🌍 All
About us
Digitalization
News
Startups
Development
Design
Harnessing Machine Learning for Robust Fraud Detection: A 2023 Perspective
Marek Majdak
Aug 24, 2023・5 min read
Table of Content
What is Fraud Detection?
The Benefits of Machine Learning for Fraud Management
Machine Learning vs. Artificial Intelligence in Fraud Detection
ML vs. Traditional Fraud Detection Systems
Fraud Detection Using Machine Learning Algorithms
Disadvantages of Machine Learning for Fraud Detection
Application: How SEON Helps Combine Machine Learning with Manual Reviews
Conclusion
FAQs
As digital transactions continue to surge, so does the challenge of detecting fraudulent activities. One key tool that has empowered businesses, particularly in the banking sector, is Machine Learning (ML). It provides a robust mechanism to identify and prevent potential frauds. In this article, we delve into how ML techniques revolutionize fraud detection.
What is
Fraud Detection?
Fraud detection involves identifying unusual activity that could be fraudulent. It's of paramount importance in the banking sector, where fraud can include identity theft, credit card fraud, or account takeover (ATO attacks).
The Benefits of Machine Learning for Fraud Management
Machine learning offers several key benefits over traditional fraud detection methods:
- Improved Accuracy: ML models are typically more effective than humans at identifying subtle patterns indicative of fraudulent transactions.
- Scalability: ML algorithms can process vast amounts of data quickly, making them scalable for large financial institutions.
- Real-time Detection: ML allows for real-time fraud detection, thus preventing potential fraud before it impacts the customer or the bank.
Machine Learning vs. Artificial Intelligence in Fraud Detection
While both ML and AI enhance fraud detection, they do so differently. Machine learning, a subset of AI, focuses on algorithms that improve through experience. It's particularly effective in fraud detection, where patterns evolve rapidly.
ML vs. Traditional Fraud Detection Systems
Traditional rule-based systems require manual review and transaction rules. They often struggle to keep up with the volume and sophistication of today's fraudulent transactions. On the other hand, ML systems leverage historical and real-time transaction data to detect anomalous behavior, making them far more effective and efficient.
Fraud Detection Using
Machine Learning Algorithms
Various ML-based fraud detection algorithms are used today, broadly categorized into supervised, unsupervised, semi-supervised, and reinforcement learning:
- Supervised Learning: Here, the ML model is trained on historical data where fraudulent and legitimate transactions are labeled. The model learns to classify new transactions based on this training. This approach is often used for credit card fraud detection.
- Unsupervised Learning: Unlike supervised learning, unsupervised learning doesn't rely on labeled data. Instead, it identifies patterns and anomalies in the data, enabling it to spot potential fraud that might not match known patterns.
- Semi-supervised Learning: It combines labeled and unlabeled data for training, making it effective when only a small amount of labeled data is available.
- Reinforcement Learning: This is a type of machine learning where an agent learns to make decisions by taking actions that maximize a reward. In fraud detection, reinforcement learning can be used to optimize the detection strategy.
Disadvantages of Machine Learning for Fraud Detection
While powerful, ML also has limitations in fraud detection:
- False Positives: An ML model might falsely identify a legitimate transaction as fraudulent, leading to customer dissatisfaction.
- Dependence on Quality of Data: The performance of ML models depends heavily on the quality and relevance of the training data.
- Complexity: Developing, testing, and implementing ML models requires a high level of expertise.
Application: How
SEON Helps Combine Machine Learning with Manual Reviews
SEON is a fraud detection solution that combines ML with manual reviews. It empowers data scientists with tools to train the ML algorithm, test rules on historical data, review, and activate the rule. SEON effectively handles various fraud scenarios like payment gateways and chargeback fraud, BNPL, account takeover attacks, and online stores transaction fraud.
Conclusion
Machine Learning has undeniably transformed the landscape of fraud detection, making it possible to predict and prevent financial fraud more accurately and efficiently than ever before. As ML technology continues to evolve, the potential to enhance security across the banking industry will only grow.
Remember, while ML offers an advanced line of defense against fraud, the blend of human intelligence with automated systems like that offered by SEON ensures optimal fraud management. A parting thought for AI enthusiasts - Why did the ML model get thrown out of school? It had too many "bias"! Onward and upward to a safer digital future!
