🌍 All
About us
Digitalization
News
Startups
Development
Design
Supervised Machine Learning: A Comprehensive Guide
Alexander Stasiak
Jun 04, 2024・7 min read
Table of Content
Key Features of Supervised Machine Learning
How Supervised Machine Learning Works
Applications of Supervised Machine Learning
Benefits of Supervised Learning
Challenges of Supervised Learning
FAQs
Supervised machine learning is one of the foundational techniques in artificial intelligence, widely used for tasks like fraud detection, risk assessment, and image classification. This method involves training models on labeled data to make accurate predictions on unseen data. Let’s delve into how supervised learning works and why it’s pivotal in modern machine learning applications.
What Is Supervised Machine Learning?
Supervised machine learning involves using input data (features) and corresponding output data (labels) to train a model. The model learns the relationship between inputs and outputs from labeled datasets, enabling it to predict the output for new, unseen inputs.
For instance, in a spam email classifier, the training data consists of emails labeled as "spam" or "not spam." The model learns patterns in the data and applies them to classify new emails.
Key Features of Supervised Machine Learning
- Labeled Training Data
Supervised learning relies on labeled datasets, where each data point has a corresponding output label. - Two Main Tasks
- Classification: Predicts discrete categories, like spam detection or image classification.
- Regression: Predicts continuous values, like house prices or stock market trends.
- Common Algorithms
- Linear Regression
- Logistic Regression
- Support Vector Machines (SVMs)
- Decision Trees
- Random Forests
- Neural Networks
- Model Training and Testing
Models are trained on a labeled dataset (training data) and evaluated on a separate dataset (test data) to assess performance. - Iterative Learning
Models improve through iterative processes, refining their predictions based on errors in training.
How Supervised Machine Learning Works
- Data Collection and Labeling
Gather and prepare labeled data relevant to the problem. - Data Splitting
Divide the dataset into training and test subsets, often in an 80:20 ratio. - Model Selection
Choose a supervised learning algorithm suited to the problem type (classification or regression). - Model Training
Train the model using the labeled training data, adjusting parameters to minimize errors. - Model Testing
Test the model on unseen data to evaluate its accuracy and generalization capabilities. - Optimization
Fine-tune the model by adjusting hyperparameters, selecting features, or using additional data.
Applications of Supervised Machine Learning
- Fraud Detection
Supervised learning models analyze transaction data to identify fraudulent patterns. - Image Classification
Used in facial recognition, medical imaging, and object detection tasks. - Natural Language Processing (NLP)
Powers applications like sentiment analysis, chatbots, and spam email detection. - Predictive Analytics
Predicts customer behavior, stock prices, or disease progression using regression models. - Anomaly Detection
Identifies unusual data points in financial transactions or system logs.
Benefits of Supervised Learning
- Accuracy: Delivers highly accurate predictions with labeled data.
- Wide Applicability: Supports diverse tasks across industries like finance, healthcare, and e-commerce.
- Scalability: Algorithms can handle large datasets effectively.
Challenges of Supervised Learning
- Data Dependency: Requires large, labeled datasets, which can be time-consuming to collect.
- Overfitting: Models may perform well on training data but fail to generalize to unseen data.
- Bias in Data: Poorly labeled or biased data can skew predictions and reduce model reliability.
FAQs
What is supervised machine learning?
Supervised machine learning uses labeled data to train models for predicting outputs based on inputs.
How does supervised learning differ from unsupervised learning?
Supervised learning uses labeled data, while unsupervised learning works with unlabeled data to find patterns.
What are the main types of supervised learning tasks?
The two main tasks are classification (predicting categories) and regression (predicting continuous values).
What are examples of supervised learning algorithms?
Popular algorithms include linear regression, logistic regression, decision trees, random forests, and neural networks.
Why is labeled data important in supervised learning?
Labeled data helps the model learn the relationship between input features and desired outputs.
What is the role of training and test data in supervised learning?
Training data teaches the model, while test data evaluates its accuracy on unseen inputs.
What industries use supervised learning?
Industries like healthcare, finance, retail, and technology use supervised learning for predictive analytics, risk assessment, and more.
What is overfitting in supervised learning?
Overfitting occurs when a model performs well on training data but poorly on new, unseen data.
How can supervised learning handle large datasets?
