🌍 All
About us
Digitalization
News
Startups
Development
Design
Transforming Finance: The Impact of Predictive Analytics Software on Financial Forecasting
Marek Pałys
Oct 31, 2024・12 min read
Table of Content
Introduction to Predictive Analytics in Finance
How Predictive Analytics Software is Shaping Financial Forecasting
Key Features of Predictive Analytics Software
Benefits for Financial Institutions
Future Trends in Predictive Analytics
Predictive analytics software is proving to be a game-changer in the realm of financial forecasting. By harnessing the power of advanced algorithms and data analysis, these tools offer unprecedented accuracy and efficiency, allowing businesses to anticipate market trends and make informed decisions with confidence. How Predictive Analytics Software is Shaping Financial Forecasting is a topic of growing importance as organisations seek to optimise their strategies and stay ahead in a competitive market. This document will delve into the transformative impact of predictive analytics on financial forecasting, exploring how these innovations are redefining the way companies approach financial planning, cash flow forecasting and risk management. Join us as we unpack the practical benefits and challenges associated with integrating predictive analytics into financial operations.
Introduction to Predictive Analytics in Finance
Defining Predictive Analytics
Predictive analytics involves using historical data, statistical algorithms, and machine learning techniques to identify the likelihood of future outcomes. Unlike traditional analytics, which focuses on understanding past events, predictive analytics aims to forecast future events. This approach allows businesses to not only react to changes but also to anticipate them. By analysing patterns and trends within large datasets, companies can make more informed decisions, reduce risks, and discover new opportunities. In the context of finance, predictive analytics software is utilised to see future financial trends, forecast market trends, assess credit risk, and optimise investment strategies. This ability to predict future scenarios with a high degree of accuracy is what sets predictive analytics apart and makes it a valuable tool in financial forecasting.
Importance in Financial Forecasting
Predictive analytics holds significant importance in financial forecasting processes due to its ability to refine predictions and increase accuracy. In a sector where even minor errors can lead to substantial financial loss, having reliable forecasts is crucial. Predictive analytics enables organisations to utilise comprehensive data analysis to gain insights into potential future financial conditions. This capability allows businesses to plan more effectively, allocate resources wisely, and mitigate risks associated with market volatility. Moreover, by understanding potential trends and consumer behaviour, companies can develop strategies that are more aligned with future market demands. This proactive approach not only enhances decision-making but also helps maintain a competitive edge. In essence, predictive analytics transforms financial forecasting from a reactive process into a strategic tool, empowering businesses to navigate uncertainties with greater confidence.
How Predictive Analytics Software is Shaping Financial Forecasting
Enhancing Accuracy in Predictions
Predictive analytics software significantly enhances the accuracy of financial predictions by leveraging vast amounts of data and advanced algorithms. Traditional methods often rely on limited datasets and static models, which can lead to imprecise forecasts. In contrast, predictive analytics employs dynamic models that adapt to new data, providing more precise and timely insights. This adaptability is crucial in the fast-paced financial environment, where conditions can change rapidly. By incorporating various machine learning algorithms, these tools can identify complex patterns and relationships within the data that may not be immediately apparent to human analysts. Consequently, businesses can make decisions based on a more comprehensive understanding of potential future scenarios. This improved accuracy not only helps in forecasting revenues and expenses but also aids in strategic planning and risk management, ensuring that organisations are better prepared for future challenges.
Streamlining Financial Processes
Predictive analytics software streamlines financial processes by automating routine tasks and reducing the need for manual intervention. Through the use of sophisticated algorithms, these tools can handle large volumes of data more efficiently than traditional methods. This efficiency not only saves time but also reduces the likelihood of human error. By automating data collection, analysis, and reporting, predictive analytics software allows financial professionals to focus on higher-level strategic decision-making. Additionally, the software provides real-time insights and updates historical financial data, enabling quicker adjustments to financial strategies as conditions evolve. The integration of predictive analytics into financial workflows can lead to more agile and responsive operations, as businesses can swiftly adapt to market changes. This streamlining of processes ultimately contributes to improved productivity and cost savings, making predictive analytics an invaluable asset in financial management.
