🌍 All
About us
Digitalization
News
Startups
Development
Design
The Ultimate Guide to Prompt Tuning: Boosting Efficiency and Maximising Performance
Marek Majdak
Jan 18, 2024・9 min read
Table of Content
Understanding Prompt Tuning
Boosting Efficiency with Prompt Tuning
Maximising Performance with Prompt Tuning
Advanced Techniques in Prompt Tuning
Measuring Success in Prompt Tuning
Prompt tuning is an essential process that holds the key to boosting efficiency and maximising performance in various applications. From enhancing responsiveness to streamlining operations, the art of fine-tuning prompts plays a crucial role in optimising outcomes. In this comprehensive guide focusing on prompt tuning, we will delve into the intricate details of how this practice can revolutionise efficiency and elevate performance levels across different platforms and systems. Stay tuned to unravel the secrets of prompt tuning and unlock its full potential for your operations.
Understanding Prompt Tuning
What is Prompt Tuning?
Prompt tuning is a technique used to adjust and refine the inputs given to machine learning models, particularly in natural language processing (NLP). It involves crafting precise prompts that guide the model to understand the task at hand more effectively, thereby producing more accurate and relevant outputs. Instead of retraining the entire model, which can be time-consuming and resource-intensive, prompt tuning fine-tunes the model to respond to specific instructions or questions. This method allows for quick adjustments and adaptations to new data or requirements, ensuring that the model remains efficient and effective in its performance.
Significance of Prompt Tuning
The significance of prompt tuning cannot be overstated, as it represents a shift towards more efficient model optimisation. It allows for the customisation of AI behaviour without the need for extensive datasets or exhaustive retraining. This is particularly important in scenarios where quick adaptation is crucial. For instance, when businesses need to deploy AI models to understand and engage with new market trends, prompt tuning offers a swift route to alignment.
Moreover, prompt tuning can lead to cost savings by reducing the computational power required for model adjustments. It also enables a more accessible approach to AI, as non-experts can modify prompts to achieve desired outcomes without deep technical knowledge. Overall, prompt tuning empowers users to harness the capabilities of advanced AI models more effectively and responsively.
Boosting Efficiency with Prompt Tuning
Role of Prompt Tuning in Efficiency
Prompt tuning serves as a pivotal tool in enhancing the efficiency of machine learning systems. By fine-tuning the prompts given to AI models, users can extract high-quality results without the need for large-scale retraining or data collection. This aspect of prompt tuning is fundamental in scenarios where rapid deployment and agile responses are valuable.
In environments where data is dynamic and constantly evolving, like social media analysis or customer service interactions, the ability to quickly adjust prompts means that AI systems can stay up-to-date with minimal downtime. This efficiency is not only beneficial in terms of speed but also in reducing the resources required for maintaining and updating AI models. Consequently, prompt tuning is a critical component in ensuring that AI systems are not just powerful, but also nimble and cost-effective.
Case Studies: Efficiency through Prompt Tuning
Several case studies highlight the efficiency gains achieved through prompt tuning. For instance, a tech company used prompt tuning to refine their customer service chatbot. By adjusting the prompts to align with the evolving language and queries of their customers, the company significantly reduced the response time and improved the relevancy of the chatbot's answers, enhancing customer satisfaction.
Another example is a content recommendation platform that implemented prompt tuning to fine-tune its algorithm. This approach allowed the platform to quickly adapt to users' changing preferences, resulting in a more personalised user experience and increased engagement. These case studies demonstrate that prompt tuning is not just theoretical but a practical tool that can lead to measurable improvements in efficiency and user satisfaction.
Maximising Performance with Prompt Tuning
How Prompt Tuning Enhances Performance
Prompt tuning enhances performance by significantly refining the output quality of AI models. It achieves this by directing the model's attention to relevant aspects of a task, which in turn increases the accuracy and applicability of its responses. This fine-tuning process allows models to quickly adapt to specific domains or specialised knowledge without the need for extensive retraining on new datasets.
For example, in language translation services, prompt tuning can be used to adapt a general model to understand and translate industry-specific jargon more effectively. Similarly, in voice recognition software, prompt tuning can improve the model's ability to understand different accents or dialects. By improving the quality of interactions and results, prompt tuning directly contributes to elevated performance levels in AI-driven applications.