Scalable algorithms and distributed computing frameworks enable supervised learning to process large datasets efficiently.
What are common challenges in supervised learning?
Challenges include collecting labeled data, avoiding overfitting, and addressing biases in datasets.
What is the difference between linear and logistic regression?
Linear regression predicts continuous values, while logistic regression predicts binary outcomes.
Can supervised learning work with unlabeled data?
No, supervised learning requires labeled data, but semi-supervised learning can use a mix of labeled and unlabeled data.
What is the role of neural networks in supervised learning?
Neural networks are powerful supervised learning models used for complex tasks like image and speech recognition.
How does a model improve during training?
The model iteratively adjusts parameters to minimize prediction errors using algorithms like gradient descent.
What are the evaluation metrics for supervised learning models?
Metrics include accuracy, precision, recall, F1-score for classification, and RMSE or MAE for regression.
What is the target variable in supervised learning?
The target variable is the labeled output that the model predicts based on input features.
How does supervised learning handle unbalanced datasets?
Techniques like oversampling, undersampling, or using weighted algorithms address unbalanced datasets.
What tools are commonly used for supervised learning?
Popular tools include Python libraries like scikit-learn, TensorFlow, and PyTorch.
Can supervised learning models predict multiple outputs?
Yes, multi-output regression and classification models can predict multiple outputs simultaneously.
How does supervised learning contribute to AI?
Supervised learning forms the foundation for many AI applications, enabling accurate predictions and decision-making.
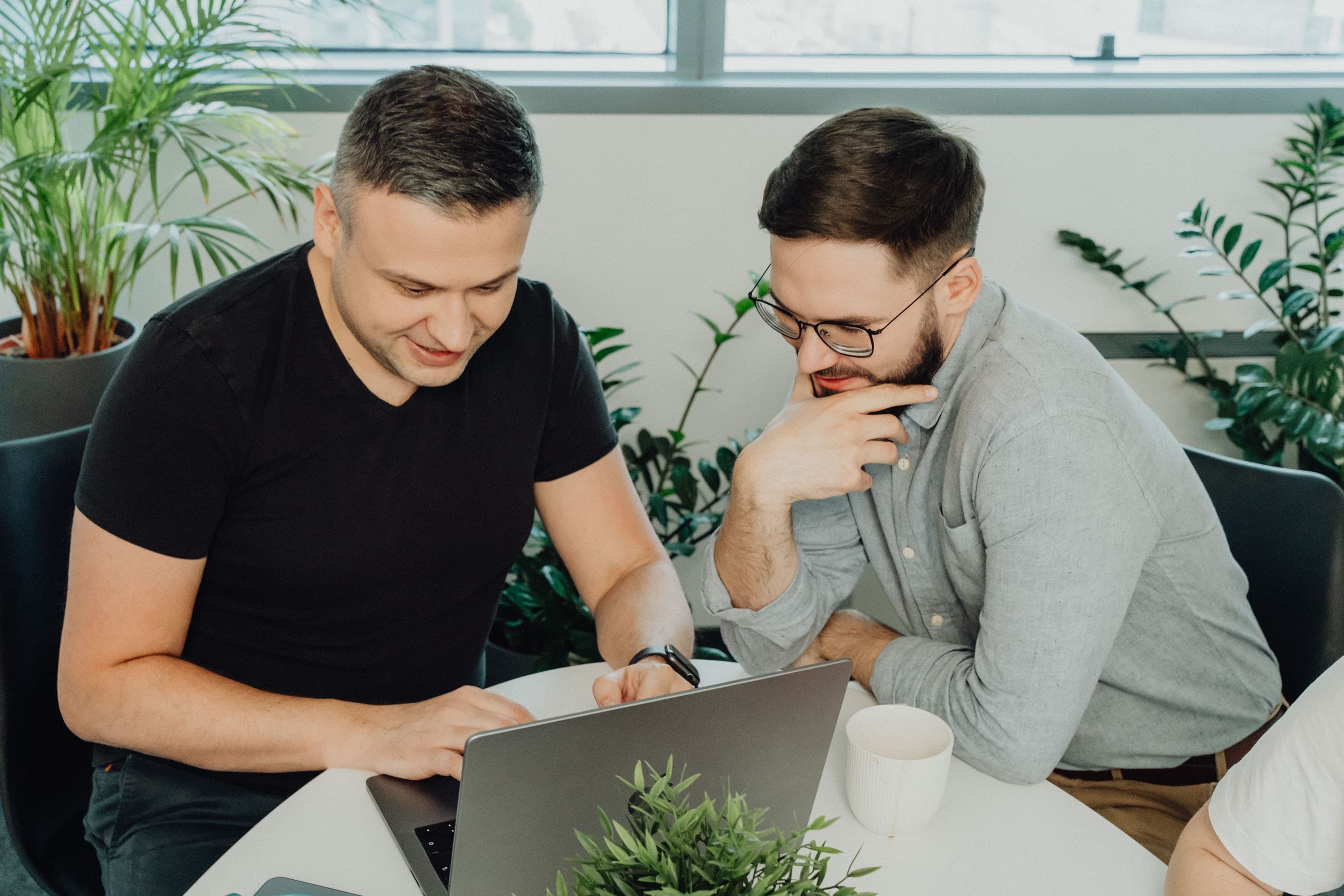
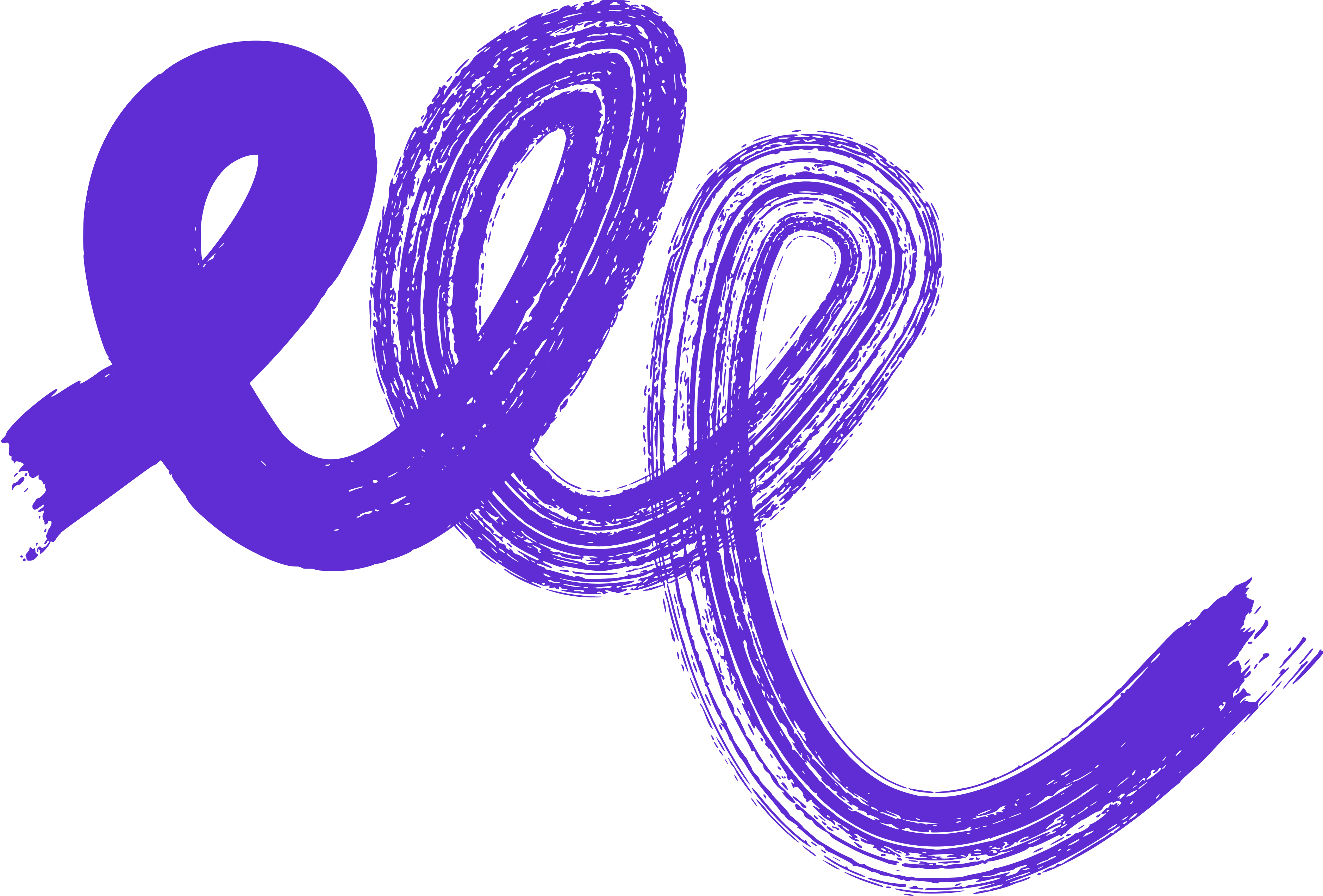
You may also
like...