Reducing Financial Risks
Predictive analytics software plays a crucial role in reducing financial risks by providing insights into potential future events and their impacts. By analysing historical data and identifying patterns, these tools can forecast risks associated with market fluctuations, credit defaults, and operational inefficiencies. This foresight allows businesses to develop risk mitigation strategies proactively, rather than reacting to issues as they arise. Additionally, predictive analytics can assess the probability of adverse financial outcomes, enabling companies to allocate resources more effectively and safeguard their assets. This proactive risk management approach helps in maintaining financial stability and protecting against significant losses. Moreover, by continuously updating predictions with new data, predictive analytics ensures that organisations remain informed about evolving risks, allowing for timely interventions. As a result of predictive modeling, businesses can navigate uncertainties with greater assurance, reducing the overall risk exposure and enhancing financial resilience.
Key Features of Predictive Analytics Software
Data Integration Capabilities
One of the standout features of predictive analytics software is its robust data integration capabilities. These tools can seamlessly consolidate data from diverse sources, such as financial databases, market reports, and customer records, into a unified platform. This integration ensures that all relevant information is considered in the analysis, leading to more comprehensive and accurate predictions. By breaking down data silos, predictive analytics software provides a holistic view of financial activities and market dynamics. Additionally, the software can handle both structured and unstructured data, allowing for a more flexible and inclusive approach to data analysis. This ability to integrate various data types from multiple sources enhances the depth and breadth of insights derived from the predictive analytics models. Consequently, businesses can make well-informed decisions based on a complete picture of their financial landscape, fostering more strategic and effective financial planning and management.
Advanced Algorithms and Models
Predictive analytics software employs advanced algorithms and models to generate accurate and actionable insights. These sophisticated algorithms, including both machine learning models and artificial intelligence, are designed to analyse large datasets, identify patterns, and predict future outcomes with high precision. The models used can adapt and learn from new data, enhancing their predictive capabilities over time. This adaptability ensures that the software remains relevant and accurate even as market conditions change. Furthermore, these advanced models can handle complex relationships within the data that traditional methods might overlook. By leveraging these cutting-edge technologies, businesses can uncover deeper insights and make more informed decisions. The use of advanced algorithms and models thus represents a significant leap forward in financial forecasting, enabling companies to stay ahead of market trends and optimise their financial strategies with greater confidence.
Real-time Data Processing
Real-time data processing is a pivotal feature of predictive analytics software, enabling businesses to receive up-to-the-minute insights and make timely decisions. This capability allows the software to analyse data as it is generated, providing instant updates on financial trends and market conditions. By processing data in real time, companies can quickly adjust their strategies in response to emerging opportunities or risks. This immediacy is particularly beneficial in volatile markets, where delays in decision-making could lead to significant financial setbacks. Furthermore, real-time processing ensures that the most current data is used in analyses, enhancing the accuracy and relevance of predictions. This feature empowers organisations to remain agile and responsive, maintaining a competitive edge by capitalising on timely insights. Thus, real-time data processing is an essential component shaping the future of predictive analytics software, supporting dynamic and proactive financial management.
Benefits for Financial Institutions
Improved Decision-Making
Predictive analytics software enhances decision-making processes within financial institutions by providing data-driven insights that inform strategic choices. By analysing vast amounts of historical and current data, this software identifies trends and patterns that may not be immediately apparent. This comprehensive understanding of the financial landscape enables institutions to make more informed and confident decisions. Moreover, predictive analytics facilitates scenario planning, allowing institutions to evaluate potential outcomes of various strategies before implementation. This foresight helps in minimising risks and maximising returns. The data analytics software's ability to deliver precise predictions also supports more effective resource allocation and risk management, ensuring decisions are not only timely but also strategically sound. Ultimately, predictive analytics empowers financial institutions to optimise their operations, adapt to market changes swiftly, and maintain a competitive advantage by making well-informed, evidence-based decisions.
Increased Operational Efficiency
Predictive analytics software significantly boosts operational efficiency in financial institutions by automating routine tasks and streamlining complex processes. With the ability to process large volumes of data quickly and accurately, these tools reduce the time and effort required for data analysis, freeing up valuable resources for more strategic activities. Automation of repetitive tasks minimises human error, ensuring that operations run smoothly and efficiently. Additionally, predictive analytics tools can identify process bottlenecks and inefficiencies, providing actionable insights for process optimisation. By enabling institutions to predict and manage demand more effectively, resources can be allocated where they are most needed, reducing waste and improving service delivery. This increased efficiency not only enhances productivity but also contributes to cost savings, as institutions can operate with greater precision and agility. In turn, improved operational efficiency leads to better customer satisfaction and a stronger competitive position in the financial market.
