hand, focuses on the attributes of items and user preferences to recommend items that are similar to those the user has interacted with before. Hybrid approaches combine both collaborative and content-based filtering techniques to provide more accurate and diverse recommendations.
Recommender systems play a crucial role in improving user satisfaction, increasing user engagement, and driving sales and revenue for businesses. By providing personalized recommendations, these systems help users discover new items, products, or content that they may not have found on their own, leading to higher user retention and loyalty. Additionally, recommender systems can help businesses optimize their marketing strategies, increase customer engagement, and drive conversions by delivering targeted and relevant recommendations to users.
Overall, recommender systems have become an essential tool for businesses looking to enhance user experience, increase customer satisfaction, and drive growth in today's highly competitive digital landscape. By leveraging data and algorithms to deliver personalized recommendations, these systems help users discover new and relevant items, products, or content, ultimately creating a more engaging and enjoyable user experience.
Recommender systems utilize various techniques such as collaborative filtering, content-based filtering, and hybrid approaches to generate personalized recommendations for users. Collaborative filtering analyzes user behavior and preferences to identify patterns and similarities between users to recommend items that similar users have liked in the past. Content-based filtering, on the other hand, focuses on the attributes of items and user preferences to recommend items that are similar to those the user has interacted with before. Hybrid approaches combine both collaborative and content-based filtering techniques to provide more accurate and diverse recommendations.
Recommender systems play a crucial role in improving user satisfaction, increasing user engagement, and driving sales and revenue for businesses. By providing personalized recommendations, these systems help users discover new items, products, or content that they may not have found on their own, leading to higher user retention and loyalty. Additionally, recommender systems can help businesses optimize their marketing strategies, increase customer engagement, and drive conversions by delivering targeted and relevant recommendations to users.
Overall, recommender systems have become an essential tool for businesses looking to enhance user experience, increase customer satisfaction, and drive growth in today's highly competitive digital landscape. By leveraging data and algorithms to deliver personalized recommendations, these systems help users discover new and relevant items, products, or content, ultimately creating a more engaging and enjoyable user experience.