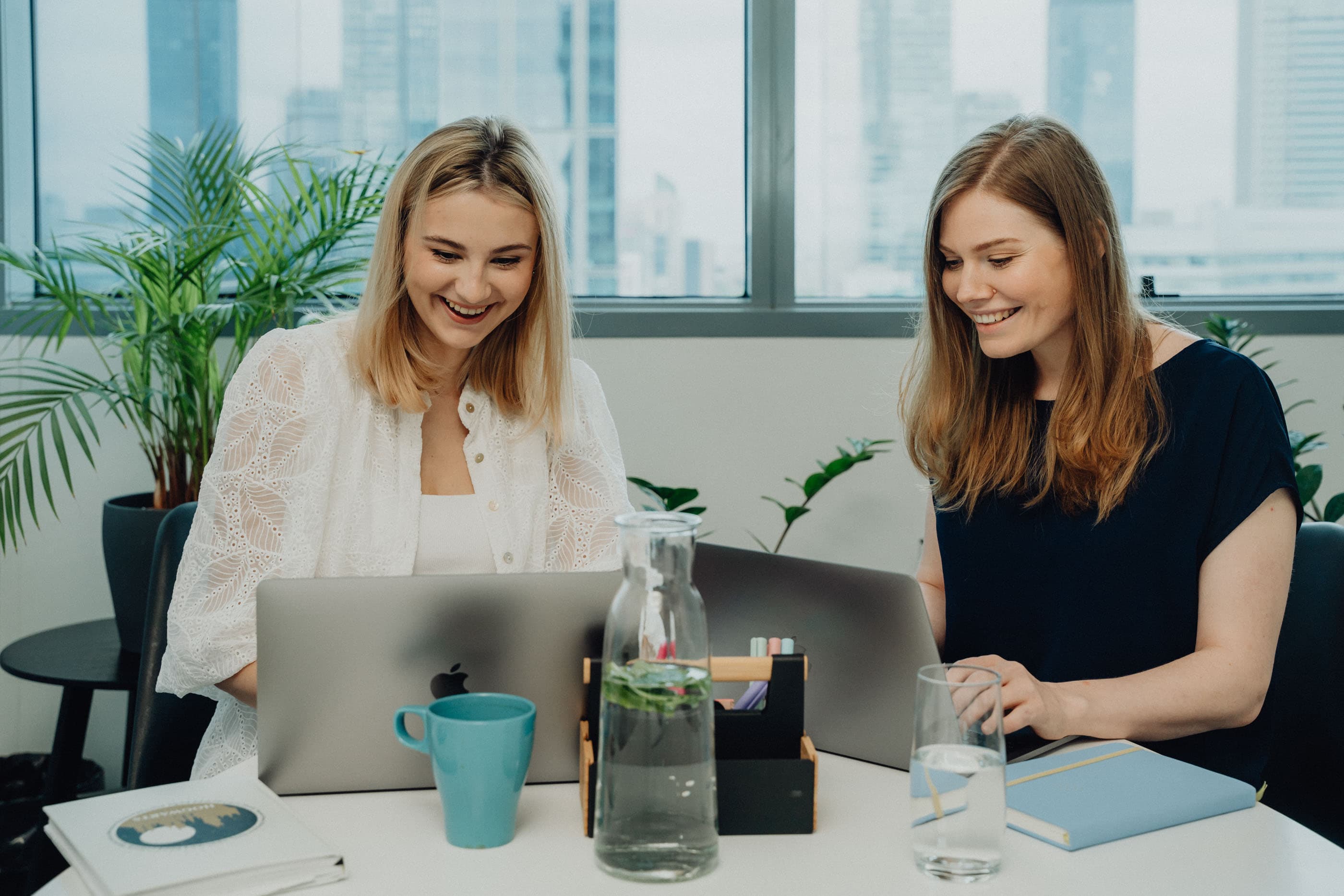
Mastering Declarative Programming: Essential Practices for Every Developer
Discover declarative programming essentials. This guide covers principles, tools, and best practices to simplify coding, enhance readability, and improve scalability.
