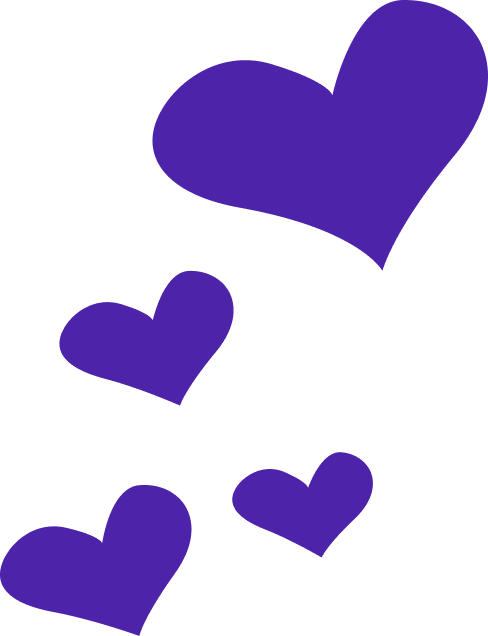
fine tuning in ai
What is Fine Tuning In Ai
Fine tuning in AI refers to the process of taking a pre-trained neural network model and further training it on a specific task or dataset to improve its performance on that particular task. This technique is commonly used in the field of machine learning to adapt a model that has been trained on a large and diverse dataset to a more specialized task.
Fine tuning is particularly useful when working with limited amounts of data or when the target task is significantly different from the original task the model was trained on. By fine tuning a pre-trained model, researchers and developers can leverage the knowledge and representations learned by the model during its initial training and apply it to a new, related task.
The process of fine tuning typically involves freezing the weights of the initial layers of the model, which are responsible for learning general features, and only updating the weights of the later layers, which are more task-specific. This allows the model to retain the knowledge it has learned from the original dataset while adapting to the nuances of the new task.
Fine tuning can significantly improve the performance of a model on a specific task, as it allows the model to leverage the large amounts of data and computational resources used during its initial training. Additionally, fine tuning can reduce the need for extensive training on a new dataset, saving time and resources.
Overall, fine tuning is a powerful technique in the field of AI that allows researchers and developers to quickly adapt pre-trained models to new tasks and datasets, improving performance and efficiency in a wide range of applications. By understanding and utilizing the principles of fine tuning, AI practitioners can continue to push the boundaries of what is possible with machine learning and artificial intelligence.
Fine tuning is particularly useful when working with limited amounts of data or when the target task is significantly different from the original task the model was trained on. By fine tuning a pre-trained model, researchers and developers can leverage the knowledge and representations learned by the model during its initial training and apply it to a new, related task.
The process of fine tuning typically involves freezing the weights of the initial layers of the model, which are responsible for learning general features, and only updating the weights of the later layers, which are more task-specific. This allows the model to retain the knowledge it has learned from the original dataset while adapting to the nuances of the new task.
Fine tuning can significantly improve the performance of a model on a specific task, as it allows the model to leverage the large amounts of data and computational resources used during its initial training. Additionally, fine tuning can reduce the need for extensive training on a new dataset, saving time and resources.
Overall, fine tuning is a powerful technique in the field of AI that allows researchers and developers to quickly adapt pre-trained models to new tasks and datasets, improving performance and efficiency in a wide range of applications. By understanding and utilizing the principles of fine tuning, AI practitioners can continue to push the boundaries of what is possible with machine learning and artificial intelligence.