Better Customer Insights
Predictive analytics software equips financial institutions with deeper customer insights by analysing behavioural patterns and preferences. This enhanced understanding allows institutions to tailor their services and products to meet specific customer needs more effectively. By examining historical transaction data and current trends in financial indicators, predictive analytics can forecast customer behaviours, such as purchasing habits and potential churn risks. This foresight enables institutions to develop personalised marketing strategies, improving customer engagement and loyalty. Furthermore, predictive analytics can identify opportunities for cross-selling and up-selling, increasing revenue potential. By leveraging these insights, financial institutions can create more targeted and relevant offerings, enhancing the overall customer experience. Additionally, understanding customer preferences and needs allows for more proactive and responsive customer service, further strengthening customer relationships. Ultimately, better customer insights lead to improved customer satisfaction and retention, which are critical for long-term success in the competitive financial sector.
Future Trends in Predictive Analytics
Emerging Technologies and Innovations
The field of predictive analytics is set to evolve significantly with the advent of emerging technologies and innovations. Artificial intelligence (AI) and machine learning will continue to play a pivotal role, enhancing the accuracy and efficiency of predictive models. These technologies enable more sophisticated data analysis, allowing for real-time predictions and adaptive learning capabilities. Additionally, the integration of blockchain technology promises to improve data security and transparency, providing more reliable datasets for analysis. Innovations such as quantum computing hold the potential to revolutionise predictive analytics by solving complex computations that are currently beyond reach, thereby delivering unprecedented predictive power. Furthermore, the rise of the Internet of Things (IoT) will contribute to the proliferation of data sources, offering richer datasets for regression analysis and more comprehensive insights. As these technologies mature, they will drive the development of even more advanced predictive analytics solutions, enabling businesses to stay ahead in an increasingly data-driven world.
Expanding Applications in Finance
The applications of predictive analytics in finance are poised to expand as technology advances and data availability increases. Beyond traditional areas like risk assessment, sentiment analysis and market forecasting, predictive analytics is set to transform various financial services. For instance, in personal finance, predictive models can offer customers personalised financial advice, helping them manage budgets and investments more effectively. In the realm of credit scoring, advanced analytics can provide a more nuanced assessment of creditworthiness, incorporating alternative data sources for a comprehensive evaluation. Additionally, in fraud detection, predictive analytics can enhance the ability to identify and prevent fraudulent activities by recognising patterns that indicate potential threats. Asset management and portfolio optimisation are also areas where predictive analytics can drive better decision-making and performance. As financial institutions continue to explore these expanding applications, predictive analytics will increasingly become integral to strategic planning and operational efficiency, offering enhanced value to both institutions and their customers.
Challenges and Opportunities Ahead
As predictive analytics becomes more ingrained in financial operations, both challenges and opportunities will emerge. One major challenge is ensuring data privacy and security, especially as data sources multiply and regulations tighten. Institutions must navigate these complexities to maintain trust and compliance. Additionally, the integration of new technologies can be resource-intensive, requiring significant investment in infrastructure and talent. On the flip side, these challenges present opportunities for innovation and differentiation. By adopting cutting-edge technologies and robust security measures, financial institutions can gain a competitive edge. Moreover, the growing emphasis on data-driven decision-making can lead to more efficient and customer-focused services. As predictive analytics capabilities expand, organisations that can effectively harness these tools will be better positioned to anticipate market shifts and optimise their offerings. The ability to balance these challenges with strategic opportunities will be crucial for financial institutions aiming to thrive in the future landscape of predictive analytics.
FAQ
- What is predictive analytics in finance?
Predictive analytics in finance uses historical data and advanced algorithms to forecast future financial trends and outcomes. - How does predictive analytics enhance financial forecasting?
Predictive analytics improves financial forecasting by analyzing patterns, providing accurate predictions, and enabling proactive decision-making. - Why is predictive modeling important in financial forecasting?
Predictive modeling helps in assessing credit risk, forecasting cash flow, and identifying potential investment opportunities with precision. - What are the key benefits of integrating predictive analytics into financial operations?
Key benefits include improved accuracy in forecasting, reduced operational costs, better cash flow management, and enhanced strategic planning. - How does predictive analytics support risk management in finance?
Predictive analytics models identify potential risks and provide actionable insights, helping organizations mitigate financial uncertainties. - Can predictive analytics improve investment strategies?
Yes, predictive analytics analyzes historical data and financial indicators to optimize investment strategies and identify profitable opportunities. - How does predictive analytics software handle fraud detection?