Marek Pałys
Apr 16, 2024・11 min read
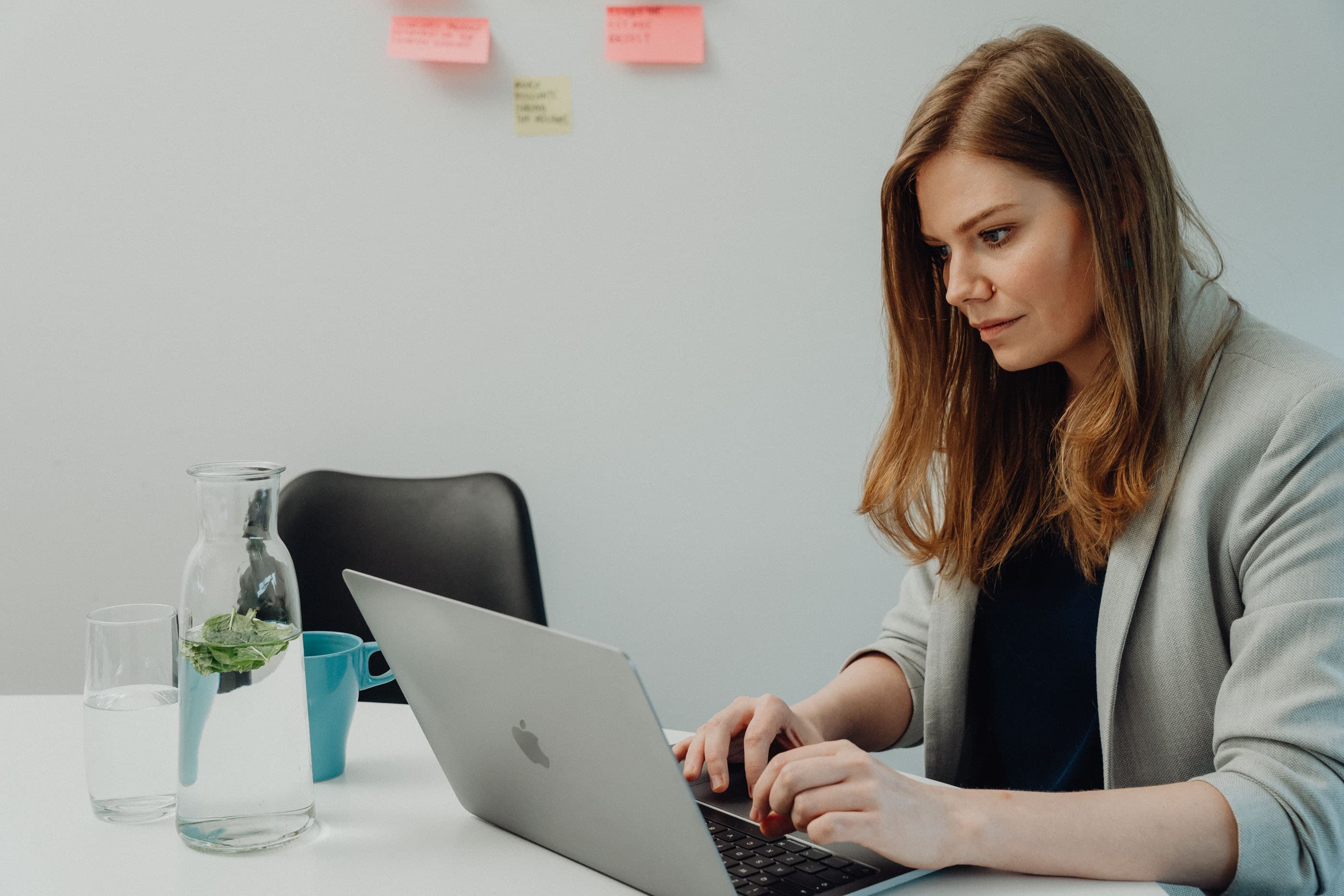
Understanding Event-Driven Programming: A Simple Guide for Everyone
Explore the essentials of event-driven programming. Learn how this responsive paradigm powers interactive applications with real-world examples and key concepts.
