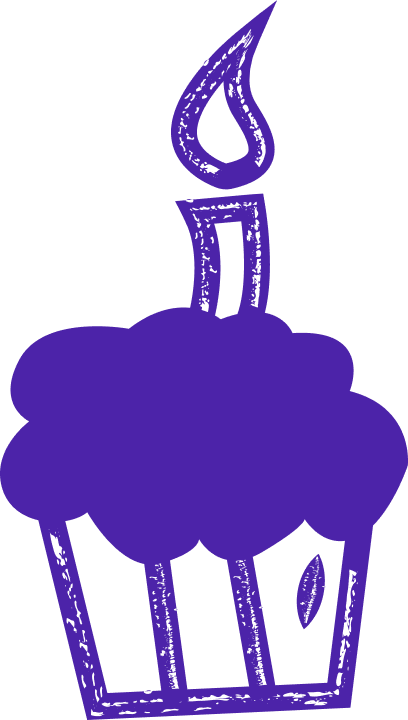
feature extraction
Feature extraction
What is Feature Extraction
In the field of machine learning and data analysis, feature extraction plays a crucial role in extracting relevant information from raw data. As a leading software development company, Startup House understands the importance of feature extraction and its impact on building high-performing algorithms and models.
Feature extraction can be defined as the process of selecting and transforming raw data into a set of meaningful features that can be used to represent the underlying patterns and characteristics of the data. These features act as input variables for machine learning algorithms, enabling them to learn and make predictions based on the extracted information.
At Startup House, we employ various techniques and methodologies to perform feature extraction, depending on the nature of the data and the problem at hand. These techniques can range from simple statistical methods to more advanced algorithms such as principal component analysis (PCA) and independent component analysis (ICA).
One of the key principles of feature extraction is to reduce the dimensionality of the data while preserving its relevant information. This is important because high-dimensional data can lead to computational inefficiencies and overfitting, where the model becomes too specific to the training data and fails to generalize well to new data.
By extracting the most informative features, we can effectively reduce the dimensionality of the data, making it easier to analyze and model. This not only improves the efficiency and performance of machine learning algorithms but also enhances the interpretability of the results.
Feature extraction involves a series of steps, including data preprocessing, feature selection, and feature transformation. In the data preprocessing stage, we clean and preprocess the raw data to remove any noise or inconsistencies that may affect the quality of the extracted features.
Next, in the feature selection stage, we identify the most relevant features that have a strong correlation with the target variable or possess discriminative power. This helps in reducing the dimensionality of the data and eliminating irrelevant or redundant features that do not contribute significantly to the model's performance.
Finally, in the feature transformation stage, we transform the selected features into a new representation that captures the underlying patterns and structures of the data. This can be achieved through various mathematical and statistical techniques, such as scaling, normalization, or dimensionality reduction algorithms.
At Startup House, we understand that feature extraction is a critical step in building robust and accurate machine learning models. Our team of experienced data scientists and engineers are well-versed in the latest feature extraction techniques and methodologies, ensuring that our clients receive the most relevant and informative features for their specific business needs.
In conclusion, feature extraction is an essential process in machine learning and data analysis that involves selecting and transforming raw data into meaningful features. Through careful preprocessing, selection, and transformation, feature extraction enables us to reduce the dimensionality of the data while preserving its relevant information. At Startup House, we leverage our expertise in feature extraction to build high-performing algorithms and models that drive business success.