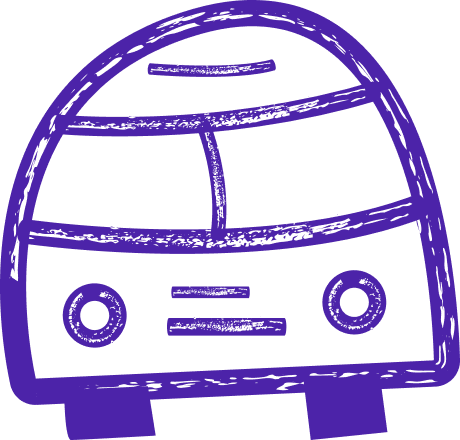
mlops devops difference
Mlops Devops Difference
MLOps and DevOps are two terms that are often used interchangeably in the world of software development and operations. However, there are some key differences between the two that are important to understand. In this post, we will explore the differences between MLOps and DevOps, and how they each play a crucial role in the success of a modern software development team.
First, let's start with DevOps. DevOps is a set of practices that combines software development (Dev) and IT operations (Ops) in order to shorten the systems development life cycle and provide continuous delivery of high-quality software. DevOps focuses on collaboration, automation, and integration between developers and IT operations teams to improve the speed and quality of software delivery.
On the other hand, MLOps is a set of practices that combines machine learning (ML) and IT operations (Ops) in order to streamline the development and deployment of machine learning models. MLOps focuses on managing the entire machine learning lifecycle, from data preparation and model training to deployment and monitoring in production. MLOps helps data scientists and machine learning engineers work more efficiently and effectively by providing a standardized and automated way to manage machine learning workflows.
One of the key differences between MLOps and DevOps is the focus on machine learning in MLOps. While DevOps is more focused on traditional software development, MLOps is specifically designed to address the unique challenges of developing and deploying machine learning models. This includes managing large volumes of data, training complex models, and monitoring model performance in production.
Another key difference between MLOps and DevOps is the tools and technologies that are commonly used in each practice. DevOps tools such as Jenkins, Docker, and Kubernetes are often used to automate and streamline the software development process. In contrast, MLOps tools such as MLflow, Kubeflow, and TensorFlow Serving are specifically designed to support the development and deployment of machine learning models.
In conclusion, while MLOps and DevOps share some similarities in terms of their goals of improving collaboration and automation between development and operations teams, they are distinct practices with their own unique focuses and challenges. Understanding the differences between MLOps and DevOps is crucial for organizations looking to successfully implement both practices and maximize the value of their machine learning and software development efforts.
First, let's start with DevOps. DevOps is a set of practices that combines software development (Dev) and IT operations (Ops) in order to shorten the systems development life cycle and provide continuous delivery of high-quality software. DevOps focuses on collaboration, automation, and integration between developers and IT operations teams to improve the speed and quality of software delivery.
On the other hand, MLOps is a set of practices that combines machine learning (ML) and IT operations (Ops) in order to streamline the development and deployment of machine learning models. MLOps focuses on managing the entire machine learning lifecycle, from data preparation and model training to deployment and monitoring in production. MLOps helps data scientists and machine learning engineers work more efficiently and effectively by providing a standardized and automated way to manage machine learning workflows.
One of the key differences between MLOps and DevOps is the focus on machine learning in MLOps. While DevOps is more focused on traditional software development, MLOps is specifically designed to address the unique challenges of developing and deploying machine learning models. This includes managing large volumes of data, training complex models, and monitoring model performance in production.
Another key difference between MLOps and DevOps is the tools and technologies that are commonly used in each practice. DevOps tools such as Jenkins, Docker, and Kubernetes are often used to automate and streamline the software development process. In contrast, MLOps tools such as MLflow, Kubeflow, and TensorFlow Serving are specifically designed to support the development and deployment of machine learning models.
In conclusion, while MLOps and DevOps share some similarities in terms of their goals of improving collaboration and automation between development and operations teams, they are distinct practices with their own unique focuses and challenges. Understanding the differences between MLOps and DevOps is crucial for organizations looking to successfully implement both practices and maximize the value of their machine learning and software development efforts.