FAQs
- What is Fraud Detection? Fraud detection is a set of activities undertaken to identify unusual activity that could be indicative of fraudulent transactions, especially in the banking sector.
- How is Machine Learning used in Fraud Detection? Machine Learning, through its ability to process and learn from large volumes of data, is used to identify patterns and anomalies that may indicate fraudulent activities. It can help in real-time detection of fraudulent transactions, making it a powerful tool in the banking industry.
- What are the benefits of using Machine Learning in Fraud Management? Machine Learning offers several benefits in fraud management. It can significantly improve the accuracy of detecting fraudulent transactions, scale effectively as transaction volume increases, and provide real-time detection to prevent potential fraud from causing harm.
- What is the difference between Machine Learning and Artificial Intelligence in Fraud Detection? Artificial Intelligence is the broad discipline of making machines smart, while Machine Learning is a subset of AI that involves training machines to learn from data and improve their performance. In the context of fraud detection, Machine Learning is particularly effective due to its ability to identify and learn from patterns in transaction data.
- How does a Machine Learning system work for Fraud Detection? A Machine Learning system for fraud detection works by training on historical transaction data, learning to identify patterns that indicate fraudulent activity. It then applies this knowledge to new, unseen transactions to detect potential fraud in real-time.
- How do traditional Fraud Detection Systems compare to those based on Machine Learning? Traditional fraud detection systems often rely on predefined rules and manual review, making them less scalable and slower to respond to evolving fraud patterns. In contrast, Machine Learning-based systems can learn and adapt to new patterns, making them more effective in detecting and preventing fraud.
- What are the disadvantages of using Machine Learning for Fraud Detection? Some disadvantages include the risk of false positives, dependency on the quality of training data, and the high level of expertise required to develop, test, and implement ML models.
- What is Supervised Learning in the context of Fraud Detection? Supervised Learning involves training an ML model on labeled data where fraudulent and legitimate transactions are clearly identified. This trained model can then classify new transactions as either fraudulent or legitimate based on its learned patterns.
- How does Unsupervised Learning aid in Fraud Detection? Unsupervised Learning doesn't rely on labeled data. Instead, it identifies patterns and anomalies in the data, enabling it to spot potential fraudulent transactions that might not match known patterns.
- What is the role of Semi-supervised Learning in Fraud Detection? Semi-supervised Learning combines labeled and unlabeled data for training, making it effective when only a small amount of labeled data is available.
- How is Reinforcement Learning utilized in Fraud Detection? In reinforcement learning, an agent learns to make decisions that maximize a reward. In the context of fraud detection, this can be used to optimize the detection strategy.
- What are some practical examples of Machine Learning application in Fraud Detection? Practical examples include credit card fraud detection, detecting account takeover attacks, and identifying transaction fraud in online stores. Machine Learning can also be used in insurance claims fraud detection and in detecting fraudulent behavior in payment gateways.
- How does the SEON platform combine Machine Learning with Manual Reviews? SEON is a fraud detection solution that combines Machine Learning with manual reviews, allowing data scientists to train the ML algorithm, test rules on historical data, and review and activate the rule.
- Why is Machine Learning important in Fraud Detection? Machine Learning is important in fraud detection because of its ability to quickly and accurately identify patterns that might indicate fraud. As fraud attempts become more sophisticated, the use of Machine Learning becomes even more critical.
- What is the role of Deep Learning in Fraud Detection? Deep Learning, a subset of Machine Learning, uses neural networks to process complex patterns and relationships in data. This can be particularly useful in fraud detection where fraudulent patterns might be deeply hidden in the data.
- How does the use of Machine Learning impact Payment Gateways and Chargeback Fraud? Machine Learning can help payment gateways detect fraudulent patterns and minimize the instances of chargeback fraud by identifying suspicious transactions in real-time.
- How can Machine Learning help in detecting Account Takeover (ATO) attacks? Machine Learning algorithms can analyze behavior patterns and detect unusual activities or anomalies that might indicate an account takeover attempt, thus preventing potential fraud.