Predictive analytics detects fraud by analyzing patterns and flagging anomalies in transaction data, ensuring secure financial operations. - What role does predictive analytics play in credit risk assessment?
Predictive analytics assesses credit risk by evaluating historical data and predicting the likelihood of loan defaults. - How does predictive analytics enhance cash flow forecasting?
Predictive analytics improves cash flow forecasting by analyzing patterns in revenue and expenses to predict future financial conditions. - Why is real-time data processing critical in predictive analytics?
Real-time data processing ensures timely updates and accurate predictions, enabling organizations to adapt quickly to market changes. - How do predictive analytics models integrate with customer relationship management systems?
Predictive analytics models enhance customer relationship management by analyzing data to forecast customer behaviors and preferences. - What are the ethical considerations in predictive analytics for finance?
Ethical considerations include data privacy, accuracy in predictions, and compliance with data protection regulations. - Can predictive analytics help in strategic decision-making?
Yes, predictive analytics provides data-driven insights that support strategic planning and better financial decision-making. - How does predictive analytics software improve operational efficiency in finance?
By automating data collection and analysis, predictive analytics reduces manual efforts and improves efficiency in financial operations. - What are the challenges of integrating predictive analytics into financial forecasting?
Challenges include ensuring data quality, aligning tools with business needs, and navigating data protection regulations. - What future trends will shape predictive analytics in financial forecasting?
Emerging technologies like AI, machine learning, and IoT will drive innovations in predictive analytics for finance. - How does predictive analytics assist in sentiment analysis for financial markets?
Predictive analytics uses sentiment analysis to assess market trends based on social media and news data, informing investment strategies. - What industries benefit most from predictive analytics software?
Financial services, insurance, and retail are among the industries leveraging predictive analytics for operational and strategic advantages. - How does predictive analytics support regulatory compliance in finance?
Predictive analytics ensures compliance by monitoring data and generating reports that meet regulatory requirements. - Why is predictive analytics considered a game-changer in financial forecasting?
Predictive analytics transforms financial forecasting by providing accurate predictions, improving cash flow management, and optimizing risk strategies.
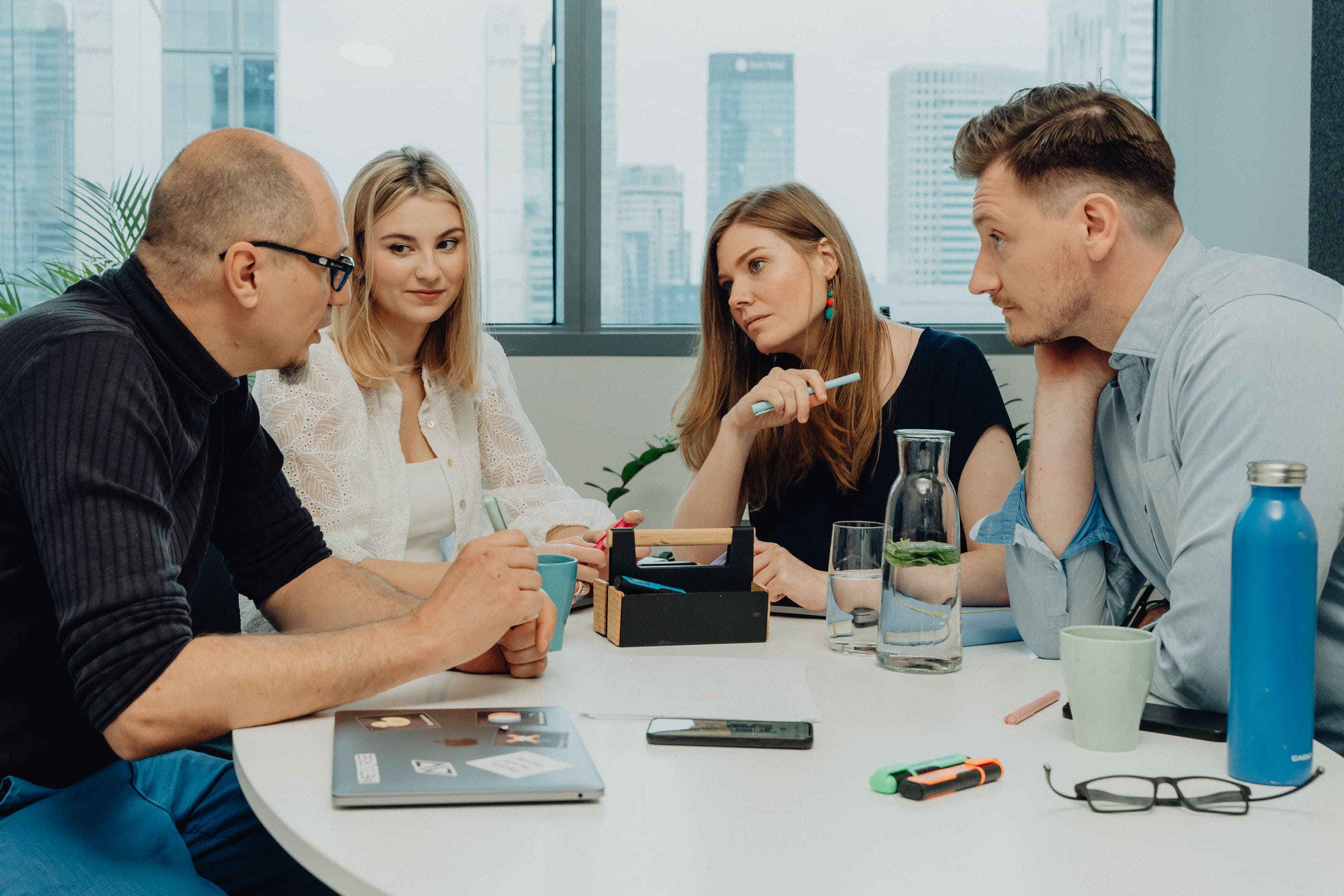
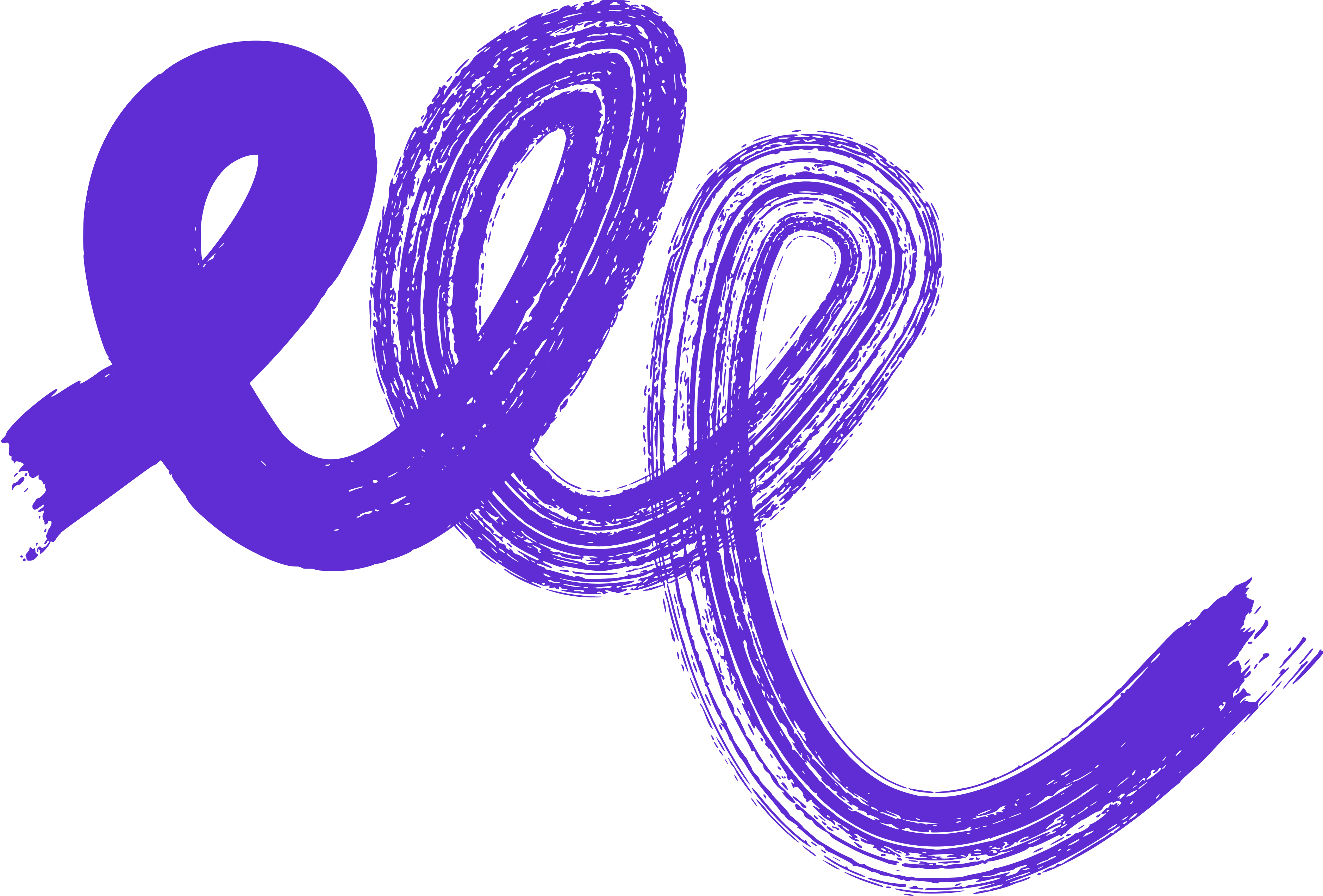
You may also
like...