Real-world examples: Performance Boost from Prompt Tuning
In the real world, prompt tuning has proven its value by boosting the performance of AI systems in various industries. A notable example is in the field of healthcare, where an AI model was prompt-tuned to understand and process medical terminology more effectively. This resulted in improved diagnostic recommendations and a better understanding of patient records, ultimately enhancing the quality of patient care.
Another case is in the finance sector, where prompt tuning helped a trading algorithm better interpret market sentiment by understanding nuanced financial language. This led to more accurate market predictions and improved trading strategies. These examples underscore the practical benefits of prompt tuning, showing that it is a powerful method for enhancing the performance of AI models in complex, real-world situations.
Advanced Techniques in Prompt Tuning
Next-Level Tuning Strategies
Taking prompt tuning to the next level involves more sophisticated strategies that can further enhance the model's performance. One such technique is the use of chained prompts, where a series of interconnected prompts guide the model through complex tasks in a step-by-step manner, improving the accuracy of the final output.
Another advanced strategy is the iterative refinement of prompts, where initial outputs are used to adjust and optimise the prompts in a feedback loop, honing the model's focus and response quality. Additionally, employing conditional prompts that adapt based on contextual cues can lead to more dynamic and situationally aware AI responses. These advanced strategies require a deeper understanding of the model's workings and the specific task requirements but can lead to significant gains in the model's performance and versatility.
Avoiding Common Tuning Pitfalls
To fully leverage the benefits of prompt tuning, it's crucial to be aware of common pitfalls and how to avoid them. One frequent mistake is overfitting the prompts to specific examples, which can reduce the model's ability to generalise to new data. It's important to use a diverse set of examples when crafting prompts to ensure broad applicability.
Another pitfall is under-tuning, where the prompts are not specific enough to guide the model effectively. This can lead to vague or off-target responses. Balancing specificity and flexibility is key.
Finally, neglecting to test prompts in different contexts can lead to unexpected results when the model encounters unfamiliar scenarios. Continuous testing and iteration of prompts can help to build robustness into the model's performance, ensuring it can handle a wide range of inputs effectively.
Measuring Success in Prompt Tuning
Key Metrics for Tuning Success
When measuring the success of prompt tuning efforts, several key metrics should be considered. Accuracy is often the primary metric, comprising the proportion of correct responses generated by the AI model. A high accuracy rate indicates that the prompts are effectively guiding the model to the desired outcomes.
Another important metric is response time, especially in applications where speed is critical. A successful prompt tuning should lead to faster response times without sacrificing quality. Additionally, the rate of model adaptation, which measures how quickly the AI model can adjust to new prompts, is a critical metric indicating the flexibility and efficiency of the tuning process.
Engagement metrics, such as user interaction rates or satisfaction scores, can also provide insights into the effectiveness of prompt tuning from an end-user perspective. Collectively, these metrics paint a comprehensive picture of the tuning success and areas for further improvement.
Continuous Improvement in Prompt Tuning
Achieving continuous improvement in prompt tuning requires a commitment to ongoing evaluation and adaptation. This involves regularly assessing how the prompts are performing against the key metrics and making iterative refinements based on the insights gathered. It's important to collect feedback, not just from the model's outputs, but also directly from the end-users who interact with the AI system.
Incorporating new data and examples into the prompt tuning process can help the model stay relevant and accurate over time. Additionally, staying informed about the latest advancements in AI and machine learning can provide new techniques and approaches for enhancing prompt tuning strategies.
By embracing a culture of continuous learning and development, organisations can ensure that their AI systems remain at the forefront of efficiency and performance, effectively utilising prompt tuning as a tool for sustainable advancement.
