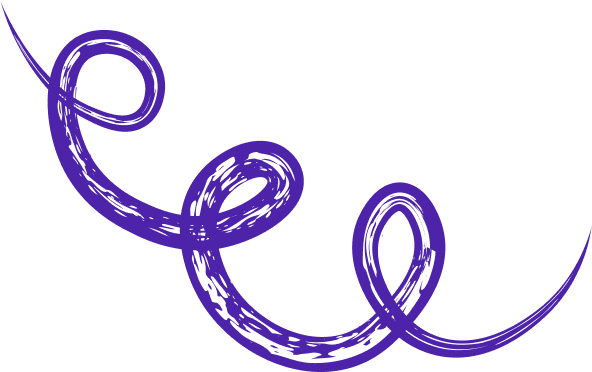
measurement scale
Measurement Scale: The Scaffolding of Quantitative Data in Research
Starting the discourse, a measurement scale in research is a structured method for collecting, organizing, and interpreting data. Think of it as the measuring tape in a researcher's toolkit, helping them make sense of the data they collect.
Measurement scales provide a systematic approach to assigning numbers or labels to data, allowing researchers to quantify and compare their findings. They come in four main types: nominal, ordinal, interval, and ratio, each providing different levels of information and lending themselves to different statistical analyses.
The nominal scale is the most basic, categorizing data without any order or hierarchy. For example, it could be used to categorize survey respondents by their favorite color. The ordinal scale, on the other hand, introduces an order, like classifying runners in a race by their finishing position.
The interval scale takes it a step further, offering not only order but also a consistent scale between values. Temperature, for instance, is usually measured on an interval scale. The ratio scale adds an absolute zero point, providing a complete quantitative analysis. This is commonly used for measurements like weight or height.
Measurement scales are crucial to the validity and interpretability of research data. They guide the data collection process, influence the choice of statistical analysis, and determine the level of insights that can be derived from the data.
However, choosing the right measurement scale requires careful consideration of the research objectives, the nature of the data, and the intended level of analysis. After all, using the wrong scale can lead to incorrect conclusions and undermine the research findings.
To close off, think of measurement scales as the lenses through which we view and interpret research data. They help us bring the data into focus, offering insights and clarity. But remember, just as you wouldn't use a microscope to view a landscape, it's crucial to choose the right measurement scale for your research. After all, a researcher without a suitable scale is like a tailor without a tape measure - you might end up with conclusions that just don't fit!
Measurement scales provide a systematic approach to assigning numbers or labels to data, allowing researchers to quantify and compare their findings. They come in four main types: nominal, ordinal, interval, and ratio, each providing different levels of information and lending themselves to different statistical analyses.
The nominal scale is the most basic, categorizing data without any order or hierarchy. For example, it could be used to categorize survey respondents by their favorite color. The ordinal scale, on the other hand, introduces an order, like classifying runners in a race by their finishing position.
The interval scale takes it a step further, offering not only order but also a consistent scale between values. Temperature, for instance, is usually measured on an interval scale. The ratio scale adds an absolute zero point, providing a complete quantitative analysis. This is commonly used for measurements like weight or height.
Measurement scales are crucial to the validity and interpretability of research data. They guide the data collection process, influence the choice of statistical analysis, and determine the level of insights that can be derived from the data.
However, choosing the right measurement scale requires careful consideration of the research objectives, the nature of the data, and the intended level of analysis. After all, using the wrong scale can lead to incorrect conclusions and undermine the research findings.
To close off, think of measurement scales as the lenses through which we view and interpret research data. They help us bring the data into focus, offering insights and clarity. But remember, just as you wouldn't use a microscope to view a landscape, it's crucial to choose the right measurement scale for your research. After all, a researcher without a suitable scale is like a tailor without a tape measure - you might end up with conclusions that just don't fit!