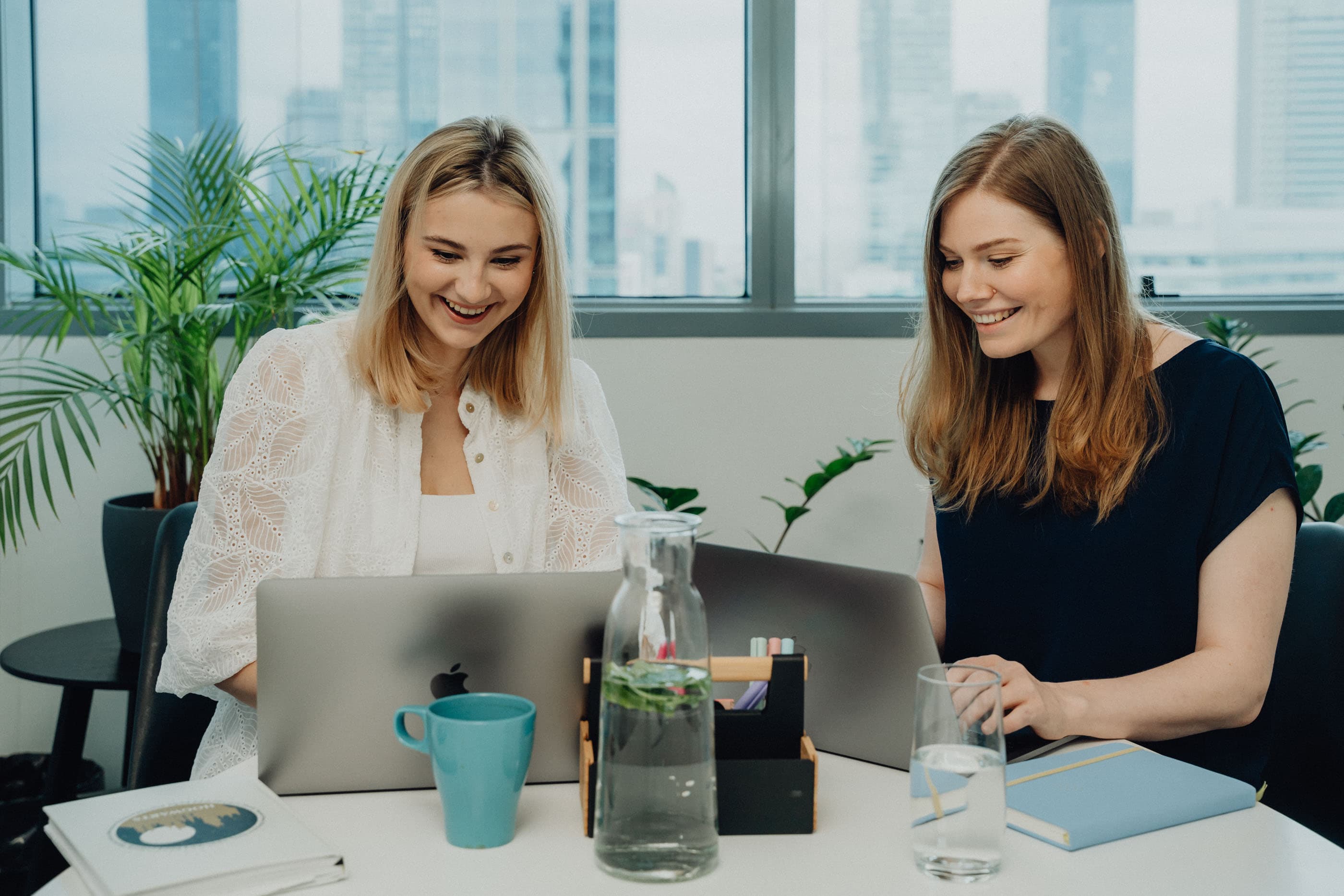
Mastering Declarative Programming: Essential Practices for Every Developer
Discover declarative programming essentials. This guide covers principles, tools, and best practices to simplify coding, enhance readability, and improve scalability.