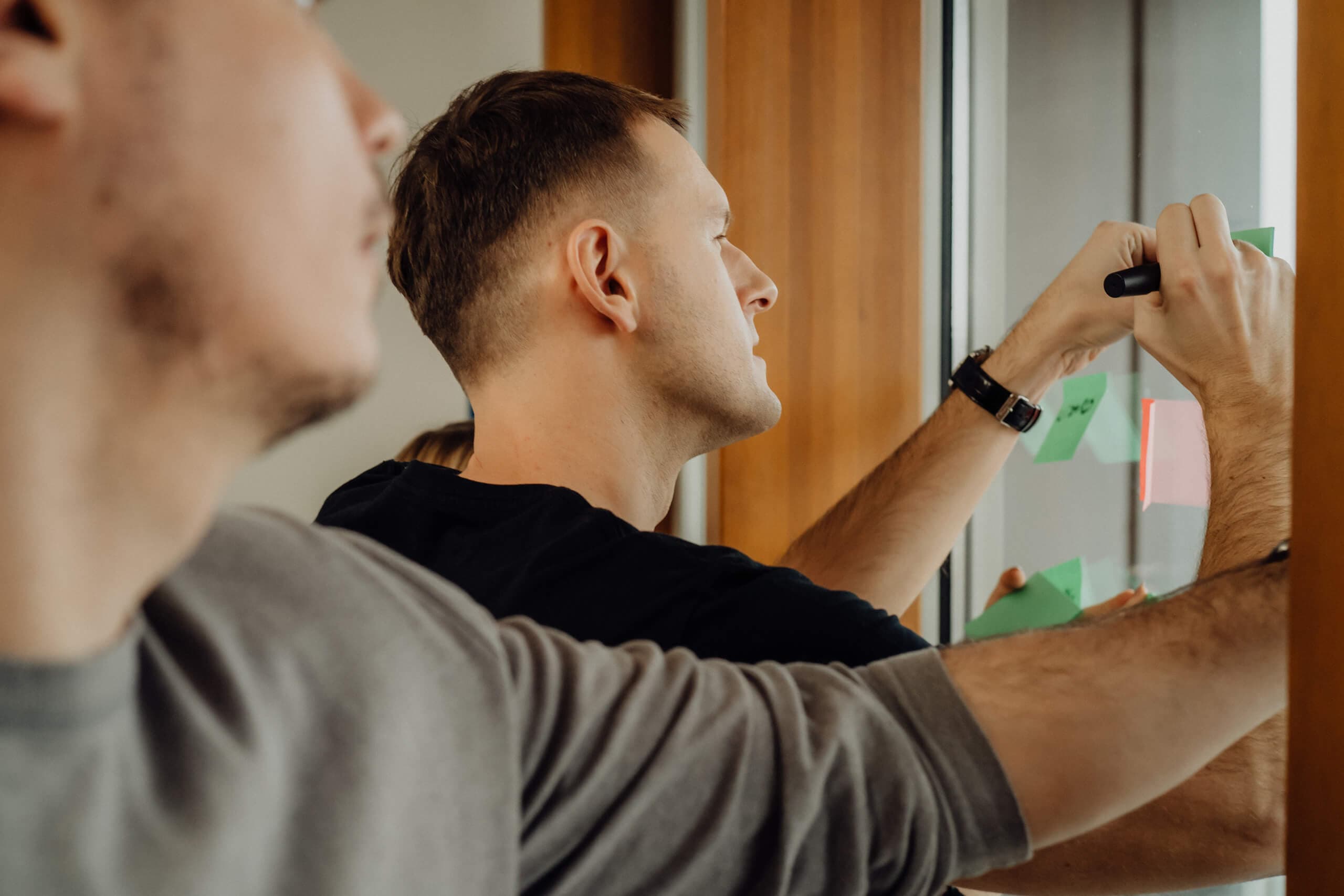
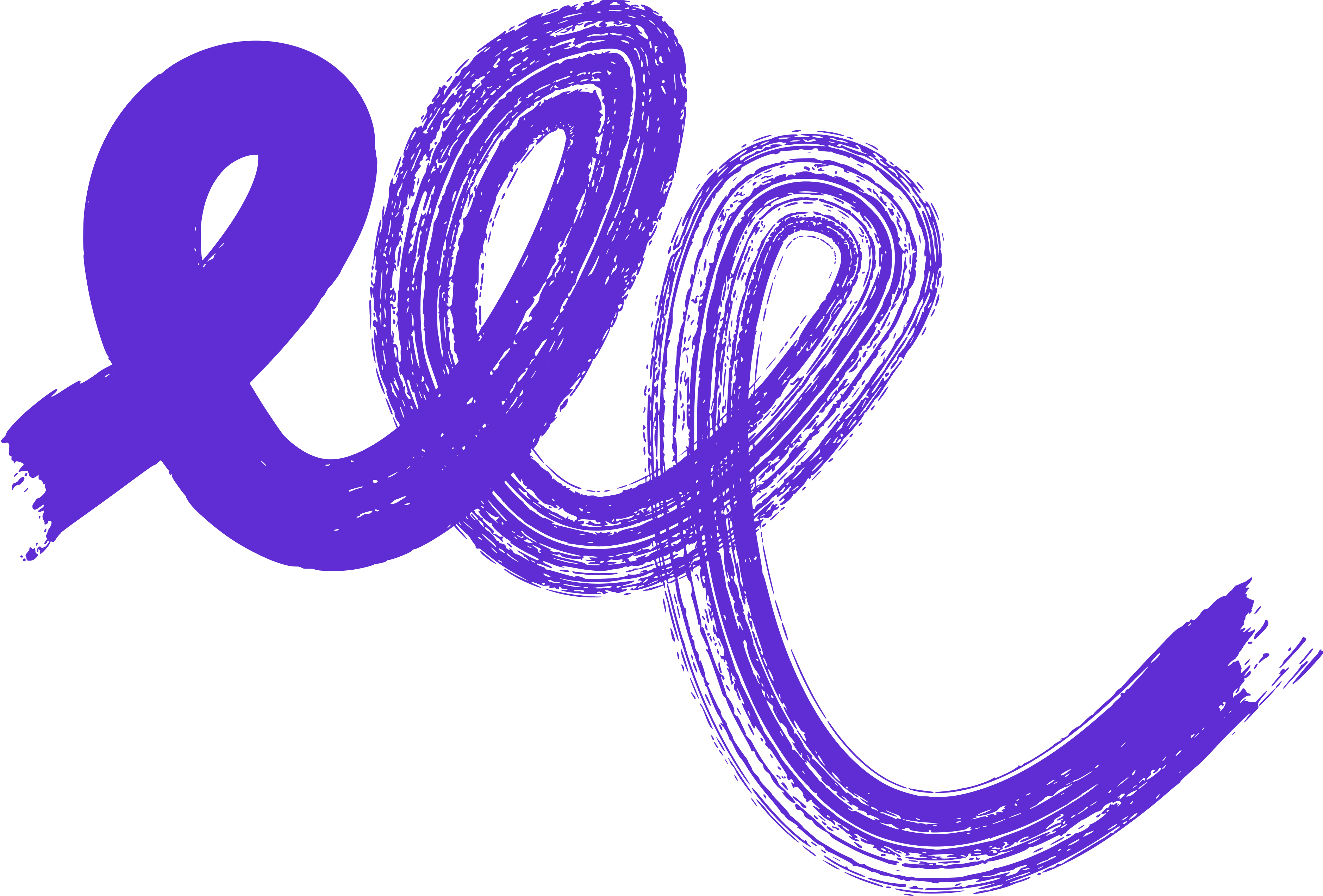
You may also
like...

Understanding Why Your App Rendered More Hooks Than During the Previous Render
This article explores the reasons why your application might render more hooks than during the previous render, a common issue faced by developers. By understanding the mechanics of React hooks and their rendering process, you'll gain practical insights to diagnose and resolve this problem. Enhancing your app's performance and stability is crucial for delivering a seamless user experience.
