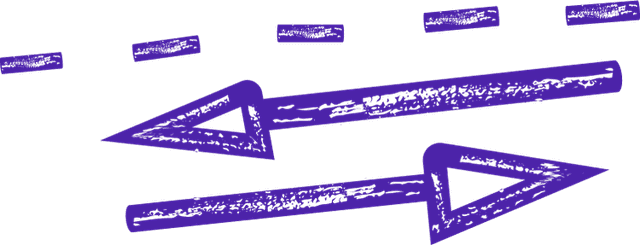
deep belief networks
What is Deep Belief Networks
Deep Belief Networks (DBNs) are a type of artificial neural network that has gained popularity in the field of artificial intelligence (AI) due to their ability to learn complex patterns and representations from large amounts of data. DBNs are composed of multiple layers of nodes, with each layer representing a different level of abstraction in the data.
One of the key features of DBNs is their hierarchical structure, which allows them to learn hierarchical representations of the input data. This means that the lower layers of the network learn simple features, such as edges and textures, while the higher layers learn more complex features, such as objects and scenes. This hierarchical representation makes DBNs well-suited for tasks such as image and speech recognition, where the input data is highly structured and contains multiple levels of abstraction.
Another important aspect of DBNs is their ability to perform unsupervised learning, which means that they can learn from unlabeled data without the need for explicit labels or annotations. This is achieved through a process called pre-training, where each layer of the network is trained independently using a technique called restricted Boltzmann machines (RBMs). Once the network has been pre-trained, it can be fine-tuned using supervised learning to improve its performance on specific tasks.
DBNs have been successfully applied to a wide range of AI tasks, including image and speech recognition, natural language processing, and recommendation systems. They have also been used in areas such as healthcare, finance, and robotics, where they have shown promise in improving the accuracy and efficiency of various applications.
In conclusion, Deep Belief Networks are a powerful tool in the field of artificial intelligence, allowing researchers and developers to build sophisticated models that can learn complex patterns and representations from large amounts of data. By leveraging their hierarchical structure and unsupervised learning capabilities, DBNs have the potential to revolutionize the way we approach AI tasks and unlock new possibilities for intelligent systems.
One of the key features of DBNs is their hierarchical structure, which allows them to learn hierarchical representations of the input data. This means that the lower layers of the network learn simple features, such as edges and textures, while the higher layers learn more complex features, such as objects and scenes. This hierarchical representation makes DBNs well-suited for tasks such as image and speech recognition, where the input data is highly structured and contains multiple levels of abstraction.
Another important aspect of DBNs is their ability to perform unsupervised learning, which means that they can learn from unlabeled data without the need for explicit labels or annotations. This is achieved through a process called pre-training, where each layer of the network is trained independently using a technique called restricted Boltzmann machines (RBMs). Once the network has been pre-trained, it can be fine-tuned using supervised learning to improve its performance on specific tasks.
DBNs have been successfully applied to a wide range of AI tasks, including image and speech recognition, natural language processing, and recommendation systems. They have also been used in areas such as healthcare, finance, and robotics, where they have shown promise in improving the accuracy and efficiency of various applications.
In conclusion, Deep Belief Networks are a powerful tool in the field of artificial intelligence, allowing researchers and developers to build sophisticated models that can learn complex patterns and representations from large amounts of data. By leveraging their hierarchical structure and unsupervised learning capabilities, DBNs have the potential to revolutionize the way we approach AI tasks and unlock new possibilities for intelligent systems.