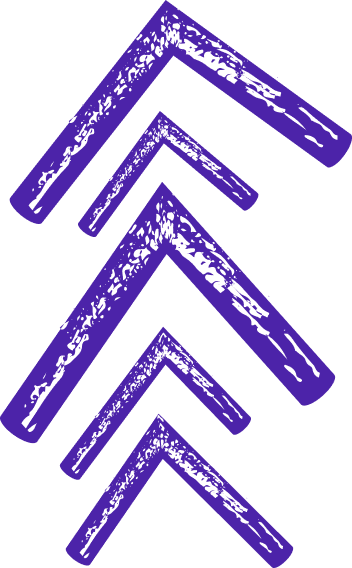
causeeffect graph
Cause-Effect Graphs: Mapping Intricacies in Data Relationships
Within the bustling metropolis of data analysis, cause-effect graphs stand as a vital tool to illuminate the intertwined relationships that exist beneath the surface of raw data.
A cause-effect graph, often referred to as a cause-effect diagram or a Fishbone diagram, is a visual representation that maps out the potential causes and effects within a system. It provides a structured way to analyze complex processes, helping to identify, sort, and display possible causes of a specific problem or quality characteristic.
The use of cause-effect graphs spans numerous fields, including but not limited to, quality control, business process improvement, and software testing. By creating a visual map of cause and effect relationships, these graphs simplify the understanding of complex systems, promoting better decision-making and problem-solving.
In the realm of software testing, cause-effect graphing is a technique used to identify and explore the different conditions and their corresponding outcomes. It aids in generating test cases, ensuring thorough coverage of all the different ways a software system could behave.
The beauty of a cause-effect graph lies in its simplicity. It breaks down complex systems into digestible parts, making it easier to identify the root cause of a problem. The 'effect' is usually a problem or issue that needs addressing, while the 'causes' are the factors leading to this problem.
Despite their simplicity, cause-effect graphs can become quite complex when dealing with intricate systems with numerous interconnected factors. However, they remain a powerful tool for distilling complexity into understandable insights, supporting informed decision-making.
Cause-effect graphs are a testament to the power of visual information. They transform abstract concepts and invisible relationships into tangible, understandable visual models. By making the unseen seen, they open up new perspectives and understanding, driving more effective solutions.
And now, for a playful ending: why was the data analyst at the aquarium? He was trying to understand the cause-effect graph of the "school" of fish. After all, in data analysis, every little detail "counts"! Here's to making sense of the complex, one graph at a time.
A cause-effect graph, often referred to as a cause-effect diagram or a Fishbone diagram, is a visual representation that maps out the potential causes and effects within a system. It provides a structured way to analyze complex processes, helping to identify, sort, and display possible causes of a specific problem or quality characteristic.
The use of cause-effect graphs spans numerous fields, including but not limited to, quality control, business process improvement, and software testing. By creating a visual map of cause and effect relationships, these graphs simplify the understanding of complex systems, promoting better decision-making and problem-solving.
In the realm of software testing, cause-effect graphing is a technique used to identify and explore the different conditions and their corresponding outcomes. It aids in generating test cases, ensuring thorough coverage of all the different ways a software system could behave.
The beauty of a cause-effect graph lies in its simplicity. It breaks down complex systems into digestible parts, making it easier to identify the root cause of a problem. The 'effect' is usually a problem or issue that needs addressing, while the 'causes' are the factors leading to this problem.
Despite their simplicity, cause-effect graphs can become quite complex when dealing with intricate systems with numerous interconnected factors. However, they remain a powerful tool for distilling complexity into understandable insights, supporting informed decision-making.
Cause-effect graphs are a testament to the power of visual information. They transform abstract concepts and invisible relationships into tangible, understandable visual models. By making the unseen seen, they open up new perspectives and understanding, driving more effective solutions.
And now, for a playful ending: why was the data analyst at the aquarium? He was trying to understand the cause-effect graph of the "school" of fish. After all, in data analysis, every little detail "counts"! Here's to making sense of the complex, one graph at a time.