Marek Majdak
Apr 29, 2024・12 min read
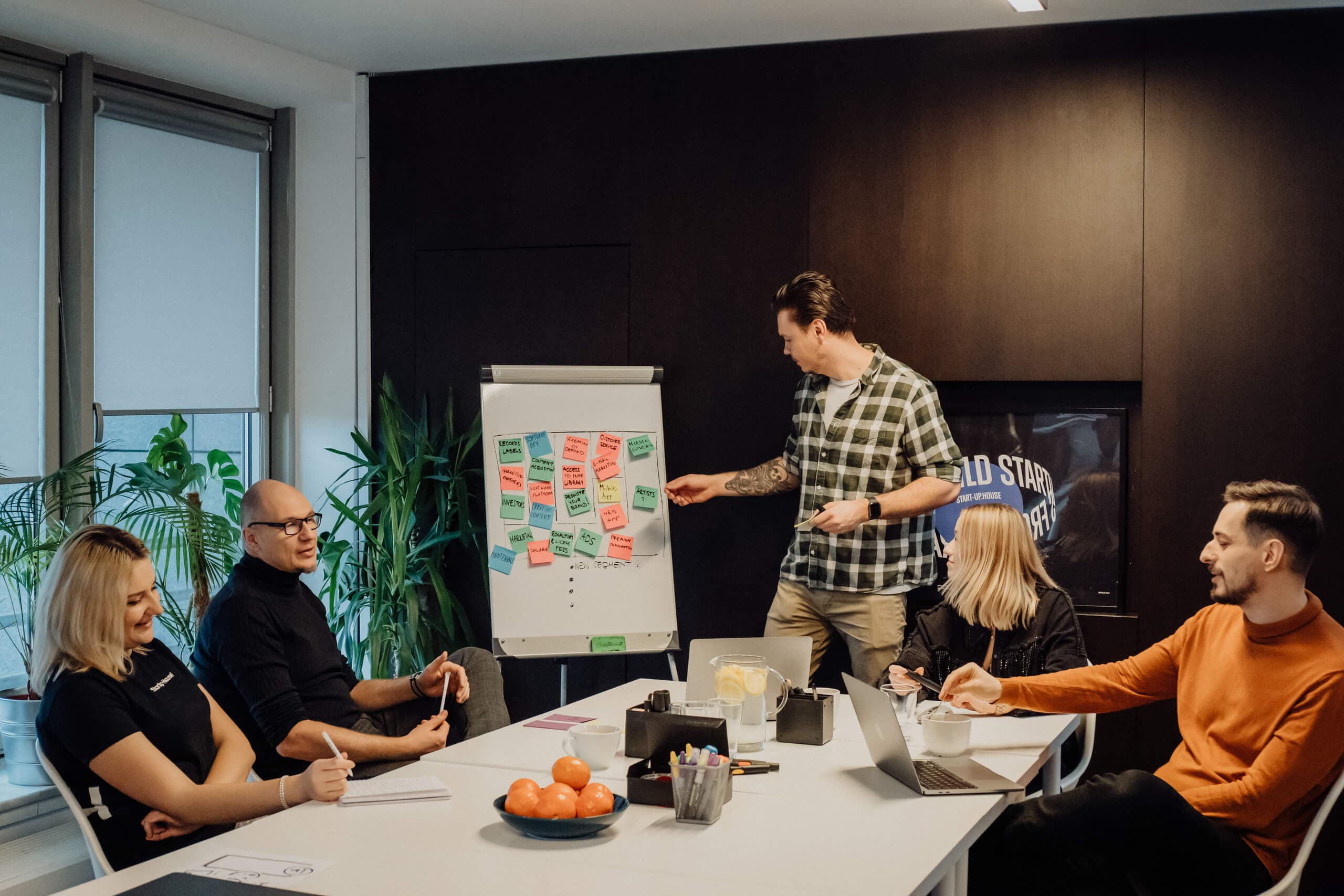
In-house vs Outsourcing Software Development: Making the Right Choice for Your Business
Choosing between in-house and outsourcing software development is a crucial decision that can significantly influence your business’s success. This article explores the distinct advantages and challenges of each approach, helping you assess your specific needs and make an informed choice. Whether you prioritize control and team cohesion or seek specialized skills and cost efficiency, understanding these factors is essential for strategic planning.