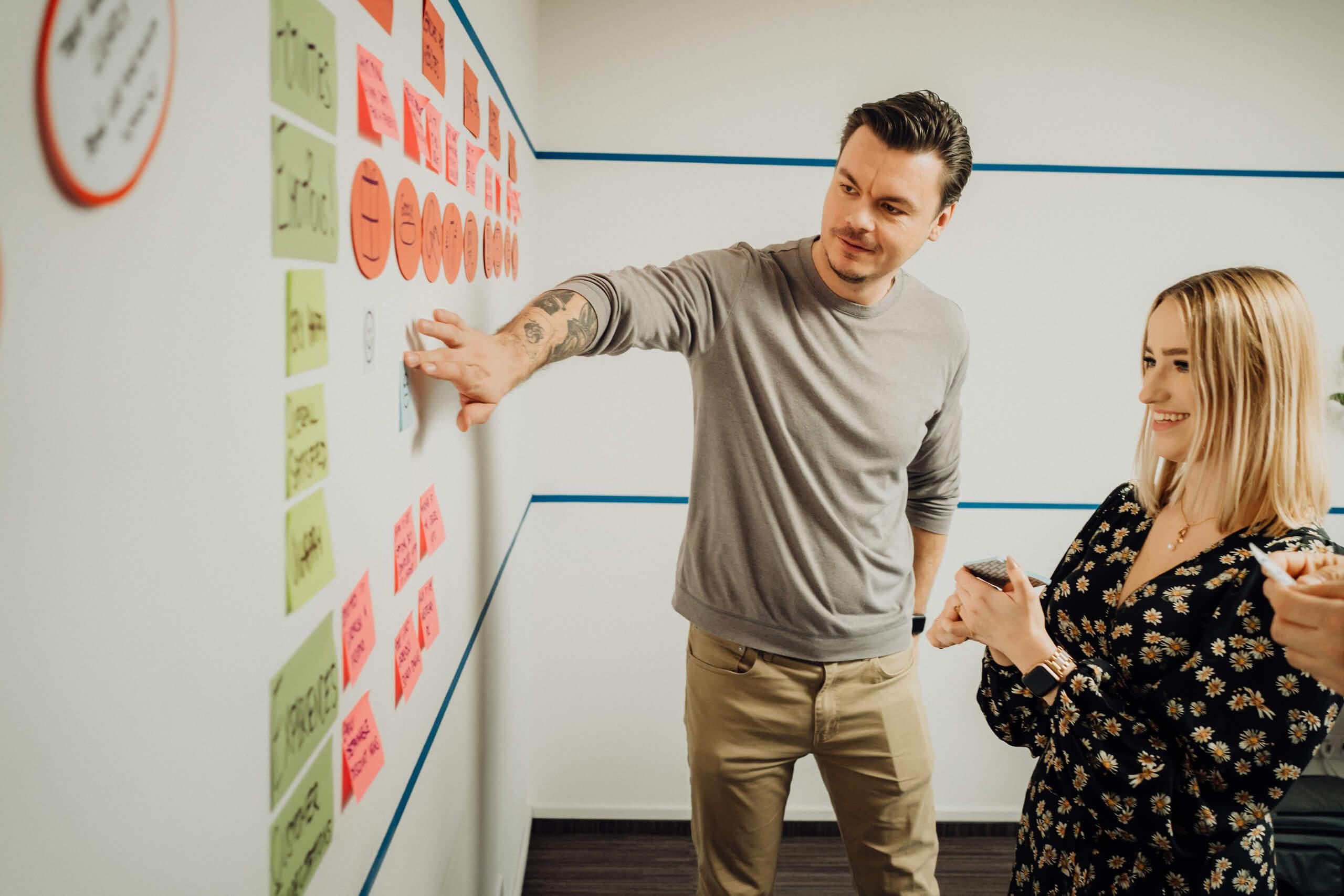
