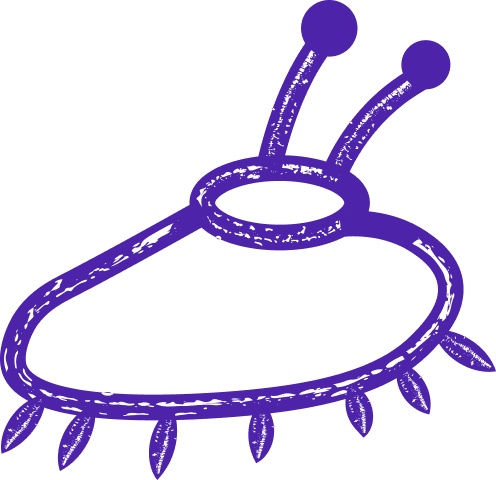
natural language inference
What is Natural Language Inference
Natural Language Inference (NLI) is a crucial task in the field of artificial intelligence that involves determining the logical relationship between two pieces of text. In NLI, the goal is to determine whether a given hypothesis can be inferred from a given premise, based on the meaning and context of the text.
NLI is a challenging problem for AI systems because it requires a deep understanding of language and the ability to reason about the relationships between different pieces of text. This task is important for a wide range of applications, including question answering, information retrieval, and dialogue systems.
In recent years, there has been a growing interest in developing AI models that can perform NLI tasks with high accuracy. One of the key challenges in NLI is the lack of large-scale annotated datasets, which are necessary for training and evaluating AI models. However, recent advancements in natural language processing and machine learning techniques have led to the development of powerful models that can effectively tackle NLI tasks.
One of the most popular approaches to NLI is the use of deep learning models, such as neural networks, which can learn to represent the meaning of text in a high-dimensional space. These models can then be used to compare the meaning of two pieces of text and determine whether one can be inferred from the other.
Another important aspect of NLI is the ability to handle ambiguity and uncertainty in language. Human language is inherently ambiguous, and different people may interpret the same text in different ways. AI models that can effectively handle ambiguity and uncertainty are essential for accurate NLI tasks.
Overall, Natural Language Inference is a complex and challenging task in the field of artificial intelligence, but recent advancements in AI technologies have made significant progress in this area. By developing more robust and accurate NLI models, we can improve the performance of AI systems in a wide range of applications, ultimately leading to more intelligent and capable AI systems.
NLI is a challenging problem for AI systems because it requires a deep understanding of language and the ability to reason about the relationships between different pieces of text. This task is important for a wide range of applications, including question answering, information retrieval, and dialogue systems.
In recent years, there has been a growing interest in developing AI models that can perform NLI tasks with high accuracy. One of the key challenges in NLI is the lack of large-scale annotated datasets, which are necessary for training and evaluating AI models. However, recent advancements in natural language processing and machine learning techniques have led to the development of powerful models that can effectively tackle NLI tasks.
One of the most popular approaches to NLI is the use of deep learning models, such as neural networks, which can learn to represent the meaning of text in a high-dimensional space. These models can then be used to compare the meaning of two pieces of text and determine whether one can be inferred from the other.
Another important aspect of NLI is the ability to handle ambiguity and uncertainty in language. Human language is inherently ambiguous, and different people may interpret the same text in different ways. AI models that can effectively handle ambiguity and uncertainty are essential for accurate NLI tasks.
Overall, Natural Language Inference is a complex and challenging task in the field of artificial intelligence, but recent advancements in AI technologies have made significant progress in this area. By developing more robust and accurate NLI models, we can improve the performance of AI systems in a wide range of applications, ultimately leading to more intelligent and capable AI systems.