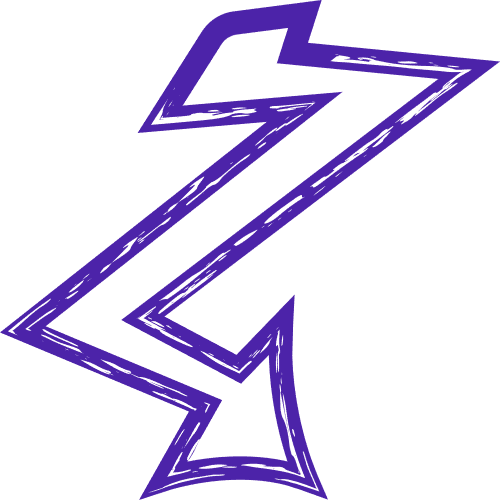
discriminative ai
What is Discriminative Ai
Discriminative AI, also known as discriminative modeling, is a type of artificial intelligence that focuses on learning the relationship between input data and output labels. Unlike generative AI, which aims to model the entire distribution of the data, discriminative AI is more focused on making predictions based on the input data.
One of the key characteristics of discriminative AI is its ability to learn complex patterns and relationships in the data without explicitly modeling the underlying data distribution. This makes discriminative AI particularly useful in tasks such as classification, regression, and anomaly detection, where the goal is to predict a specific label or value based on the input data.
Discriminative AI algorithms typically rely on supervised learning, where the model is trained on a labeled dataset to learn the relationship between the input data and the output labels. This training process involves adjusting the model's parameters to minimize the error between the predicted output and the true label.
One of the main advantages of discriminative AI is its ability to handle high-dimensional data and complex relationships between variables. This makes it well-suited for tasks such as image recognition, natural language processing, and speech recognition, where the input data can be highly complex and varied.
However, discriminative AI also has its limitations. For example, it may struggle with tasks where the underlying data distribution is not well understood or where the relationships between variables are not easily discernible. In such cases, generative AI may be more appropriate as it can model the entire data distribution and generate new data samples.
Overall, discriminative AI plays a crucial role in the field of artificial intelligence by enabling machines to learn complex patterns and relationships in the data and make accurate predictions. By understanding the principles and applications of discriminative AI, researchers and practitioners can develop more effective and efficient AI systems for a wide range of tasks and applications.
One of the key characteristics of discriminative AI is its ability to learn complex patterns and relationships in the data without explicitly modeling the underlying data distribution. This makes discriminative AI particularly useful in tasks such as classification, regression, and anomaly detection, where the goal is to predict a specific label or value based on the input data.
Discriminative AI algorithms typically rely on supervised learning, where the model is trained on a labeled dataset to learn the relationship between the input data and the output labels. This training process involves adjusting the model's parameters to minimize the error between the predicted output and the true label.
One of the main advantages of discriminative AI is its ability to handle high-dimensional data and complex relationships between variables. This makes it well-suited for tasks such as image recognition, natural language processing, and speech recognition, where the input data can be highly complex and varied.
However, discriminative AI also has its limitations. For example, it may struggle with tasks where the underlying data distribution is not well understood or where the relationships between variables are not easily discernible. In such cases, generative AI may be more appropriate as it can model the entire data distribution and generate new data samples.
Overall, discriminative AI plays a crucial role in the field of artificial intelligence by enabling machines to learn complex patterns and relationships in the data and make accurate predictions. By understanding the principles and applications of discriminative AI, researchers and practitioners can develop more effective and efficient AI systems for a wide range of tasks and applications.