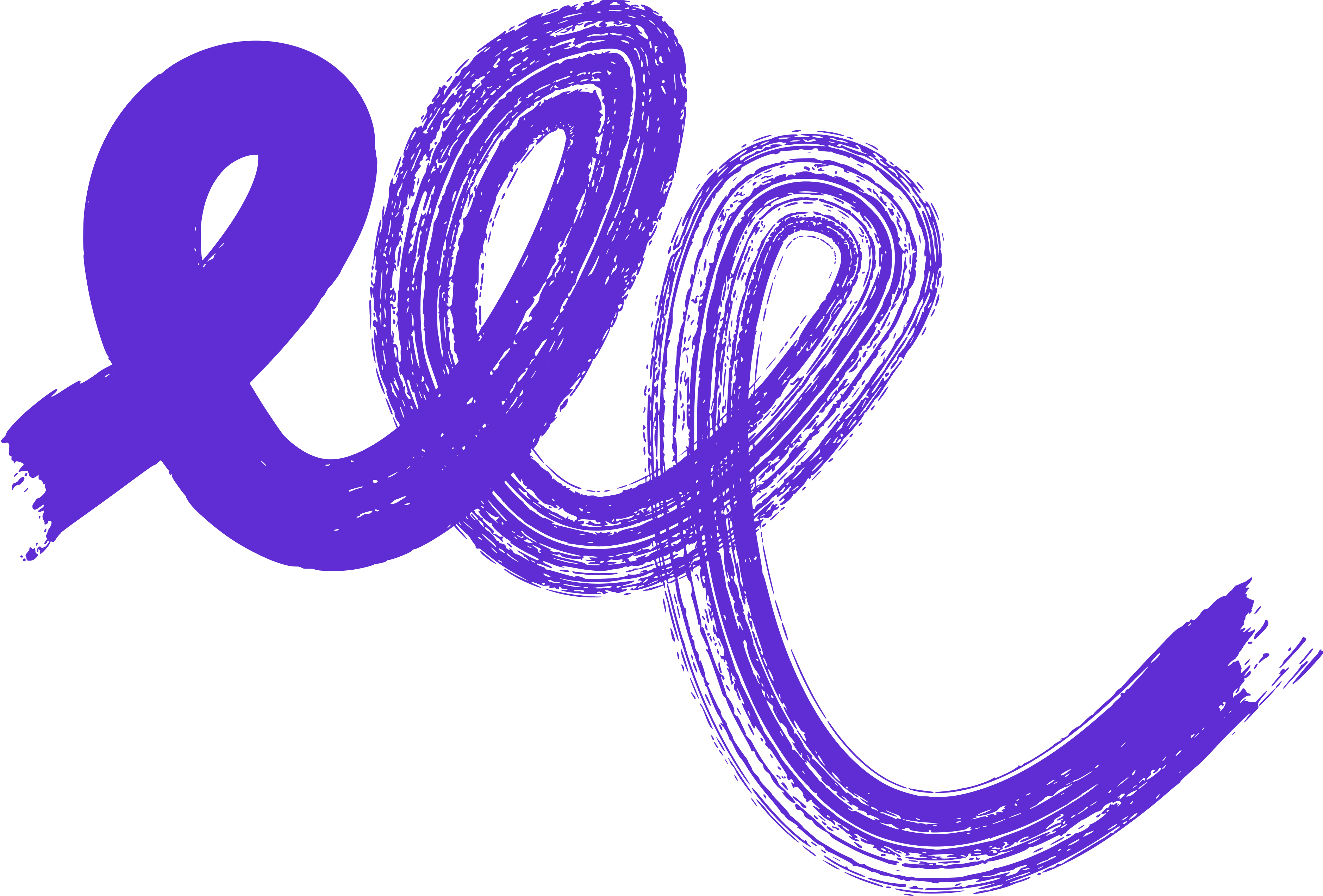
You may also
like...