Marek Pałys
Apr 30, 2024・9 min read
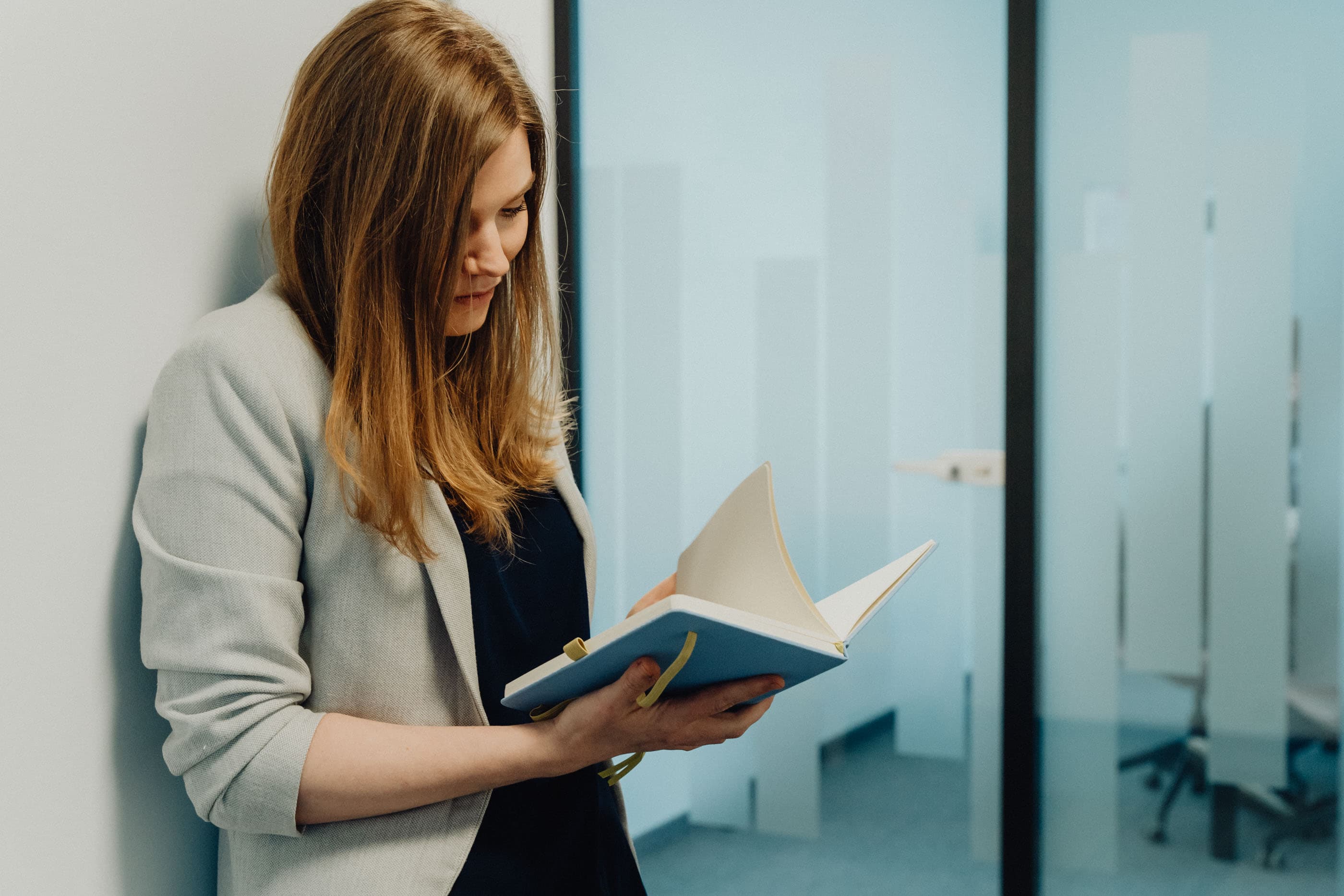
Demystifying Procedural Programming: Simple Examples for All
Explore procedural programming with easy-to-follow examples and insights into its core principles. Learn how this step-by-step approach forms the basis of many programming paradigms.
Marek Pałys
Jul 05, 2024・10 min read