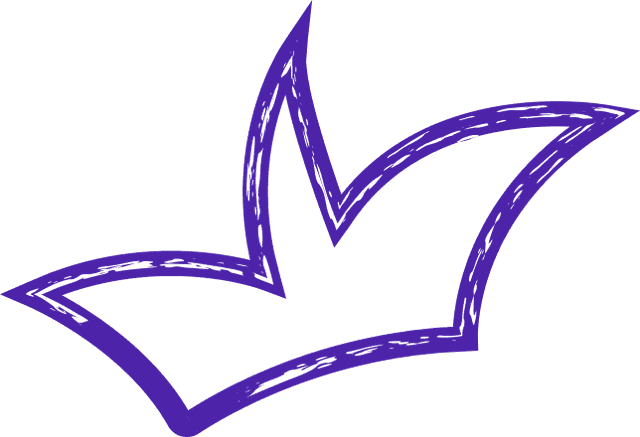
neural architecture search
What is Neural Architecture Search
Neural Architecture Search (NAS) is a cutting-edge technique in the field of artificial intelligence that aims to automate the design of neural networks. Neural networks are a fundamental component of deep learning, a subset of machine learning that has revolutionized the field of AI in recent years. These networks are composed of layers of interconnected nodes, or neurons, that process and learn from data to make predictions or decisions.
Traditionally, the design of neural networks has been a time-consuming and labor-intensive process, requiring expert knowledge and extensive trial and error. However, with the advent of NAS, researchers are now able to leverage the power of algorithms and computational resources to automatically search for optimal network architectures.
The goal of NAS is to find neural network architectures that are not only highly accurate and performant, but also efficient in terms of computational resources and memory usage. By automating the design process, NAS can potentially lead to the development of more powerful and efficient AI systems that can tackle complex tasks such as image recognition, natural language processing, and autonomous driving.
There are several approaches to NAS, including reinforcement learning, evolutionary algorithms, and gradient-based optimization. These methods involve searching through a vast space of possible network architectures, evaluating their performance on a given task, and iteratively refining the search process to find the best architecture.
One of the key challenges in NAS is the trade-off between exploration and exploitation. On one hand, researchers want to explore a wide range of network architectures to find novel and innovative solutions. On the other hand, they also want to exploit known architectures that have proven to be effective in the past. Balancing these competing objectives is crucial for the success of NAS.
Overall, Neural Architecture Search represents a promising direction in the field of AI research, with the potential to revolutionize the way neural networks are designed and deployed. By automating the design process, NAS can accelerate the development of AI systems and enable researchers to focus on higher-level tasks such as problem formulation and data analysis. As the field continues to evolve, we can expect to see even more sophisticated and efficient NAS algorithms that push the boundaries of what is possible in artificial intelligence.
Traditionally, the design of neural networks has been a time-consuming and labor-intensive process, requiring expert knowledge and extensive trial and error. However, with the advent of NAS, researchers are now able to leverage the power of algorithms and computational resources to automatically search for optimal network architectures.
The goal of NAS is to find neural network architectures that are not only highly accurate and performant, but also efficient in terms of computational resources and memory usage. By automating the design process, NAS can potentially lead to the development of more powerful and efficient AI systems that can tackle complex tasks such as image recognition, natural language processing, and autonomous driving.
There are several approaches to NAS, including reinforcement learning, evolutionary algorithms, and gradient-based optimization. These methods involve searching through a vast space of possible network architectures, evaluating their performance on a given task, and iteratively refining the search process to find the best architecture.
One of the key challenges in NAS is the trade-off between exploration and exploitation. On one hand, researchers want to explore a wide range of network architectures to find novel and innovative solutions. On the other hand, they also want to exploit known architectures that have proven to be effective in the past. Balancing these competing objectives is crucial for the success of NAS.
Overall, Neural Architecture Search represents a promising direction in the field of AI research, with the potential to revolutionize the way neural networks are designed and deployed. By automating the design process, NAS can accelerate the development of AI systems and enable researchers to focus on higher-level tasks such as problem formulation and data analysis. As the field continues to evolve, we can expect to see even more sophisticated and efficient NAS algorithms that push the boundaries of what is possible in artificial intelligence.