Marek Pałys
Apr 16, 2024・11 min read
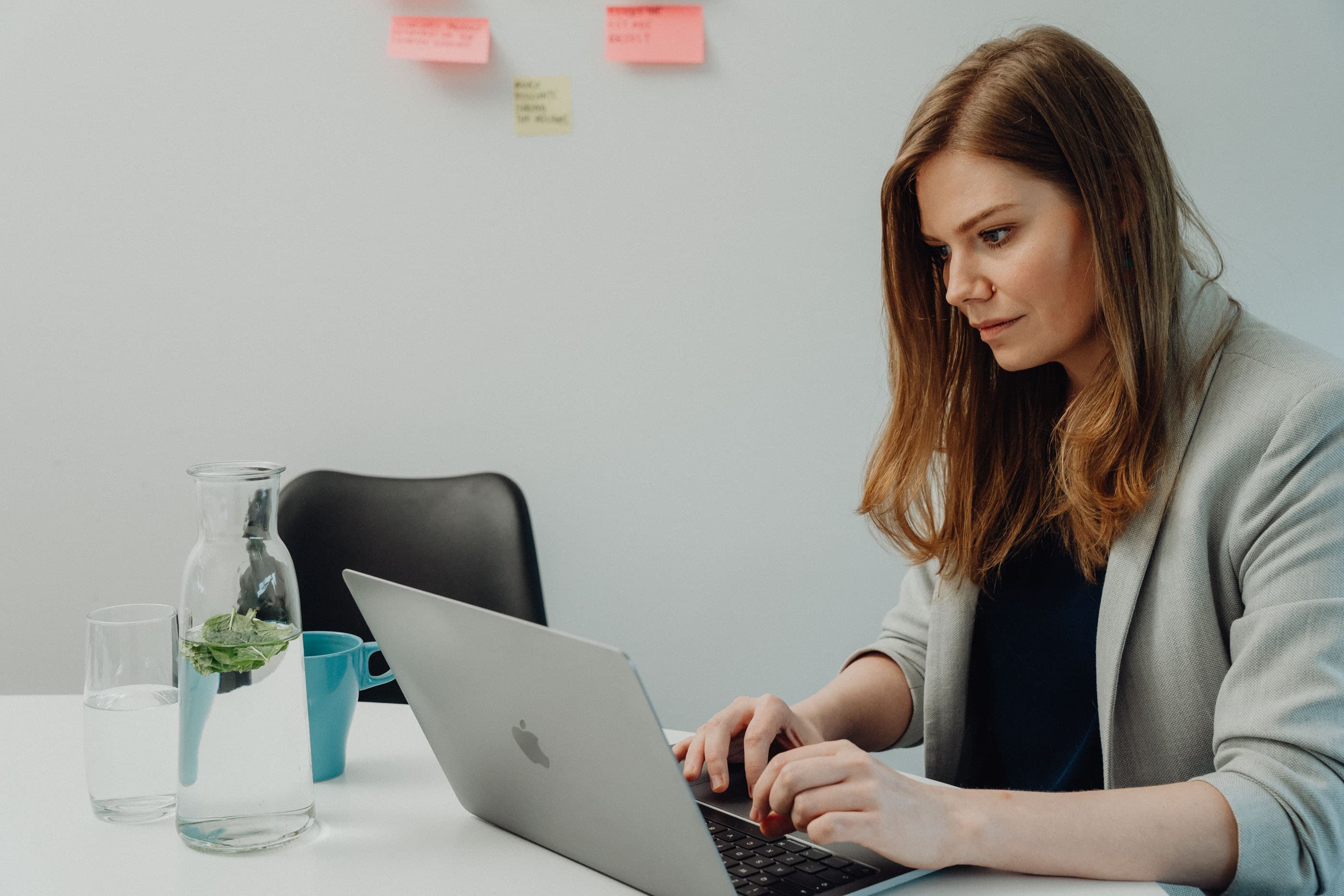
Understanding Event-Driven Programming: A Simple Guide for Everyone
Explore the essentials of event-driven programming. Learn how this responsive paradigm powers interactive applications with real-world examples and key concepts.