Alexander Stasiak
Aug 20, 2024・11 min read
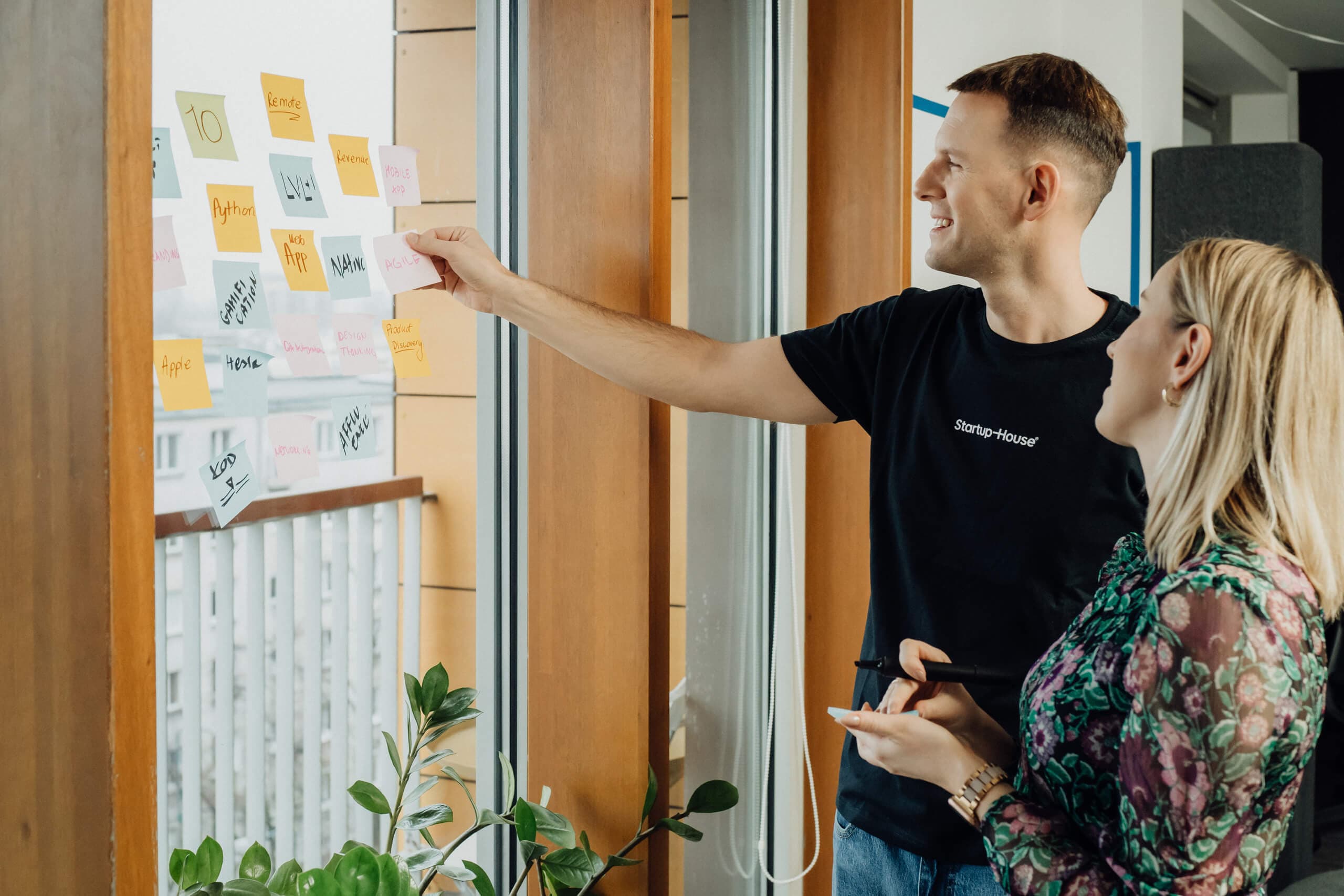
Understanding Software Engineering and Architecture: A Guide for Everyone
This guide provides an insightful overview of software engineering and architecture, essential for anyone interested in technology and development. Covering fundamental concepts, methodologies, and best practices, it equips readers with practical knowledge to navigate the complexities of software systems. Whether you're a beginner or a seasoned professional, understanding these disciplines is vital for building reliable and scalable software solutions.
Marek Pałys
Jan 16, 2024・11 min read