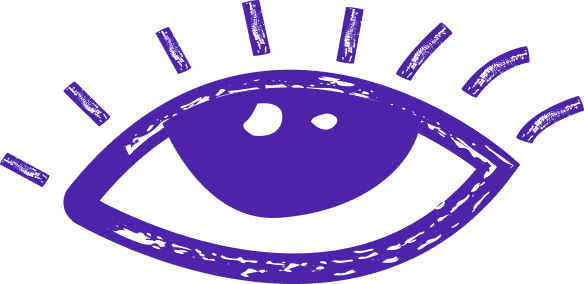
data sampling
What is Data Sampling
Data sampling is a crucial concept in the field of artificial intelligence (AI) that involves selecting a subset of data from a larger dataset to represent the whole. This process is essential for training machine learning models and making predictions based on data.
In AI, data sampling is used to ensure that the model is trained on a diverse and representative sample of the data. By selecting a subset of the data, researchers can reduce the computational burden of processing large datasets while still capturing the essential patterns and relationships within the data.
There are several methods of data sampling that are commonly used in AI, including random sampling, stratified sampling, and cluster sampling. Random sampling involves selecting data points at random from the dataset, while stratified sampling involves dividing the dataset into subgroups and sampling from each subgroup proportionally. Cluster sampling involves dividing the dataset into clusters and sampling entire clusters rather than individual data points.
Data sampling is important in AI because it helps to prevent overfitting, which occurs when a model learns the noise in the data rather than the underlying patterns. By selecting a representative sample of the data, researchers can ensure that the model generalizes well to unseen data and makes accurate predictions.
In conclusion, data sampling is a critical concept in the field of artificial intelligence that helps to ensure that machine learning models are trained on representative data. By selecting a subset of the data, researchers can reduce the computational burden of processing large datasets while still capturing the essential patterns and relationships within the data. Data sampling is essential for preventing overfitting and ensuring that AI models make accurate predictions.
In AI, data sampling is used to ensure that the model is trained on a diverse and representative sample of the data. By selecting a subset of the data, researchers can reduce the computational burden of processing large datasets while still capturing the essential patterns and relationships within the data.
There are several methods of data sampling that are commonly used in AI, including random sampling, stratified sampling, and cluster sampling. Random sampling involves selecting data points at random from the dataset, while stratified sampling involves dividing the dataset into subgroups and sampling from each subgroup proportionally. Cluster sampling involves dividing the dataset into clusters and sampling entire clusters rather than individual data points.
Data sampling is important in AI because it helps to prevent overfitting, which occurs when a model learns the noise in the data rather than the underlying patterns. By selecting a representative sample of the data, researchers can ensure that the model generalizes well to unseen data and makes accurate predictions.
In conclusion, data sampling is a critical concept in the field of artificial intelligence that helps to ensure that machine learning models are trained on representative data. By selecting a subset of the data, researchers can reduce the computational burden of processing large datasets while still capturing the essential patterns and relationships within the data. Data sampling is essential for preventing overfitting and ensuring that AI models make accurate predictions.