Marek Pałys
Apr 30, 2024・9 min read
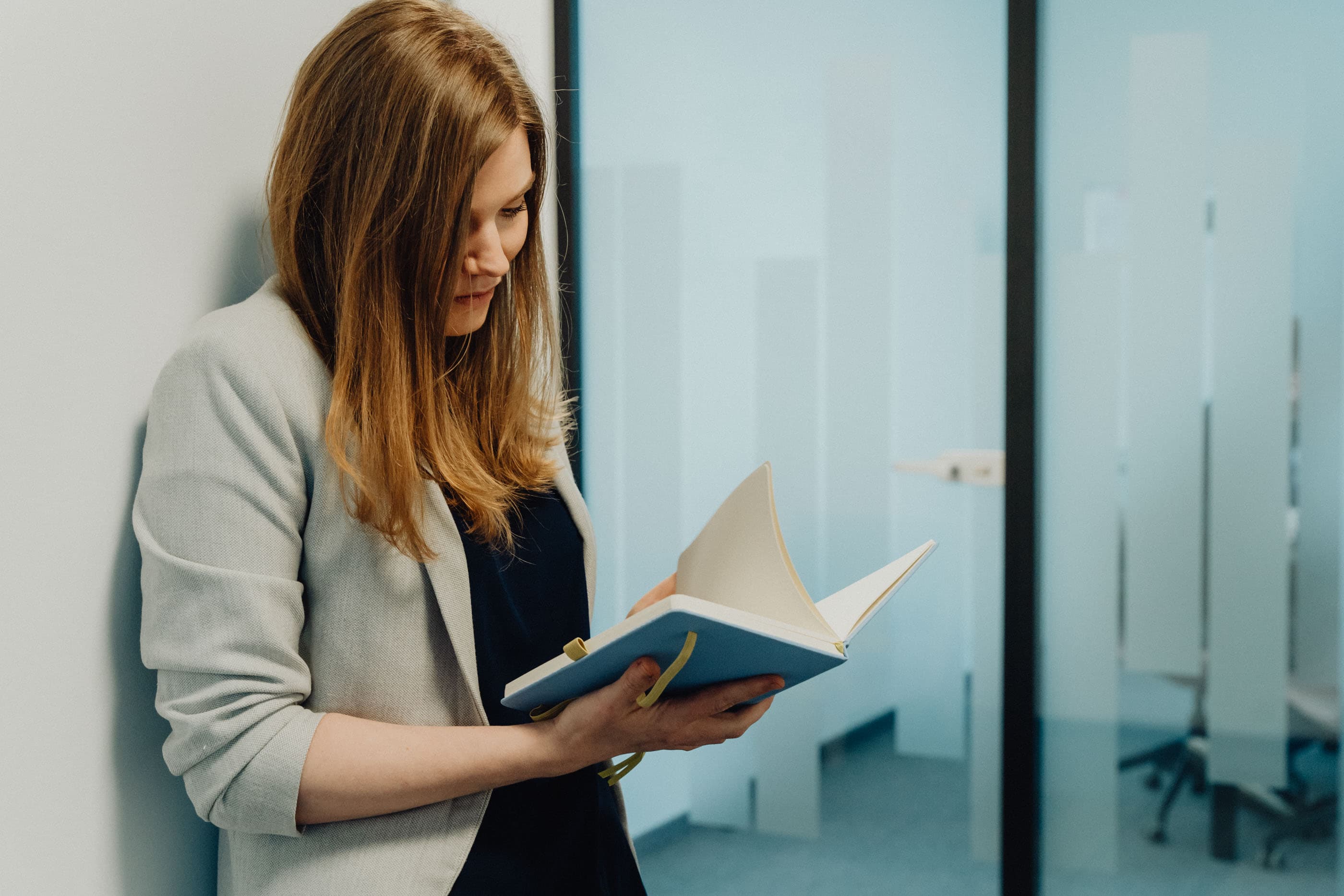
Demystifying Procedural Programming: Simple Examples for All
Explore procedural programming with easy-to-follow examples and insights into its core principles. Learn how this step-by-step approach forms the basis of many programming paradigms.
Marek Pałys
Jul 05, 2024・10 min read