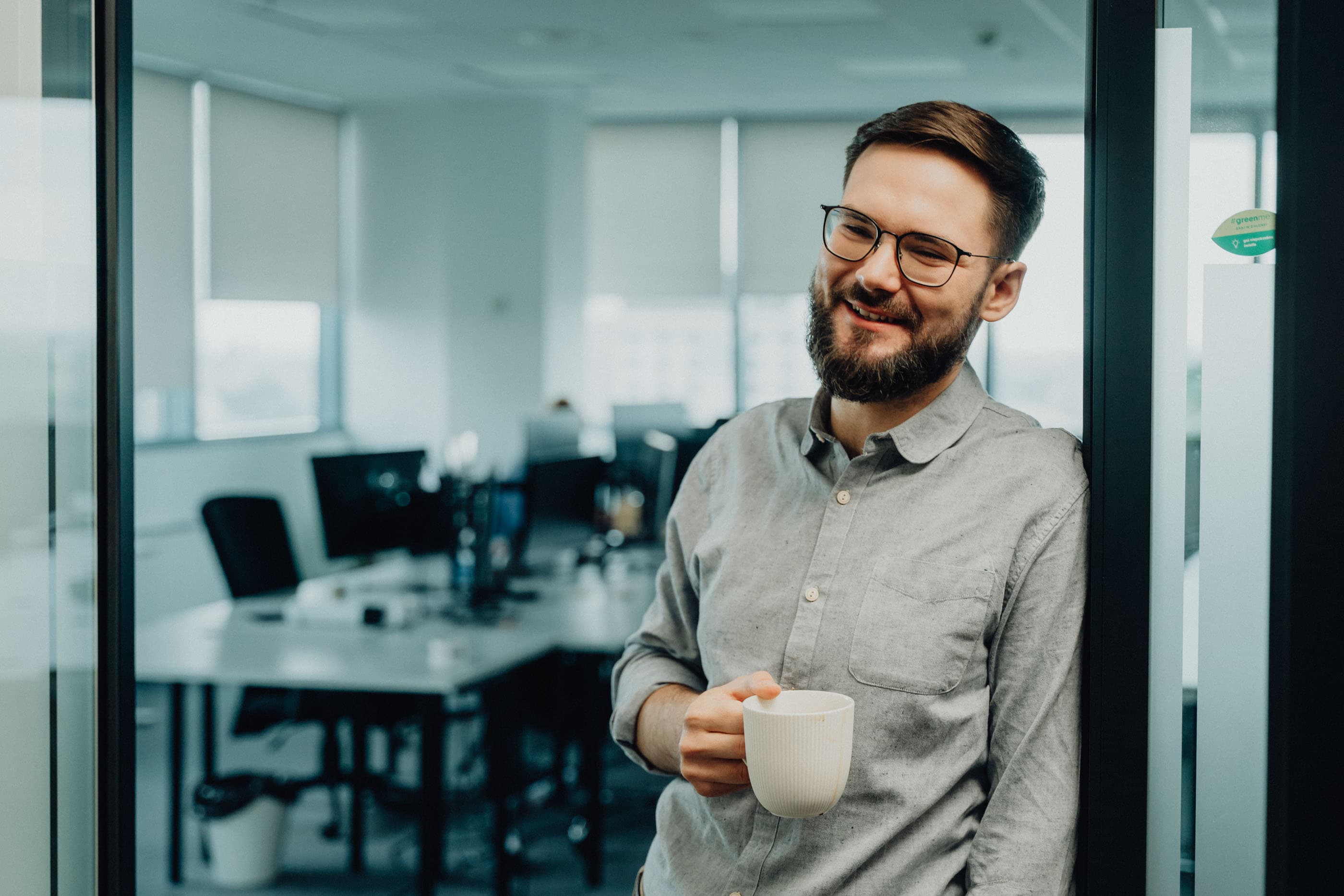
Mastering the Art of Hiring Remote Developers: A Comprehensive Guide
As the demand for skilled remote developers continues to grow, mastering the art of hiring remote teams is crucial for any business looking to stay competitive. This guide provides a step-by-step approach to hiring remote developers, from understanding the importance of remote work and its challenges to crafting the perfect job description and sourcing talent through the right platforms. Learn how to conduct effective remote interviews, assess technical skills, and evaluate cultural fit to build a strong and cohesive remote team. We also cover essential onboarding and integration practices to ensure your new hires are set up for success and fully integrated into your company culture. Whether you're a seasoned recruiter or new to remote hiring, this guide offers practical tips and insights to help you navigate the complexities of hiring remote developers efficiently and successfully.
