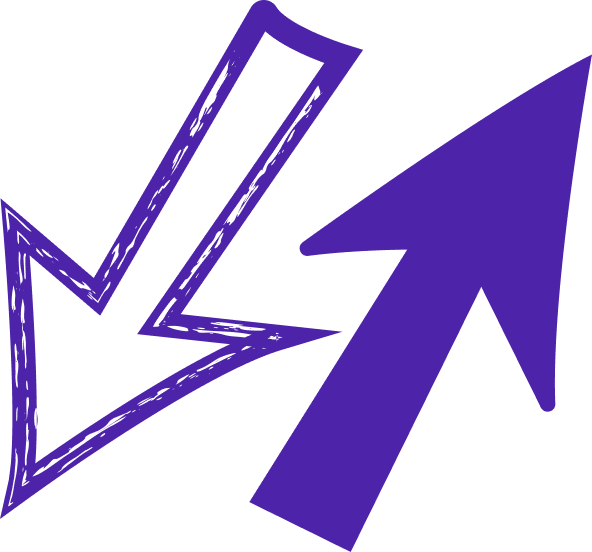
one shot learning
What is One Shot Learning
One Shot Learning is a concept in the field of artificial intelligence that refers to the ability of a machine learning model to learn from just a single example of a new class or category. Traditional machine learning algorithms often require a large amount of labeled data to train on in order to accurately classify new instances. However, One Shot Learning aims to mimic the way humans learn, by being able to generalize from just one or a few examples.
This type of learning is particularly useful in situations where collecting a large amount of labeled data is not feasible or practical, such as in medical imaging or rare event detection. By being able to learn from just a single example, One Shot Learning can significantly reduce the time and resources required to train a machine learning model.
One Shot Learning is typically achieved through the use of techniques such as siamese networks, which are neural networks that are designed to learn similarities between pairs of inputs. By comparing a new input to a single example of a class, the model can determine whether the new input belongs to that class or not.
One of the key challenges in One Shot Learning is designing a model that can effectively generalize from just one example, without overfitting to the training data. This requires careful design of the network architecture, as well as the use of techniques such as data augmentation and regularization to prevent the model from memorizing the training examples.
Overall, One Shot Learning is an exciting area of research in artificial intelligence that has the potential to revolutionize the way machine learning models are trained and deployed. By enabling machines to learn from just a single example, we can unlock new possibilities for applications in fields such as healthcare, finance, and security.
This type of learning is particularly useful in situations where collecting a large amount of labeled data is not feasible or practical, such as in medical imaging or rare event detection. By being able to learn from just a single example, One Shot Learning can significantly reduce the time and resources required to train a machine learning model.
One Shot Learning is typically achieved through the use of techniques such as siamese networks, which are neural networks that are designed to learn similarities between pairs of inputs. By comparing a new input to a single example of a class, the model can determine whether the new input belongs to that class or not.
One of the key challenges in One Shot Learning is designing a model that can effectively generalize from just one example, without overfitting to the training data. This requires careful design of the network architecture, as well as the use of techniques such as data augmentation and regularization to prevent the model from memorizing the training examples.
Overall, One Shot Learning is an exciting area of research in artificial intelligence that has the potential to revolutionize the way machine learning models are trained and deployed. By enabling machines to learn from just a single example, we can unlock new possibilities for applications in fields such as healthcare, finance, and security.