Marek Pałys
Jun 11, 2024・5 min read
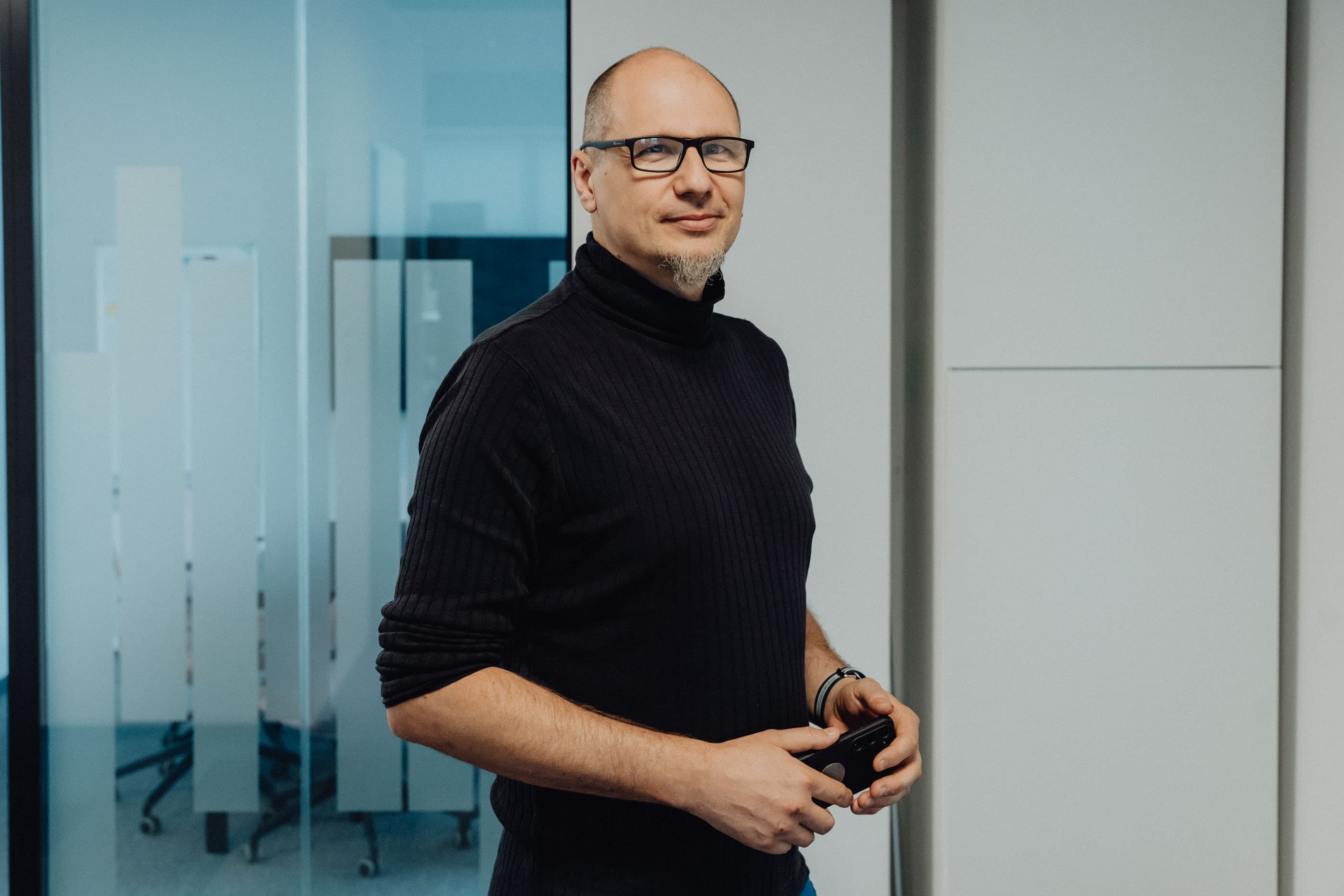
How to Hire Mobile App Developers: A Practical Guide for Businesses
Hiring the right mobile app developers is crucial for building a strong mobile presence. This guide provides a comprehensive overview of the steps involved in hiring skilled developers who can deliver on your business goals. From defining your project scope and identifying key skills to finding developers and evaluating their portfolios, we cover it all. Learn how to conduct effective interviews, assess technical skills, and evaluate cultural fit to ensure you make the right hiring decisions. Finally, discover tips on negotiating terms and successfully onboarding your new developers to set the stage for productive collaboration.
Marek Pałys
Mar 25, 2024・7 min read
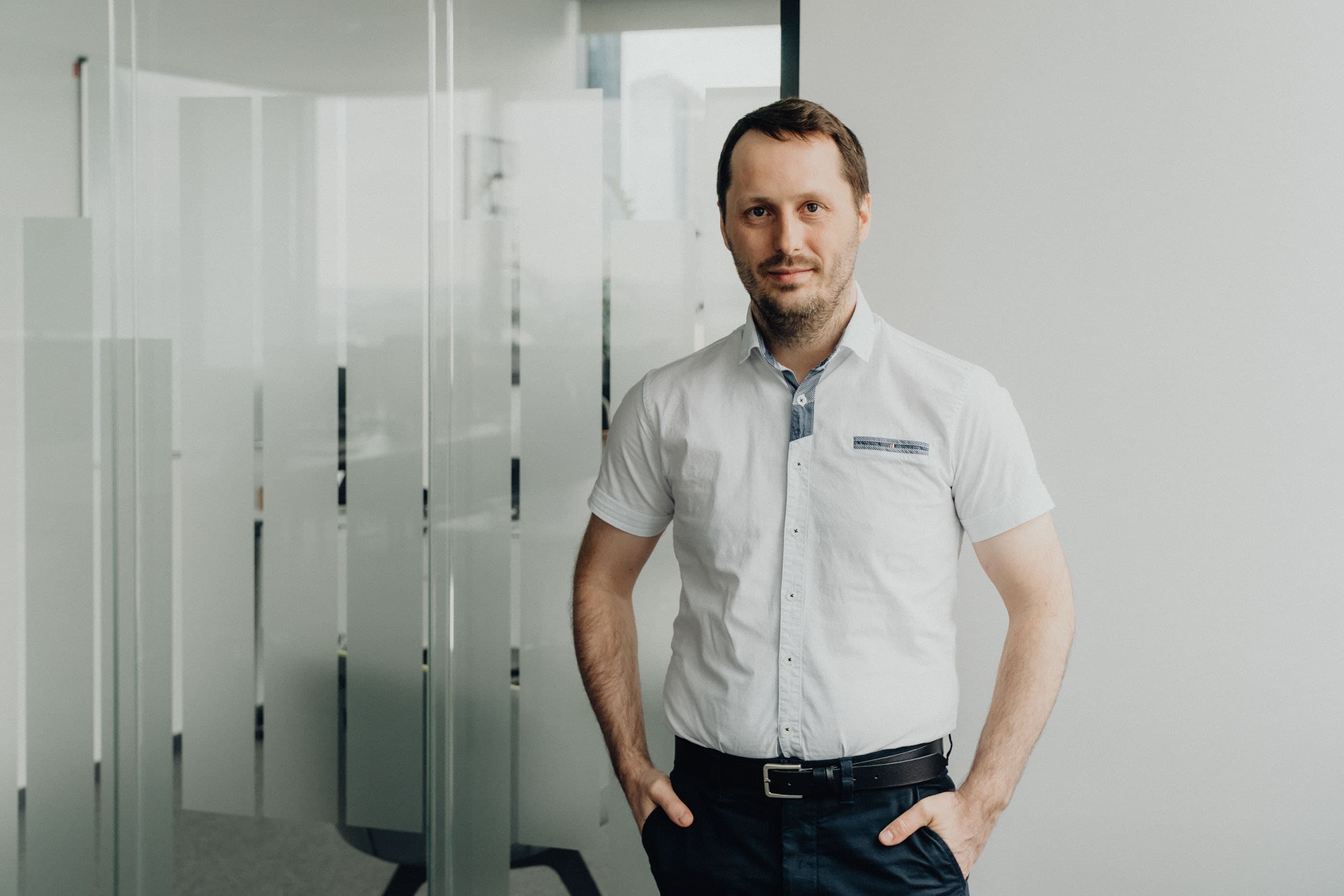
How to Hire Developers for a Startup: A Step-by-Step Guide
Hiring developers for a startup can be challenging but crucial for success. This guide offers practical steps to help you find and recruit the right talent. Whether you're defining project requirements, identifying the necessary skills, or assessing cultural fit, these strategies will ensure you build a technical team that aligns with your startup's vision. Learn how to leverage online platforms, conduct effective interviews, and craft competitive offers that attract top developers. Plus, discover tips on onboarding and fostering a collaborative environment to drive your startup's growth and innovation.
Marek Pałys
Apr 24, 2024・5 min read